08:00 |
1023.
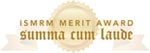 |
Noise map estimation in
diffusion MRI using Random Matrix Theory 
Jelle Veraart1, Els Fieremans1,
and Dmitry S. Novikov1
1Center for Biomedical Imaging, NYU Langone
Medical Center, New York, NY, United States
We propose a new technique to estimate the spatially
varying noise map based on diffusion MRI data to enable
Rician bias correction. The technique makes use of a
random matrix theorem, i.e. Marchenko-Pastor’s law, to
estimate the noise level by exploiting the redundancy in
multi-directional diffusion MR data.
|
08:12 |
1024. |
Caveats of non-linear
fitting to brain tissue models of diffusion 
Ileana O. Jelescu1, Jelle Veraart1,
Els Fieremans1, and Dmitry S. Novikov1
1Center for Biomedical Imaging, Dept. of
Radiology, NYU Langone Medical Center, New York, New
York, United States
Compared to DTI, white/gray matter models of diffusion
should have improved specificity. However, fit outputs
notoriously suffer from bias and poor precision, with
most models employing simplifying assumptions to
stabilize the fit. Here, we use the example of NODDI to
assess the behavior of nonlinear fitting when all model
parameters are free. We reveal that the typical full
model of brain tissue cannot be reliably determined, due
to a duality of solutions, and to the narrow and shallow
(boomerang-shaped) minimization landscape. Constraining
the fit with fixed parameter values that lack biological
validation is not a trustworthy solution to the problem.
|
08:24 |
1025.
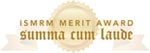 |
Joint estimation of
microstructural and biomechanical features of the brain
using a phase sensitive reconstruction of DWIs 
Tim Sprenger1,2, Jonathan I. Sperl2,
Axel Haase1, Brice Fernandez3,
Christopher Hardy4, Luca Marinelli4,
Michael Czisch5, Philipp Saemann5,
and Marion I. Menzel2
1IMETUM, Technical University, Munich,
Germany, 2GE
Global Research, Munich, Germany, 3GE
Healthcare, Munich, Germany, 4GE
Global Research, Niskayuna, NY, United States, 5Max
Planck Institute of Psychiatry, Munich, Select, Germany
Diffusion weighted magnetic resonance imaging (DWI)
allows for non-invasive measurement of microstructural
features of the human brain. Usually all data processing
in DWI is based on the magnitude of the complex MR
signal, and the inherent phase of the signal is
discarded as it is considered to be spoiled by different
sources. One source for a non-zero phase in DWI signal
is the pulsation of the brain itself, which is
potentially impacted by disorders such as hydrocephalus
or traumatic brain injury. In this work, a phase
correction approach is introduced to sequentially remove
all phase contributions except for brain pulsation.
|
08:36 |
1026. |
A compressed sensing
approach to super-resolution diffusion MRI from multiple
low-resolution images 
Lipeng Ning1,2, Kawin Setsompop2,3,
Cornelius Eichner3, Oleg Michailovich4,
Carl-Fredrik Westin1,2, and Yogesh Rathi1,2
1Brigham and Women's Hospital, Boston, MA,
United States, 2Harvard
Medical School, Boston, MA, United States, 3Massachusetts
General Hospital, MA, United States, 4University
of Waterloo, Ontario, Canada
We present a novel compressed sensing approach for super
resolution reconstruction (SRR) of diffusion MRI using
multiple anisotropic low-resolution images. The
diffusion signal in each voxel is estimated using
spherical ridgelets while the spatial correlation
between neighboring voxels is accounted for using
total-variation (TV) regularization. The experimental
result using in-vivo human brain data shows that the
proposed SRR method is capable of recovering complex
fiber orientations at a very high spatial resolution,
similar to a physically acquired “gold-standard” data.
Hence it has potential to be applied in clinical
settings to study mental diseases and to reduce
partial-volume effect.
|
08:48 |
1027.
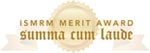 |
Time to move on: an
FOD-based DEC map to replace DTI's trademark DEC FA 
Thijs Dhollander1, Robert Elton Smith1,
Jacques-Donald Tournier2,3, Ben Jeurissen4,
and Alan Connelly1,5
1The Florey Institute of Neuroscience and
Mental Health, Melbourne, Victoria, Australia, 2Centre
for the Developing Brain, King's College London, London,
United Kingdom, 3Department
of Biomedical Engineering, King's College London,
London, United Kingdom, 4iMinds-Vision
Lab, University of Antwerp, Antwerp, Belgium, 5The
Florey Department of Neuroscience, University of
Melbourne, Melbourne, Victoria, Australia
The "traditional" directionally-encoded colour (DEC) FA
map is an icon of DTI, but is also affected by its
inherent flaws. The first eigenvector is known to be
ill-defined in regions of crossing fibres, resulting in
misleading specific DEC values as well as "false edges"
in the overall map. Additionally, the FA shows naturally
low values in these regions. In a clinical setting, this
might potentially lead to false positive findings; but
also to false negative ones in case these false features
mask out or otherwise distract from real pathological
features. We propose an FOD-based DEC map that solves
these issues.
|
09:00 |
1028. |
Resolving crossing fibers
and generalizing biomarkers using the diffusion kurtosis
tensor 
Rafael Neto Henriques1, Marta Morgado Correia1,
Rita Gouveia Nunes2, and Hugo Alexandre
Ferreira2
1Cognition and Brain Science Unit, MRC,
Cambridge, England, United Kingdom, 2Instituto
de Biofisica e Engenharia Biomedica, Faculdade de
Ciencias da Universidade de Lisboa, Lisbon, Lisbon,
Portugal
Diffusion Kurtosis Imaging (DKI) models the non-Gaussian
behaviour of water diffusion by the diffusion kurtosis
tensor (KT), which can be used to provide indices of
tissue heterogeneity and a better characterisation of
the spatial architecture of tissue microstructure. In
this study, the advantages and disadvantages of using KT
based fiber direction estimates to resolve crossing
fibers and compute a generalized version of radial
kurtosis (RK) are investigated. Our results show that KT
fiber direction estimates provides smaller angular
errors when compared to previous DKI fiber estimation
procedures and RK measures less sensitive to noise bias.
|
09:12 |
1029.
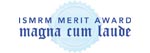 |
Comparing Fourier to SHORE
Basis Functions for Sparse DSI Reconstruction 
Alexandra Tobisch1,2, Thomas Schultz2,
Rüdiger Stirnberg1, Gabriel Varela3,
Hans Knutsson4, Pablo Irarrázaval3,5,
and Tony Stöcker1,6
1German Center for Neurodegenerative
Diseases, Bonn, Germany, 2Department
of Computer Science, University of Bonn, Bonn, Germany, 3Biomedical
Imaging Center, Pontificia Universidad Católica de
Chile, Santiago, Chile, 4Linköping
University, Linköping, Sweden, 5Department
of Electrical Engineering, Pontificia Universidad
Católica de Chile, Santiago, Chile, 6Department
of Physics and Astronomy, University of Bonn, Bonn,
Germany
Compressed Sensing (CS) theory accelerates Diffusion
Spectrum Imaging (DSI) acquisition, while still
providing high angular and radial resolution of
intra-voxel microstructure. Several groups have proposed
to reconstruct the diffusion propagator from sparse
q-space samples by fitting continuous basis functions.
Among these, the SHORE basis has recently been found to
perform best. This work compares the SHORE-based
approach to traditional CS recovery that combines the
discrete Fourier transform with a sparsity term. For
simulated diffusion signals, the CS reconstruction is
found to deviate less from the ground truth when using
Fourier basis functions for sparse DSI reconstruction.
|
09:24 |
1030. |
How to avoid biased
streamlines-based metrics for streamlines with variable step
sizes 
Jean-Christophe Houde1, Marc-Alexandre
Côté-Harnois1, and Maxime Descoteaux1
1Computer Science department, Université de
Sherbrooke, Sherbrooke, Quebec, Canada
We show that metrics computed over streamlines can
easily be biased or incorrect for streamlines with a
step size that is too large or variable. The basic
methods to compute those statistics, sometimes called
Tractometry methods, generally only use the points of
the streamlines to sample the corresponding image
volumes. However, for streamlines where the step size is
too large or variable, this sampling is skewed, and
derived metrics are biased. We present a simple updated
method that correctly handles those streamlines, and we
show that metrics computed using this method are robust
to the streamline sampling.
|
09:36 |
1031.
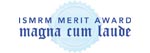 |
Imposing label priors in
global tractography can resolve crossing fibre ambiguities 
Daan Christiaens1,2, Frederik Maes1,2,
Stefan Sunaert2,3, and Paul Suetens1,2
1Electrical Engineering, KU Leuven, Leuven,
Vlaams-Brabant, Belgium, 2Medical
Imaging Research Center, UZ Leuven, Leuven,
Vlaams-Brabant, Belgium,3Translational MRI,
KU Leuven, Leuven, Vlaams-Brabant, Belgium
Ambiguity in the local diffusion profile is an important
cause of spurious fibre tracks in DWI. We propose to use
bundle labels as an additional prior in global
tractography to overcome this issue. We have evaluated
such label prior in a Tractometer study, and
demonstrated its effect on real data using the Catani
atlases of 30 white matter tracts. Results show an
important decrease of invalid connections and spurious
tracks. Additionally, a probabilistic segmentation of
the fibre bundles is obtained.
|
09:48 |
1032. |
Connectivity based
segmentation of the Corpus Callosum using a novel data
mining approach 
Gowtham Atluri1, An Wu2, Essa
Yacoub2, Kamil Ugurbil2, Vipin
Kumar1, and Christophe Lenglet2
1Computer Science and Engineering, University
of Minnesota, Minneapolis, Minnesota, United States, 2Center
for Magnetic Resonance Research, University of
Minnesota, Minneapolis, Minnesota, United States
Existing approaches that are used to do finer
segmentation of cortical regions using DTI based
tractography data do not make use of the underlying
spatial structure. In this work, we extend a popular
Shared Nearest Neighbor (SNN) based clustering approach
in order to account for spatial structure in the data.
We use this approach to discover finer segmentation of
the Corpus Callosum in 2 normal subjects using
tractography data computed from a 3T and a 7T DTI scan.
Our results suggest that our new approach results in a
segmentation that is consistent between 3T and 7T.
|
|