|
16:00
|
1040.
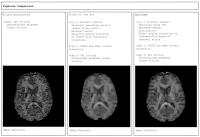 |
Diffusion parameter EStImation with Gibbs and NoisE Removal
(DESIGNER) 
Benjamin Ades-Aron1, Jelle Veraart1,2,
Elias Kellner3, Yvonne W. Lui1, Dmitry
S. Novikov1, and Els Fieremans1
1Center for Biomedical Imaging, New York
University School of Medicine, New York, NY, United States, 2iMinds
Vision Lab, University of Anterp, Antwerp, Belgium, 3Department
of Radiology, University Medical Center Freiburg, Freiburg,
Germany
We propose a new pipeline (DESIGNER) for diffusion image
processing that includes Marchenko Pastur denoising and
Gibbs artifact removal, and thereby improves the precision
and accuracy of the diffusion tensor and kurtosis tensor
parameter estimation. In particular, our results show no
notorious black voxels on kurtosis maps, while the original
resolution is maintained in contrast to state-of-the-art
processing methods that apply smoothing.
|
|
16:12
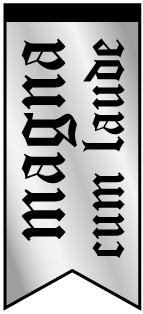 |
1041.
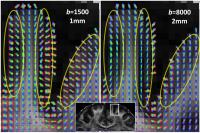 |
HIgh B-value and high Resolution Integrated Diffusion (HIBRID)
Imaging 
Qiuyun Fan1, Aapo Nummenmaa1, Jonathan
R. Polimeni1, Thomas Witzel1, Susie Y.
Huang1, Van J. Wedeen1, Bruce R. Rosen1,2,
and Lawrence L. Wald1,2
1Massachusetts General Hospital, Boston, MA,
United States, 2Harvard-MIT
Division of Health Sciences and Technology, MIT, Cambridge,
MA, United States
The cerebral cortex is rich in gyral folding. Axonal fibers
take sharp turns when bending into the cortex. High
resolution diffusion MRI is needed to characterize cortical
structures in finer scale, while high b-value
is desired to resolve complex white matter structures. We
examined the impact of imaging resolution on characterizing
the radial diffusion pattern in cortex, and proposed to
improve the HIgh B-value
and high Resolution Integrated Diffusion (HIBRID) imaging by
incorporating information about each voxel’s proximity to
the cortex. The combined data demonstrated the desired
features from both high resolution and high b-value
diffusion imaging.
|
|
16:24
|
1042.
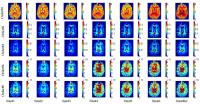 |
Harmonizing diffusion MRI data from multiple scanners 
Hengameh Mirzaalian1, Lipeng Ning1,
Peter Savadjiev1, Ofer Pasternak1,
Sylvain Bouix1, Oleg Michailovich2,
Marek Kubicki1, Carl Fredrik Westin1,
Martha E. Shenton1, and Yogesh Rathi1
1Harvard Medical School and Brigham and Women’s
Hospital, Boston, USA., Boston, MA, United States, 2University
of Waterloo, Toronto, ON, Canada
Diffusion MRI (dMRI) is increasing being used to study
neuropsychiatric brain disorders. To increase sample size
and statistical power of neuroscience studies, we need to
aggregate data from multiple sites1. However this
is a challenging problem due to the presence of inter-site
variability in the signal originating from several sources,
e.g. number of head coils and their sensitivity,
non-linearity in the imaging gradient, and other scanner
related parameters2. Prior works have addressed
this issue either using meta analysis3, or by
adding a statistical covariate4, which are not
model free and may produce erroneous results.
|
|
16:36
|
1043.
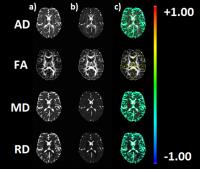 |
Free water elimination using a bi-tensor model improves
test-retest reproducibility of diffusion tensor imaging indices
in the brain: a longitudinal multisite reliability study of
healthy elderly subjects 
Angela Albi1, Ofer Pasternak2,
Ludovico Minati1,3, Moira Marizzoni4,
Giovanni Frisoni4,5, David Bartrés-Faz6,
Núria Bargalló7, Beatriz Bosch8, Paolo
Maria Rossini9,10, Camillo Marra11,
Bernhard Müller12, Ute Fiedler12, Jens
Wiltfang12,13, Luca Roccatagliata14,15,
Agnese Picco16, Flavio Mariano Nobili16,
Oliver Blin17, Julien Sein18,
Jean-Philippe Ranjeva18, Mira Didic19,20,
Stephanie Bombois21, Renaud Lopes21,
Régis Bordet21, Hélène Gros-Dagnac22,23,
Pierre Payoux22,23, Giada Zoccatelli24,
Franco Alessandrini24, Alberto Beltramello24,
Antonio Ferretti25,26, Massimo Caulo25,26,
Marco Aiello27, Carlo Cavaliere27,
Andrea Soricelli27,28, Lucilla Parnetti29,
Roberto Tarducci30, Piero Floridi31,
Magda Tsolaki32, Manos Constantinidis33,
Antonios Drevelegas34, and Jorge Jovicich1
1Center for Mind/Brain Sciences (CIMEC),
University of Trento, Rovereto, Trento, Rovereto (Trento),
Italy, 2Departments
of Psychiatry and Radiology, Brigham and Women's Hospital,
Harvard Medical School, Boston, Massachusetts, Boston, MA,
United States, 3Scientific
Department, Fondazione IRCCS Istituto Neurologico Carlo
Besta, Milan, Italy, Milan, Italy, 4LENITEM
Laboratory of Epidemiology, Neuroimaging, & Telemedicine —
IRCCS San Giovanni di Dio-FBF, Brescia, Italy, Brescia,
Italy, 5Memory
Clinic and LANVIE, Laboratory of Neuroimaging of Aging,
University Hospitals and University of Geneva, Geneva,
Switzerland, Geneva, Switzerland, 6Department
of Psychiatry and Clinical Psychobiology, Universitat de
Barcelona and IDIBAPS, Barcelona, Spain, Barcelona, Spain, 7Department
of Neuroradiology and Magnetic Resonance Image core
Facility, Hospital Clínic de Barcelona, IDIBAPS, Barcelona,
Spain, Barcelona, Spain, 8Alzheimer's
Disease and Other Cognitive Disorders Unit, Department of
Neurology, Hospital Clínic, and IDIBAPS, Barcelona, Spain,
Barcelona, Spain, 9Deptartment
Geriatrics, Neuroscience & Orthopaedics, Catholic
University, Policlinic Gemelli, Rome, Italy, Rome, Italy, 10IRCSS
S.Raffaele Pisana, Rome, Italy, Rome, Italy,11Center
for Neuropsychological Research, Catholic University, Rome,
Italy, Rome, Italy, 12LVR-Clinic
for Psychiatry and Psychotherapy, Institutes and Clinics of
the University Duisburg-Essen, Essen, Germany, Essen,
Germany, 13Department
of Psychiatry and Psychotherapy, University Medical Center
(UMG), Georg August University, Göttingen, Germany,
Göttingen, Germany, 14Department
of Neuroradiology, IRCSS San Martino University Hospital and
IST, Genoa, Italy, Genoa, Italy, 15Department
of Health Sciences, University of Genoa, Genoa, Italy,
Genoa, Italy, 16Department
of Neuroscience, Ophthalmology, Genetics and Mother–Child
Health (DINOGMI), University of Genoa, Genoa, Italy, Genoa,
Italy, 17Pharmacology,
Assistance Publique — Hôpitaux de Marseille, Aix-Marseille
University — CNRS, UMR 7289, Marseille, France, Marseille,
France, 18CRMBM–CEMEREM,
UMR 7339, Aix Marseille Université — CNRS, Marseille,
France, Marseille, France, 19APHM,
CHU Timone, Service de Neurologie et Neuropsychologie,
Marseille, France, Marseille, France, 20Aix
Marseille Université, Inserm, INS UMR_S 1106, 13005,
Marseille, France, Marseille, Italy, 21Université
de Lille, Inserm, CHU Lille, U1171 - Degenerative and
vascular cognitive disorders, F-59000 Lille, France, Lille,
France, 22INSERM,
Imagerie cérébrale et handicaps neurologiques, UMR 825,
Toulouse, France, Toulouse, France, 23Université
de Toulouse, UPS, Imagerie cérébrale et handicaps
neurologiques, UMR 825, CHU Purpan, Place du Dr Baylac,
Toulouse Cedex 9, France, Toulouse, France, 24Department
of Neuroradiology, General Hospital, Verona, Italy, Verona,
Italy, 25Department
of Neuroscience Imaging and Clinical Sciences, University
“G. d'Annunzio” of Chieti, Italy, Chieti, Italy, 26Institute
for Advanced Biomedical Technologies (ITAB), University “G.
d'Annunzio” of Chieti, Italy, Chieti, Italy,27IRCCS
SDN, Naples, Italy, Naples, Italy, 28University
of Naples Parthenope, Naples, Italy, Naples, Italy, 29Section
of Neurology, Centre for Memory Disturbances, University of
Perugia, Perugia, Italy, Perugia, Italy,30Medical
Physics Unit, Perugia General Hospital, Perugia, Italy,
Perugia, Italy, 31Neuroradiology
Unit, Perugia General Hospital, Perugia, Italy, Perugia,
Italy, 323rd
Department of Neurology, Aristotle University of
Thessaloniki, Thessaloniki, Greece, Thessaloniki, Greece, 33Interbalkan
Medical Center of Thessaloniki, Thessaloniki, Greece,
Thessaloniki, Greece, 34Interbalkan
Medical Center of Thessaloniki, Thessaloniki, Greece
Department of Radiology, Aristotle University of
Thessaloniki, Thessaloniki, Greece, Thessaloniki, Greece
Brain diffusion tensor imaging (DTI) provides in-vivo
characterization of white matter tissue microstructure. In
this study we demonstrate that free water elimination in
brain diffusion MRI significantly improves the test-retest
reproducibility of DTI metrics (fractional anisotropy,
axial, radial and mean diffusivity) in a multsite 3T
setting. This work has important clinical applications since
the improved reliability may provide increased sensitivity
in longitudinal studies quantifying white matter
neurophysiological processes related to disease
stage/progression and treatment responses.
|
|
16:48
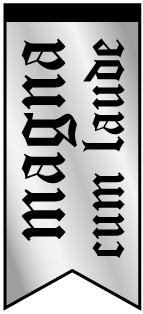 |
1044.
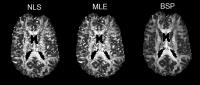 |
Robust DKI parameter estimation in case of CSF partial volume
effects 
Quinten Collier1, Arnold Jan den Dekker1,2,
Ben Jeurissen1, and Jan Sijbers1
1iMinds Vision Lab, University of Antwerp,
Antwerp, Belgium, 2Delft
Center for Systems and Control, Delft University of
Technology, Delft, Netherlands
Diffusion kurtosis imaging (DKI) suffers from partial volume
effects caused by cerebrospinal fluid (CSF). We propose a
DKI+CSF model combined with a framework to robustly estimate
the DKI parameters. Since the estimation problem is
ill-conditioned, a Bayesian estimation approach with a
shrinkage prior is incorporated. Both simulation and real
data experiments suggest that the use of this prior leads to
a more accurate, precise and robust estimation of the
DKI+CSF model parameters. Finally, we show that not
correcting for the CSF compartment can lead to severe biases
in the parameter estimations.
|
|
17:00
|
1045.
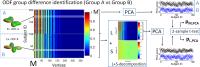 |
Low Rank plus Sparse Decomposition of ODF Distributions for
Improved Detection of Group Differences in Diffusion Spectrum
Imaging 
Steven H. Baete1,2, Jingyun Chen1,2,3,
Ricardo Otazo1,2, and Fernando E. Boada1,2
1Center for Advanced Imaging Innovation and
Research (CAI2R), NYU School of Medicine, New York, NY,
United States, 2Center
for Biomedical Imaging, Dept of Radiology, NYU School of
Medicine, New York, NY, United States, 3Steven
and Alexandra Cohen Veterans Center for Posttraumatic Stress
and Traumatic Brain Injury, Dept of Psychiatry, NYU School
of Medicine, New York, NY, United States
Recent advances in data acquisition make it possible to use
Diffusion Spectrum Imaging (DSI) as a clinical tool for in
vivo study of white matter architecture. The dimensionality
of DSI data sets requires a more robust methodology for
their statistical analyses than currently available. Here we
propose a combination of Low-Rank plus Sparse (L+S) matrix
decomposition and Principal Component Analysis to reliably
detect voxelwise group differences in the Orientation
Distribution Function that are robust against the effects of
noise and outliers. We demonstrate the performance of this
approach using simulations to assess group differences
between known ODF distributions.
|
|
17:12
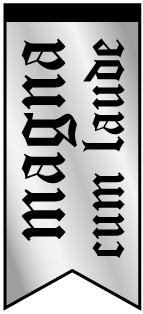 |
1046.
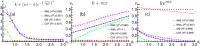 |
Investigating the effects of intrinsic diffusivity on neurite
orientation dispersion and density imaging (NODDI) 
Jose M Guerrero1, Nagesh Adluru2,
Steven R Kecskemeti2, Richard J Davidson3,
and Andrew L Alexander1
1Medical Physics, University of Wisconsin -
Madison, Madison, WI, United States, 2Waisman
Center, University of Wisconsin - Madison, Madison, WI,
United States, 3Psychology
and Psychiatry, University of Wisconsin - Madison, Madison,
WI, United States
NODDI model and its widely used estimation toolbox assume
the intracellular (or intrinsic) diffusivity (ID) to a fixed
value suitable for healthy adult brains. For broader
applicability of the model in neurological diseases it is
important to understand the effects of ID. Using multi-shell
diffusion data we investigated the variability of estimated
NODDI indices as well as the model residuals with respect to
variations in ID. Our results suggest that the value for ID
cannot simply be set to that offering the least residual
since there are appreciable effects on the indices even in a
small range of ID values.
|
|
17:24
|
1047.
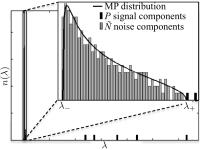 |
Denoising of diffusion MRI data using Random Matrix Theory 
Jelle Veraart1,2, Dmitry S. Novikov2,
Jan Sijbers1, and Els Fieremans2
1iMinds Vision Lab, University of Antwerp,
Antwerp, Belgium, 2Center
for Biomedical Imaging, New York University School of
Medicine, New York, NY, United States
We here adopt the idea of noise removal by means of
transforming redundant data into the Principal Component
Analysis (PCA) domain and preserving only the components
that contribute to the signal to denoise diffusion MRI
(dMRI) data. We objectify the threshold on the PCA
eigenvalues for denoising by exploiting the fact that the
noise-only eigenvalues are expected to obey the universal
Marchenko-Pastur (MP) distribution. By doing so, we design a
selective denoising technique that reduces signal
fluctuations solely rooting in thermal noise, not in fine
anatomical details.
|
|
17:36
|
1048.
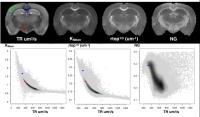 |
A systematic comparative study of DTI and higher order diffusion
models in brain fixed tissue 
Elizabeth B Hutchinson1, Alexandru Avram1,
Michal Komlosh1, M Okan Irfanoglu1,
Alan Barnett1, Evren Ozarslan2, Susan
Schwerin3, Kryslaine Radomski3, Sharon
Juliano3, and Carlo Pierpaoli1
1SQITS, NICHD/NIH, Bethesda, MD, United States, 2Bogazici
University, Istanbul, Turkey, 3APG,
USUHS, Bethesda, MD, United States
We have systematically compared four diffusion MRI models –
DTI, DKI, MAP-MRI and NODDI – in the same DWI data sets for
fixed brain tissue to identify the relative strengths of
these approaches and characterize the effects of
experimental design and image quality on the generated
metrics. Metric-specific advantages in sensitivity and
specificity were shown as well as differential vulnerability
across the metrics to DWI sampling scheme and noise. The
intention of this work is to provide an integrative view of
diffusion metrics that contributes to their utility in brain
research.
|
|
17:48
|
1049.
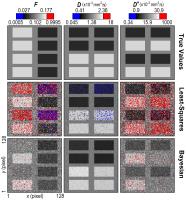 |
A caveat to Bayesian estimation in intravoxel incoherent motion
modelling 
Peter T. While1, Igor Vidic2, and Pål
E. Goa2
1Department of Radiology and Nuclear Medicine,
St. Olav's University Hospital, Trondheim, Norway, 2Department
of Physics, Norwegian University of Science and Technology
(NTNU), Trondheim, Norway
Intravoxel incoherent motion (IVIM) modelling has the
potential to provide pixel-wise maps of pseudo-diffusion
parameters that offer insight into tissue microvasculature.
However, standard approaches using least-squares fitting
yield parameter maps that are typically heavily corrupted by
noise. Bayesian modelling has been shown recently to be a
promising alternative. In this work we test the robustness
of one such Bayesian approach by applying it to simulated
noisy data, and obtain clearer parameter maps with much
lower estimation uncertainty than least-squares fitting.
However, certain features are found to disappear completely,
indicating that a level of caution is required when
implementing such techniques.
|
|