10:30 |
0740. |
Dynamic MRI Reconstruction
using Low-Rank plus Sparse model with Optimal Rank
Regularized Eigen-Shrinkage 
Brian E. Moore1, Rajesh R. Nadakuditi1,
and Jeffrey A. Fessler1
1Department of Electrical Engineering and
Computer Science, University of Michigan, Ann Arbor, MI,
United States
Low-rank plus sparse matrix decomposition algorithms
have seen fruitful application in dynamic
contrast-enhanced MRI because the data is well modeled
as the superposition of a low-rank static background and
temporally sparse dynamic contrast enhancement. In this
setting, we propose a novel algorithm that replaces the
standard nuclear norm with a recently discovered optimal
rank regularizer from random matrix theory. This
regularizer preserves the quality of high
signal-to-noise ratio image features while maintaining
data compressibility, resulting in better qualitative
and quantitative image reconstruction than existing
nuclear norm techniques. We substantiate these claims on
undersampled multicoil cardiac perfusion MRI data.
|
10:42 |
0741. |
Calibration for Parallel
MRI Using Robust Low-Rank Matrix Completion 
Dan Zhu1, Martin Uecker2, Joseph Y
Cheng3, Zhongyuan Bi1, Kui Ying4,
and Michael Lustig2
1Biomedical Engineering, Tsinghua University,
Beijing, China, 2Electrical
Engineering and Computer Sciences, University of
California, Berkeley, California, United States, 3Electrical
Engineering, Stanford University, California, United
States, 4Department
of Engineering Physics, Tsinghua University, Beijing,
China
The goal of this work is to develop a practical
calibration method for parallel MRI which is robust
against both under-sampling and corruption of the
calibration data. Previously, it has been demonstrated
that robust low-rank matrix completion can reconstruct
corrupted and under-sampled k-space data without
specific auto-calibration data (ACS). Here, we show a
generalized formulation for motion-robust
auto-calibration and reconstruction from under-sampled
data that is incorporated into ESPIRiT. The method is
general and can incorporate navigation information when
available. The feasibility of the method was
demonstrated in simulation and in-vivo experiments.
|
10:54 |
0742. |
Motion-guided low-rank plus
sparse (L+S) reconstruction for free-breathing dynamic MRI 
Ricardo Otazo1, Thomas Koesters1,
Emmanuel Candès2, and Daniel K Sodickson1
1Bernard and Irene Schwartz Center for
Biomedical Imaging, Department of Radiology, New York
University School of Medicine, New York, NY, United
States, 2Departments
of Mathematics and Statistics, Stanford University,
Stanford, CA, United States
A non-rigid motion model is incorporated into the
low-rank plus sparse (L+S) reconstruction approach for
free-breathing accelerated dynamic MRI with separation
of background and dynamic components. The motion
operator registers the time-series of images, which
improves low-rank and sparsity conditions along the
temporal domain and enhances temporal fidelity in the
reconstructed images. The motion-guided L+S
reconstruction approach is tested in prospectively
undersampled dynamic cardiac and abdominal data sets
acquired during free-breathing.
|
11:06 |
0743.
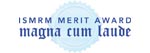 |
Real-Time Phase Contrast
Cardiovascular Flow Imaging with Joint Low-Rank and Sparsity
Constraints 
Bo Zhao1, Aiqi Sun2, Ke Ma2,
Rui Li2, Anthony Christodoulou1,
Chun Yuan2,3, and Zhi-Pei Liang1
1Department of Electrical and Computer
Engineering and Beckman Institute, University of
Illinois at Urbana-Champaign, Urbana, IL, United States,2Department
of Biomedical Engineering and Center for Biomedical
Imaging Research, Tsinghua University, Beijing, China, 3Department
of Radiology, University of Washington, WA, United
States
A novel method is presented for real-time phase contrast
(PC) cardiovascular flow imaging. It integrates a
real-time data acquisition scheme (without ECG gating
and respiration control) with constrained reconstruction
using joint low-rank and sparsity constraints. It
achieves a temporal resolution of 34.4 msec and spatial
resolution of 2.58 mm in a 2D real-time flow imaging
experiment with three velocity encoding directions. The
method should prove useful for real-time cardiovascular
flow imaging.
|
11:18 |
0744. |
MRI Reconstruction by
Learning the Dictionary of Spatialfrequency-Bands
Correlation: A novel algorithm integratable with PI and CS
to further push acceleration 
Enhao Gong1 and
John M Pauly1
1Electrical Engineering, Stanford University,
Stanford, CA, United States
Parallel Imaging (PI) and Compressed Sensing (CS) enable
MR acceleration by exploiting channel-correlation and
sparsity. However, the acceleration capability is
limited by channel-encoding, increased noise and blurred
details. In this work, a novel algorithm is proposed to
further improve the undersampled MRI reconstruction by
exploiting the correlation between image details in
different bands of spatial-frequencies. Dictionaries of
image patches in different spatial-frequency bands were
learned from database and undersampled MR images were
reconstructed by solving as a sparse representation of
the dictionary. The proposed algorithm demonstrated
great advantages and were integrated with PI-CS to
further push acceleration.
|
11:30 |
0745.
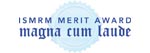 |
P-LORAKS: Low-rank modeling
of local k-space
neighborhoods with parallel imaging data 
Jingwei Zhuo1,2 and
Justin P. Haldar1
1Signal and Image Processing Institute,
University of Southern California, Los Angeles, CA,
United States, 2Electronic
Engineering, Tsinghua University, China
This work presents P-LORAKS, a novel approach to
constrained image reconstruction from parallel imaging
data. Similar to the original LORAKS (low-rank matrix
modeling of local k-space
neighborhoods) method, P-LORAKS uses low-rank matrix
models to generate parsimonious constrained
reconstruction representations of images with limited
spatial support and/or slowly varying phase. Combining
LORAKS with parallel imaging data leads to further
improvements in image reconstruction quality. Results
are illustrated with real data, where P-LORAKS compares
favorably to existing parallel imaging methods like
SPIRiT and SAKE.
|
11:42 |
0746.
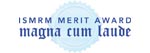 |
MR Image Reconstruction
Exploiting Nonlinear Transforms 
Johannes F. M. Schmidt1 and
Sebastian Kozerke1,2
1Institute for Biomedical Engineering,
University and ETH Zurich, Zurich, Switzerland, 2Imaging
Sciences and Biomedical Engineering, King's College
London, London, United Kingdom
MR image reconstruction exploiting nonlinear transforms
has successfully been implemented using projections onto
principal components in a high-dimensional kernel
feature space, employing kernel PCA. Image quality was
found to improve considerably relative to standard CS
reconstruction with wavelet and finite-differences
transforms.
|
11:54 |
0747. |
Motion Compensated Dynamic
Imaging without Explicit Motion Estimation -
permission withheld
Yasir Q Mohsin1, Zhili Yang2,
Sajan Goud Lingala3, and Mathews Jacob4
1Electrical Eng, University of Iowa, Iowa
City, IA, United States, 2Electrical
Engineering, Univeristy of Rochester, NY, United States, 3BME,
University of Iowa, IA, United States, 4Electrical
Eng, University of Iowa, IA, United States
The focus of this abstract is to recover dynamic MRI
data from highly under-sampled measurements. Compressed
sensing schemes that exploit sparsity in Fourier and
gradient domains have enjoyed a lot of success in
breath-held cardiac MRI. However, these schemes often
result in un-acceptable spatio-temporal blurring and
residual alias artifacts, when applied to free breathing
cardiac MRI with or without cardiac gating. The main
reason is the high inter-frame motion, often introduced
by respiration. Methods that combine motion estimation
and compensation (ME-MC) have been shown to improve the
results in this context, but they come with considerably
increased computational complexity. In addition, the
joint estimation of the motion model parameters and the
signal involves a complex non-convex optimization
criterion, which is often difficult to solve. Our main
objective is to introduce a novel framework for
motion-compensated dynamic MR image recovery that does
not suffer from the above mentioned drawbacks.
|
12:06 |
0748. |
Integrating Principal
Component Analysis and Dictionary Learning with Coherence
Constraint for Fast T1 Mapping 
Yanjie Zhu1, Qiegen Liu2, Qinwei
Zhang3, Jing Yuan3, and Dong Liang1
1Paul C. Lauterbur Research Centre for
Biomedical Imaging, Shenzhen Institutes of Advanced
Technology, Chinese Academy of Sciences, Shenzhen,
Guangdong, China, 2Department
of Electronic Information Engineering, Nanchang
University, Nanchang, Jiangxi, China, 3Department
of Imaging and Interventional Radiology, The Chinese
University of Hong Kong, Shatin, Hong Kong
Long scanning time hinders the widespread application of
T1¦Ñ in clinics. A new approach utilizing the advantages
of both fixed and adaptive transform is proposed to
accelerate T1¦Ñ imaging under the framework of
compressed sensing. Specifically, PCA is applied first
along the parameter direction, and the dictionary
learning technique is used to reconstruct the PC
coefficients. Additionally, a coherence constraint is
introduced to guarantee the sparse representation
ability of learned dictionary. Experimental results
demonstrate that the proposed method can improve the
accuracy of estimated T1¦Ñ map compared with the one
without coherence constraint and conventional dictionary
learning based method.
|
12:18 |
0749. |
Compressed Sensing MRI
Exploiting Complementary Dual Decomposition 
Suhyung Park1, Chul-Ho Sohn2, and
Jaeseok Park1
1Department of Brain and Cognitive
Engineering, Korea University, Seoul, Seoul, Korea, 2Department
of Radiology, Seoul National University Hospital, Seoul,
Korea
Compressed sensing (CS) [1,2] exploits the sparsity of
an image in a transform domain. However, it has been
shown that CS suffers particularly from loss of low
contrast image features with increasing reduction
factor. To retain image details, in this work we
introduce a novel CS algorithm exploiting feature-based
complementary dual decomposition with joint estimation
of local scale mixture (LSM) model and images. Images
are decomposed into dual block sparse components: total
variation (TV) for piecewise smooth parts and wavelets
for residuals. The LSM model parameters of residuals in
the wavelet domain are estimated and then employed as a
regional constraint in spatially adaptive reconstruction
of high frequency subbands to restore image details
missing in piecewise smooth parts. Experiments
demonstrate the superior performance of the proposed
method in preserving low-contrast image features even at
high reduction factors.
|
|