Wednesday, 26 April 2017
Room 316BC |
16:15 - 18:15 |
Moderators: James Pekar, Stephen Smith |
Slack Channel: #s_fmri
Session Number: O77
16:15
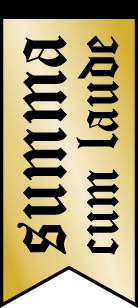 |
0952.
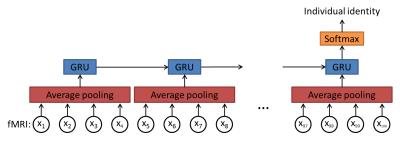 |
Individual identification using brain functional fingerprint detected by recurrent neural network 
Shiyang Chen, Xiaoping Hu
We introduce a deep learning approach to derive functional fingerprint of the brain that can identify individuals. By investigating the features extracted by our model, we noticed that they mostly resemble the existing resting state networks, and three networks (default mode, attention, and frontopariental control networks) contribute the most to individual discriminability.
|
16:27
|
0953.
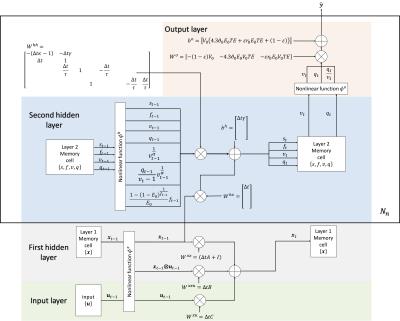 |
Generalized Recurrent Neural Network accommodating Dynamic Causal Modelling for functional MRI analysis 
Yuan Wang, Yao Wang, Yvonne Lui
We propose DCM-RNN, a new model for effective connectivity estimation from fMRI signal that links the strengths of traditional Dynamic Casual Modelling (DCM) and deep learning. It casts DCM as a generalized Recurrent Neural Network (RNN) and estimates the effective connectivity using backpropagation. It extends DCM with a more flexible framework, unique estimation methods, and neural network compatibility. In simulated experiments, we demonstrate that DCM-RNN is feasible and can be used to estimate the effective connectivity.
|
16:39
|
0954.
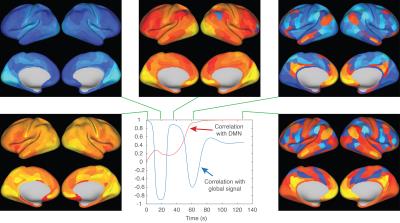 |
A simple data driven predictive dynamical model of whole brain resting state fMRI signal behavior 
Eric Wong
In this work we present a simple dynamical model that learns from resting state fMRI data to predict future brain states using information from one or more past brain states. We use a fully connected neural network model, and data from the Human Connectome Project. Our group model explains over 45% of the variance across 472 subjects, is very consistent when trained on subsets of the subjects, predicts realistic dynamics, gravitates towards the default mode when started in nearly any simulated brain state, and is complementary to correlation analysis.
|
16:51
|
0955.
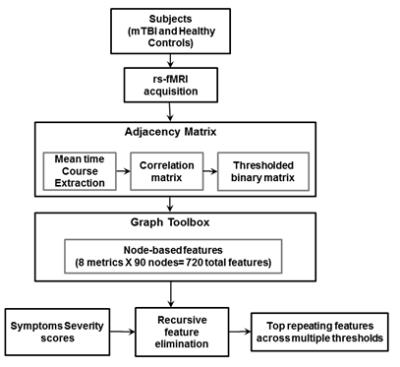 |
Selection of node-based graph metrics to predict symptom severity in mild traumatic brain injury (mTBI) using recursive feature elimination - permission withheld
Bharath Sundar, Hariharan Ravishankar, Suresh Joel, Luca Marinelli, Teena Shetty, Pratik Mukherjee, Joseph Masdeu, Rakesh Mullick, Radhika Madhavan
Recursive feature elimination (RFE), a machine learning technique, is used to sub-select node-based graph theoretical features that correlate with symptom severity in mTBI. Resting state functional connectivity was represented as a binary graph by thresholding correlation values computed between time courses of functional ROIs. Node-based graph theoretical metrics were computed and fed to the feature elimination model to regress on mTBI symptom scores. Using RFE we identified top features correlated to symptom severity in mTBI, which include eigen centrality and closeness of nodes within the salience and default-mode networks. Top features were analyzed for repeatability over multiple runs and multiple thresholds.
|
17:03
|
0956.
 |
Dynamic parcellation of cortical functional networks in developing preterm brains 
Qinmu Peng, Risheng Liu, Minhui Ouyang, Chenying Zhao, Xin Fan, Bo Hong, Hao Huang
Connectivity is a major organizing principle of the central nervous system. However, little is known on how the cortical functional connectivity emerges in the network organization in the preterm brain. Here, we aimed to demonstrate emergence of primary functional regions using a parcellation method based on measurements from resting-state fMRI. Our results showed the clear emergence of primary sensorimotor and visual regions at 32, 36 and 40 postmenstrual weeks, while coherent parcellations of higher-order network regions are not apparent. The study demonstrated that dynamic parcellation based on resting-state fMRI can effectively delineate differentiated emergence of cortical networks during preterm development.
|
17:15
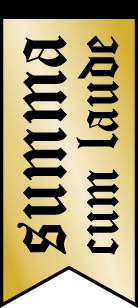 |
0957.
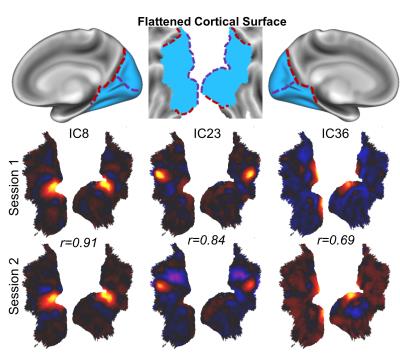 |
Spontaneous Activity Patterns Reveal Non-Retinotopic Functional Parcellation and Organization of Human Visual Cortex 
Kun-Han Lu, Jun Young Jeong, Haiguang Wen, Zhongming Liu
We applied Independent Component Analysis (ICA) for mapping cortical visual areas and networks based on resting-state fMRI signals within the visual cortex. We found that spontaneous brain activity at rest exhibits reliable network patterns not only in the whole-brain scale but also in much finer scales. These networks show notable differences compared to the classical visual regions defined with retinotopic mapping or cytoarchitecture. In addition, these networks are anatomically segregated and functionally specialized into three modules: the dorsal pathway, the ventral pathway, and the early visual areas shared by these two pathways.
|
17:27
|
0958.
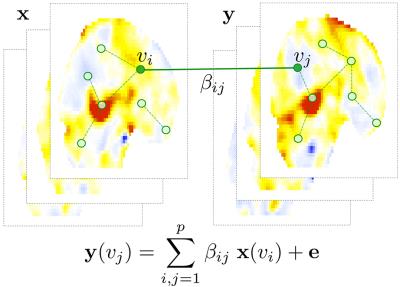 |
Hyper Network Analysis on Paired Images 
Moo Chung, Victoria Vilalta-Gil, Paul Rathouz, Benjamin Lahey, David Zald
We present a new integrative framework for analyzing paired images using hyper-networks. The method is applied to twin fMRI study in characterizing the amount of heritability in the functional network.
|
17:39
|
0959.
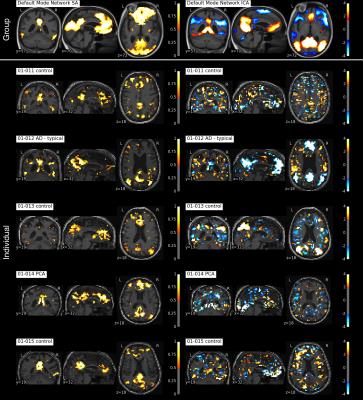 |
Sparse Network Analysis of Individual Resting-State BOLD-fMRI 
Michael Hütel, Andrew Melbourne, David Thomas, Jonathan Rohrer, Sebastien Ourselin
Functional Magnetic Resonance Imaging (fMRI) is a key neuroimaging technique in large cohort studies, allowing the analysis of healthy and pathological networks of spontaneous brain function. However, resting stage fMRI analysis is often limited by the requirement for image registration and the resulting spatial smoothing used to ensure spatial consistency between subjects. We propose an analysis strategy to overcome these limitations using a novel non-linear Sparse Autoencoder to produce functional network decompositions in each subject without the need for spatial smoothing nor registration. This technique applied at both the group and individual level retains the capability to obtain unique single subject functional network representations. We use this technique to reveal consistent individual-level network differences between a group of healthy controls and individuals diagnosed with young-onset dementia; most strikingly in areas representing a working-memory network.
|
17:51
|
0960.
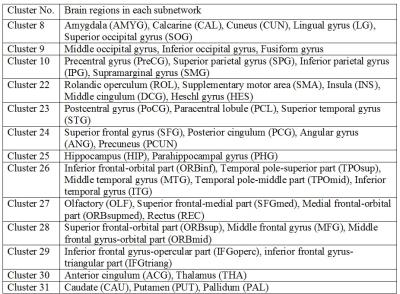 |
A novel method for extracting hierarchical subnetworks based on a multi-subject spectral clustering approach 
Xiaoyun Liang, Chun-Hung Yeh, Alan Connelly, Fernando Calamante
Brain-network has an intrinsic hierarchical structure, which, however, cannot be uncovered using the current methods exclusively for modularity analysis. A recent study has investigated hierarchical structure of brain-network using a hierarchical-clustering approach, which, nevertheless, has the following issues: (i) it relies on applying somewhat arbitrary thresholds to cross-coefficients (different thresholds likely yield distinct clustering outcomes); (ii) individual-level clustering results at early steps are likely to introduce biases at later stages, compromising the final clustering. We propose a method, Network Hierarchical Clustering (NetHiClus), based on a multi-subject spectral-clustering approach, which can robustly identify functional sub-networks at hierarchical-level, without thresholding the cross-coefficients.
|
18:03
|
0961.
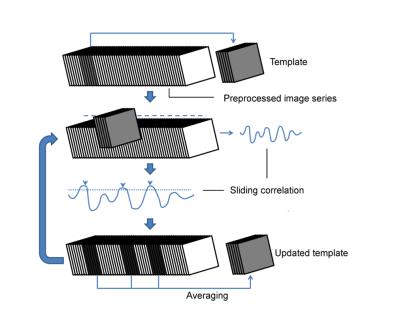 |
Dynamic resting state fMRI in mice: detection of Quasi-Periodic Patterns 
Michaël Belloy, Maarten Naeyaert, Georgios Keliris, Anzar Abbas, Shella Keilholz, Annemie Van Der Linden, Marleen Verhoye
We report, to our knowledge, the first application of a dynamic rsfMRI approach in mice and show the reliable detection of Quasi-Periodic Patterns (QPP) at both the group-level as well as in single-subject data. These patterns are consistent with QPPs detected previously in humans and rats, displaying a high intensity wave spreading across the cortex from lateral towards upward medial, followed by a low intensity wave in the same direction. These findings are promising for the application of QPPs towards investigating mouse resting state functional connectivity and its development as a potential new pre-clinical tool.
|
|