BY JESSICA MCKAY
This month’s Editor’s Pick features a project that makes kurtosis imaging more accessible to clinicians and researchers, alike, from a group in Denmark that includes our interviewees: Brian Hansen and Sune Jespersen. Diffusion kurtosis imaging (DKI) increases sensitivity to microstructural changes by extending the diffusion signal expression to account for non-Gaussian effects, but it typically requires time-consuming acquisitions with high diffusion weighting. Brian and Sune’s group had previously described a fast protocol, referred to as the 1-3-9 scheme, that includes three diffusion directions at a low b-value to determine the mean diffusivity and 9 specific diffusion directions at a higher b-value to calculate mean kurtosis [Hansen et al. MRM 69, 2013]. In this work, they extend the protocol to make it more robust to experimental imperfections by acquiring all 9 directions at the lower b-value, which they call 1-9-9. They further characterize the optimum b-values and propose a method to correct for imperfect diffusion directions. Keep reading to find out how their acquisition scheme expands the clinical value and feasibility of kurtosis imaging and you may even be inspired to add this ~1-minute scan onto your own protocol.
MRMH: Tell us a little bit about yourselves. What lead you into this project?
Brian: I am a physicist, biomedical engineering-type. I have been interested in microstructural imaging for a while, but the experiments are very remote from the clinical applications. This project attempts to lower the data demands of DKI and make microstructural imaging techniques more suited for the clinic.
Sune: My background is in theoretical physics, and I am interested in how the diffusion signal reports on microstructure and what information is actually contained in the diffusion signal. I have mostly been working with modeling, but kurtosis imaging is a different approach that is more generic. It is very sensitive but not very specific; nevertheless, it may be a valuable biomarker. It is not very widespread in the clinic yet, so we wanted to lower the barrier: both the analysis and the acquisition times. People are less likely to adopt a time-consuming protocol, especially since they are still not sure how kurtosis might be used. If they can just use a one-minute sequence, it is easier to explore its possible uses and to persuade doctors to add it to their exams.
MRMH: How long does your DKI protocol take and how does that compare to a standard DKI acquisition?
Brian: Most people use a 2-shell acquisition with ~30 directions for each b-value in 7 to 10 minutes. With our protocol, we can reduce that to about a minute. That might not sound so impressive, but it makes a big difference for clinicians and can be added cheaply to your protocol.
MRMH: Well a factor of 7 to 10 is pretty impressive!
Sune: I think so, too! You can also trade the time gain for SNR. If you are willing to sacrifice those extra 6 minutes, who wants to say ‘no thanks’ to extra SNR?
MRMH: Kurtosis is something that a lot of us keep hearing about, but many don’t really understand what it is.
Brian: The diffusion kurtosis framework contains diffusion tensor imaging (DTI), but it takes non-Gaussian effects into account. By including kurtosis you partially account for the effects of the microstructure on the diffusion and indirectly become more sensitive to the microstructure.
MRMH: Is DKI more challenging that DTI?
Brian: Not really in principle, but in practice DKI has some additional experimental challenges. It is acquired with the same sequence as DTI, but with higher b-values where you are closer to a low SNR limit.
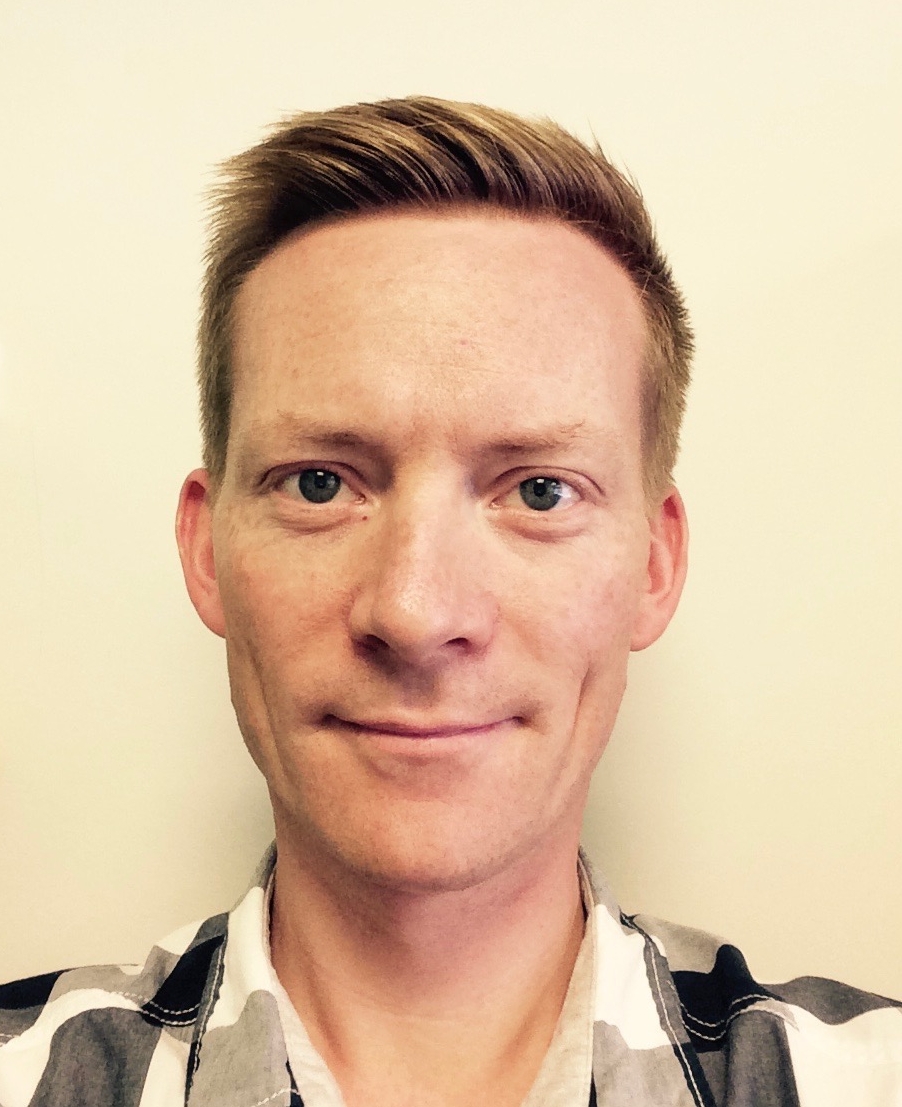
Sune Jespersen.
Sune: The higher gradients also make things like eddy currents more problematic, and you need more directions with those higher gradients. The estimation problem is also harder because you have more parameters to estimate: 22 in DKI vs. 7 for DTI.
Brian: The fitting is a difficult numerical problem and will be influenced by noise. One advantage of our technique is that it doesn’t involve fitting. It calculates the mean kurtosis from a weighted sum of log signals, so that removes a whole set of problems related to analysis.
MRMH: Is the computation time limiting?
Brian: Yes, especially in an acute setting, such as a stroke patient. It can take hours to perform the DKI post processing, so most clinicians would say, ‘we can’t use that for decision making; we could perhaps use it for follow up’, which limits the clinical applicability. In our technique, since there is no optimization procedure, the post processing can be done very rapidly. Jurgen Finsterbusch (co-author) implemented an online reconstruction of these parameter maps for Siemens systems. The code is available as a Siemens c2p sequence. That way the clinician has the maps available to him/her immediately.
Sune: Even for cohort studies, a small computation time is convenient. We all know that in research you rarely have to do anything only once because we often make mistakes the first time around. So, two hours for each brain is a bit of a hurdle.
MRMH: Why might a clinician care about the resulting parameter maps?
Sune: It is not a routine clinical tool yet, but many groups have shown that in various diseases DKI contains complementary information to the DTI parameters.
Brian: DKI has increased sensitivity to microstructural changes. For instance in stroke, DKI has been shown to outline the lesion better than the typical DTI-derived measures. It is also possible to detect more subtle changes earlier in the disease progression for earlier diagnosis, which is crucial for many diseases.
MRMH: Are there any body applications?
Brian: There’s growing interest for DKI in the body. We recently published a paper on fast DKI of renal fibrosis, where biopsy is often used to monitor disease, but with many complications. It would be great if we could replace those biopsies with a scan.
MRMH: What makes 9 the magic number of directions to measure kurtosis?
Brian: When you measure mean diffusivity you can measure along just three orthogonal directions because the mean diffusivity is proportional to the trace of the tensor. Similarly, we define the mean kurtosis proportional to the trace of the kurtosis tensor so that you can use the same trick, but the kurtosis tensor is bigger so three directions are not enough. The directions you need were derived by Sune… by magic. On a more serious note: we provided a detailed derivation that explains the need for these specific directions. It’s available, here, as online supplementary materials for the 1-3-9 paper.
Sune: For DKI you need extra directions to remove unwanted cross-terms, and that turns out to be 9: three orthogonal directions and two directions for each cross term.
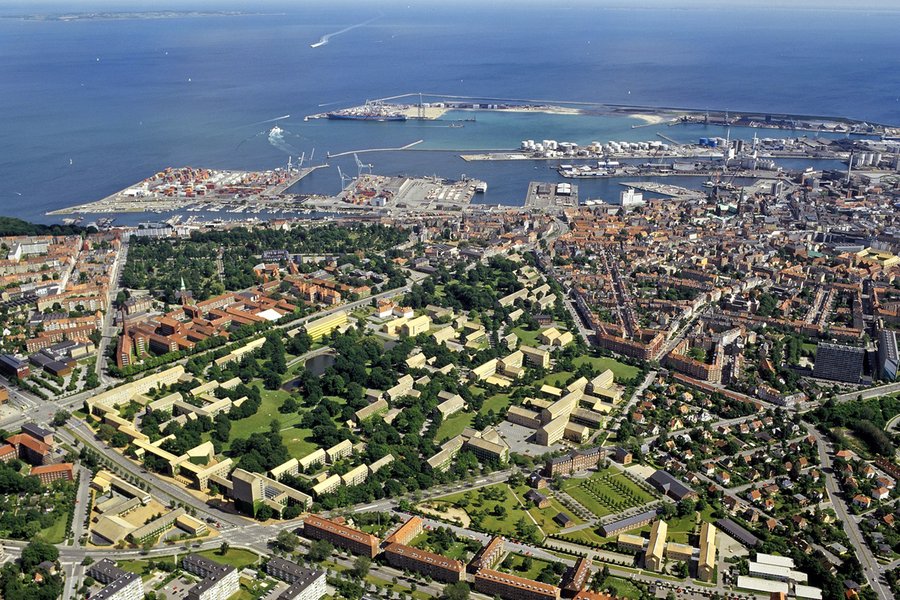
View of Aarhus University in Denmark.
MRMR: Are the kurtosis tensor and fractional anisotropy (FA) related?
Brian: In general, the FA relates to the diffusion tensor and is not connected to the kurtosis tensor.
Sune: You can actually have a situation where you have zero FA, but you still have an anisotropic diffusion kurtosis tensor. In practice they might tend to follow, but theoretically speaking they are independent.
MRMH: Where might this project go next?
Sune: We are trying to extend this to not only get the mean kurtosis but also to get two other commonly used kurtosis measurements, axial kurtosis and radial kurtosis. We can estimate basically all kurtosis measures in current use with our protocol.
MRMH: How can other researchers in your field apply your conclusions to their own work?
Sune: We are hoping that many more researchers would be interested in applying this technique, especially in the body. When my collaborators do diffusion imaging in the body they often use trace imaging. This work is basically an extension of that approach to kurtosis, and it might help people find out if kurtosis is a useful biomarker or not.
Brian: We’d also like to say that if anybody reading this is interested in applying the fast kurtosis technique we are very happy to help set up the method, test pilot data quality, and provide analysis scripts. Look us up on http://www.cfin.au.dk.