|
16:30
|
0247.
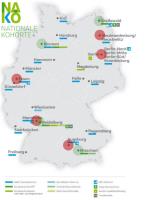 |
Fully Automated Data Management and Quality Assurance in Very
Large Prospective Cohort MR Imaging Studies – the MR Imaging
Study within the German National Cohort 
Jochen G. Hirsch1, Alexander Köhn1,
Daniel C. Hoinkiss1, Jonas Peter1,
Andreas Thomsen1, Matthias Günther1,2,
and the German National Cohort MRI Study Investigators3
1Fraunhofer MEVIS, Bremen, Germany, 2University
Bremen, Bremen, Germany, 3NAKO
MR Imaging Core, Munich, Germany
We present a fully automated data management workflow and
quality assurance, which is set up for large, multicentric
cohort studies including whole-body MR imaging. The workflow
includes a modality worklist, exam-synchronous DICOM
transfer to centralized storage, quality control of MR
acquisition, various image-based quality measures, web-based
radiological image review for incidental findings, visual
quality scores, as well as long-term archiving. This
workflow, implemented in the MRI Study of the German
National Cohort, enables to acquire and process more than 30
whole-body MRI scans per day, available for IF reading
within 4 hours. Deviations, outliers, technical failures are
pointed out on-the-fly.
|
|
16:42
|
0248.
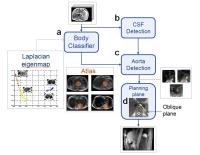 |
Automated slice positioning for 2D MRA in bolus tracking of
DCE-MRI 
Takao Goto1 and
Mirai Araki1
1MR Engineering, GE Healthcare, Hino-shi, Japan
Accurate placement of a 2D plane across the aorta while
examining scout images is a complex task and makes the
operator's workflow difficult in bolus tracking of DCE-MRI.
We present a new method for automated slice positioning for
2D MRA used to monitor bolus arrival. The 2D plane was
planned by aorta detection using both Hough Forests and
AdaBoost classifiers following the classification of axial
images. A dataset with 40 patients was tested, and 35 cases
depicted the cross section of the aorta clearly. This
automation will help the operator and decrease the total
study time.
|
|
16:54
|
0249.
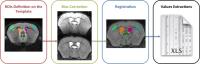 |
Automatic Pipeline for Regional Brain Analyses in Demyelinated
Mice 
Emilie Poirion1, Daniel Garcia Lorenzo1,
Isaac Adanyeguh1, Marie-Stéphane Aigrot1,
Alexandra Petiet2, and Bruno Stankoff1,3
1Brain and Spine Institute, INSERM U1127/CNRS UMR
7225, Sorbonnes Universités, UPMC, CHU Pitié-Salpêtrière, 47
Bd de l'hôpital, 75013 Paris, Paris, France, 2Brain
and Spine Institute, Center for Neuroimaging Research (CENIR),
CHU Pitié-Salpêtrière, 47 Bd de l'hôpital, 75013 Paris,
Paris, France, 3AP-HP,
Saint Antoine Hospital, Department of Neurology, 184 Bd du
Faubourg Saint Antoine, 75012 Paris, Paris, France
Experimental studies in mouse models offer the opportunity
to combine in-vivo longitudinal
high-field MRI and histological analyses. However, automatic
MRI tools for processing rodent data to avoid manual
processing are lacking. We proposed an automatic pipeline to
perform systematic analyses on large murine cohorts with
longitudinal data. We first applied artifacts correction as
bias correction to optimize the subsequent steps. We then
registered masks of regions of interest (ROIs) for our
analyses onto each subject from which we extracted the
quantitative data. This pipeline provides a way of quickly
analyzing ROI regardless of disease models or the MRI
sequence.
|
|
17:06
|
0250.
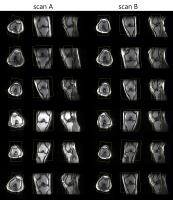 |
Improving robustness in automated slice positioning for knee MR
by combining landmark detection and image processing 
Takamasa Sugiura1, Shuhei Nitta1,
Taichiro Shiodera1, Yuko Hara1,
Yasunori Taguchi1, Tomoyuki Takeguchi1,
Takuya Fujimaki2, Kensuke Shinoda2,
Hiroshi Takai2, and Ayako Ninomiya2
1TOSHIBA CORPORATION, Kawasaki, Japan, 2TOSHIBA
MEDICAL SYSTEMS CORPORATION, Otawara, Japan
We propose an improved automatic slice positioning algorithm
for knee MR which combines conventional machine-learning
based landmark detection with advanced image processing
techniques. Conventional slice positioning methods determine
the diagnostic slice center and orientation by detecting
anatomical landmarks in the scout image. However, computing
slice positions from landmarks can be inadequate since
landmarks vary across patients and can be cut-off from scout
images. Here, we use not only landmark detection but also
image processing based contour detection of the femoral
condyle and angle estimation of the femur and tibia to
enable slice positioning for a wider range of scout images.
|
|
17:18
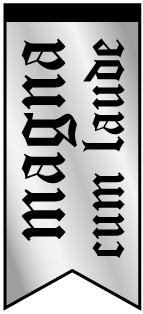 |
0251.
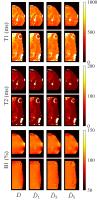 |
3D magnetic resonance fingerprinting with a clustered
spatiotemporal dictionary 
Pedro A. Gómez1,2, Guido Buonincontri3,
Miguel Molina-Romero1,2, Cagdas Ulas1,2,
Jonatahn I. Sperl2, Marion I. Menzel2,
and Bjoern H. Menze1
1Technische Universität München, Garching,
Germany, 2GE
Global Research, Garching, Germany, 3Istituto
Nazionale di Fisica Nucleare, Pisa, Italy
We present a method for creating a spatiotemporal dictionary
for magnetic resonance fingerprinting (MRF). Our technique
is based on the clustering of multi-parametric spatial
kernels from training data and the posterior simulation of a
temporal fingerprint for each voxel in every cluster. We
show that the parametric maps estimated with a clustered
dictionary agree with maps estimated with a full dictionary,
and are also robust to undersampling and shorter sequences,
leading to increased efficiency in parameter mapping with
MRF.
|
|
17:30
|
0252.
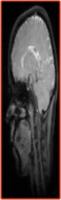 |
Multi-dimensional phase unwrapping: a new and efficient linear
algebraic formulation using weighted least-squares - Permission Withheld
Laurent Lamalle1,2, Georgios Gousios3,
and Matthieu Urvoy3
1Inserm US 17 & CNRS UMS 3552, Grenoble, France, 2Université
Joseph Fourier & CHU de Grenoble, UMS IRMaGe, Grenoble,
France, 3SFR
RMN Biomédicale et Neurosciences, Université Joseph Fourier,
Grenoble, France
Phase information of MR images can provide quantitative
access to various physical properties of the examined
sample, such as local $$$B_0$$$ values, magnetic
susceptibility or flow. Phase is a continuous information
whose estimation typically requires unwrapping. In this
study, we propose a novel phase estimation algorithm which:
(1) relies on a numeric scheme that is robust to phase
jumps, and (2) is optimized for execution on modern parallel
processors.
|
|
17:42
|
0253.
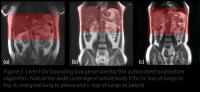 |
Fast liver FOV localization for improved liver-MRI workflow - Permission Withheld
Arathi Sreekumari1, K S Shriram1, Uday
Patil1, Ersin Bayram2, Dattesh
Shanbhag1, and Rakesh Mullick1
1GE Global Research, Bangalore, India, 2GE
Healthcare, Houston, TX, United States
In this work we are focusing on automating the scan coverage
and FOV for liver MRI acquisitions. We demonstrate that
using fast scout images, we can achieve very good
localization of liver FOV, irrespective of anatomy
differences and hand-up / hands-down positioning.
|
|
17:54
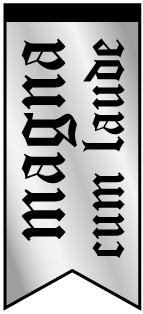 |
0254.
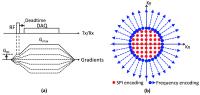 |
Simultaneous measurement of short and long T2* components using
hybrid encoding 
Hyungseok Jang1,2, Curtis N Wiens1,
and Alan B McMillan1
1Department of Radiology, University of
Wisconsin, Madison, WI, United States, 2Department
of Electrical and Computer Engineering, University of
Wisconsin, Madison, WI, United States
In this study, we propose a highly time efficient
quantitative imaging scheme where short and long T2*
components can be simultaneously estimated. This method is
based on a multi-echo UTE hybrid encoding scheme, where the
central SPI region is oversampled to allow measurement of
short T2* across a wide range of TEs. The UTE acquisition is
immediately followed by a gradient echo train to measure
long T2*. We show the proposed method can obtain an
extensive number of images (e.g., approximately 750 images)
within a single acquisition and reasonable scan time.
|
|
18:06
|
0255.
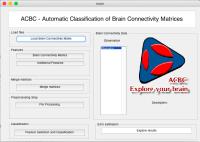 |
Automatic Classification of Brain Connectivity Matrices - a
toolbox for supporting neuropsychiatric diagnosis 
Ricardo Jorge Maximiano1, Tiago Constantino1,2,3,
André Santos-Ribeiro1,4, and Hugo Alexandre
Ferreira1
1Institute of Biophysics and Biomedical
Engineering, Faculty of Sciences of the University of
Lisbon, Lisbon, Portugal, 2Spitalzentrum
Biel, Bienne, Switzerland, 3Lisbon
School of Health Technology - ESTeSL, Lisbon, Portugal, 4Centre
for Neuropsychopharmacology, Imperial College London,
London, United Kingdom
In this work, a user-friendly toolbox that aims to classify
automatically brain connectivity matrices is described. To
test this tool, we used the Parkinson’s Progression Markers
Initiative (PPMI) data which includes structural and
functional Magnetic Resonance Imaging data of healthy
subjects, patients with “scans without evidence for
dopaminergic deficit” (SWEDD) and patients diagnosed with
Parkinson’s Disease (PD). Using default parameters, this
tool was able to achieve a maximum accuracy of 85.4% in
classifying the 3 groups of subjects by selecting features
that were related to the rostral middle frontal gyrus and
splenium, which are in agreement with PD literature.
|
|
18:18
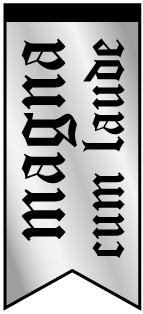 |
0256.
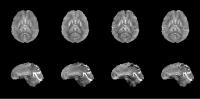 |
Rapid Two-Step QSM Without A Priori Information 
Christian Kames1,2, Vanessa Wiggermann1,3,4,
and Alexander Rauscher1,4
1UBC MRI Research Centre, University of British
Columbia, Vancouver, BC, Canada, 2Department
of Engineering Physics, University of British Columbia,
Vancouver, BC, Canada, 3Department
of Physics and Astronomy, University of British Columbia,
Vancouver, BC, Canada, 4Department
of Pediatrics, University of British Columbia, Vancouver,
BC, Canada
Current state-of-the-art QSM reconstruction algorithms are
plagued by the trade-off between reconstruction speed and
quality. We propose a novel two-step dipole inversion
algorithm 20x faster than MEDI and HEIDI, while producing
qualitatively appealing images with a root-mean-square error
less than MEDI’s and HEIDI’s when compared to COSMOS. The
proposed method works by first reconstructing the
well-conditioned k-space region through the use of a Krylov
subspace solver, followed by a total variation minimization
to fill in the ill-conditioned k-space region.
|
|