Tuesday, 25 April 2017
Room 313BC |
08:15 - 10:15 |
Moderators: Tim Leiner, Reza Nezafat |
Slack Channel: #s_latebreaking
Session Number: O89
08:15
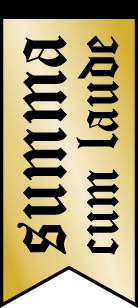 |
5657.
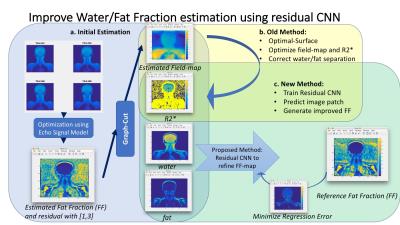 |
Improved Multi-echo Water-fat Separation Using Deep Learning 
Enhao Gong, Greg Zaharchuk, John Pauly
Multi-echo water/fat separation may fail on cases due to noise, inaccurate estimation of water/fat signal and inhomogeneous $$$B_0$$$ field. Here we developed novel data-driven method to improve water/fat separation using Deep Learning. A Residual-Convolutional-Neural-Network model was trained on image patches of multi-contrast information (from initial estimation of water/fat signal, R2* map and field map), to generate better estimation of Fat-Fraction (FF) image patches and entire FF image. The proposed approach was validated and demonstrated improvement from existing methods on ISMRM datasets with variable anatomies. This method can handle flexible echo times in acquisition and is efficient and effective.
|
08:27
|
5658.
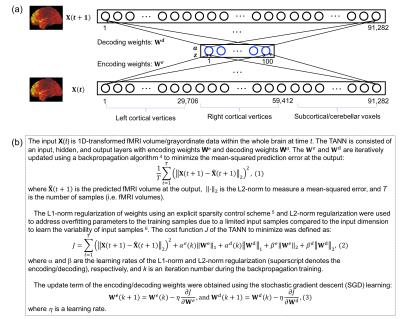 |
Temporal-autoencoding neural network revealed the underlying functional dynamics of fMRI data: Evaluation using the Human Connectome Project data 
Jong-Hwan Lee, Eric Wong, Peter Bandettini
We proposed a novel approach based on a temporal autoencoding neural network (TANN) model to predict the fMRI volume in the next time point or repetition time (TR) based on the fMRI volume in the present TR. Using motor task data from the Human Connectome Project, our TANN model revealed the human motor cortex dynamics. The highly task-specific foot, hand, and tongue networks within the motor-related areas were clearly identified from the TANN weight features and the task-associated networks across the frontal, parietal, temporal, and visual areas were also clearly parcellated without any task information.
|
08:39
|
5659.
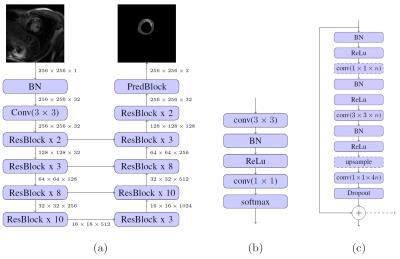 |
Fully Automated Left Ventricle Scar Quantification with Deep Learning - permission withheld
Johannes Rausch, Bjoern Menze, Raymond Chan, Jihye Jang, Evan Appelbaum, Reza Nezafat
We present a fully automated approach for quantification of left ventricle scar in Late Gadolinium Enhancement (LGE) cardiac MR (CMR), using a residual neural network. LGE images were acquired in 1075 patients with known hypertrophic cardiomyopathy in a multi-center clinical trial. Scar segmentation was performed in all patients by a CMR-trained cardiologist. For training, we use a two-phase procedure, using cropped and full-sized images consecutively. We train different models using sigmoid cross-entropy loss and Dice loss and measure average LV segmentation Dice scores of 0.77 ± 0.10 and 0.70 ± 0.12 and estimated scar percentage mismatches of 3.59% and 3.00%, respectively.
|
08:51
|
5660.
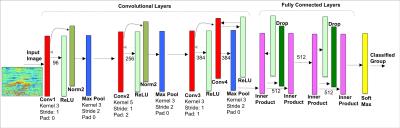 |
Predicting Osteoarthritis Radiographic Incidence by Coupling Quantitative Compositional MRI and Deep Learning - permission withheld
Valentina Pedoia, Jan Neumann , Ursula Heilmeier , Jenny Haefeli, Adam Ferguson, Thomas Link, Sharmila Majumdar
In this study quantitative compositional MRI and deep learning were coupled to discover latent feature representations, non-linear aggregation among elementary features able to characterize relaxation maps for Osteoarthritis diagnosis and progression prediction. 1,348 subjects from the Osteoarthritis Initiative (OAI) public dataset were considered. T2 relaxation map were automatically analyzed to build a 2D feature map used to train a convolutional neural network for the classification of subjects in OA, control and progression groups. The proposed method was able to detect OA subjects with 95.2% accuracy, and to detect controls subjects that demonstrated OA signs 4 years later with 80.7% accuracy.
|
09:03
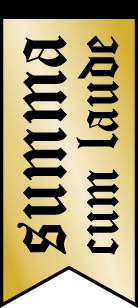 |
5661.
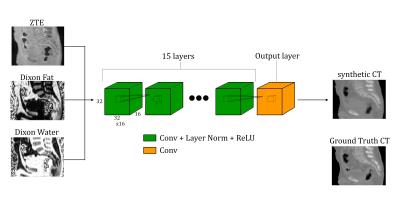 |
Direct Pseudo-CT Image Synthesis Using Deep Learning for Pelvis PET/MR Attenuation Correction 
Andrew Leynes, Jaewon Yang, Dattesh Shanbhag, Sandeep Kaushik, Florian Wiesinger, Youngho Seo, Thomas Hope, Peder Larson
A deep learning model with fully-convolutional networks was used to directly synthesize pseudo-CT images from MR images. The pseudo-CT images were used for MR-based attenuation correction (MRAC) of PET reconstruction in PET/MRI. The effects of the MRAC on high-uptake volumes are evaluated quantitatively. We demonstrate that the deep learning-based MRAC significantly improves PET uptake quantification.
|
09:15
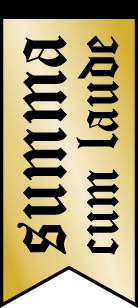 |
5662.
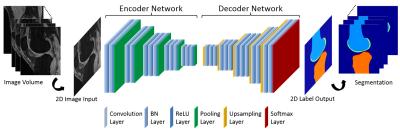 |
Deep Convolutional Auto-Encoder and 3D Deformable Approach for Tissue Segmentation in Magnetic Resonance Imaging 
Fang Liu, Zhaoye Zhou, Hyungseok Jang, Alan McMillan, Richard Kijowski
A fully-automated segmentation pipeline was built by combining a deep Convolutional Auto-Encoder (CAE) network and 3D simplex deformable modeling. The CAE was applied as the core of the segmentation method to perform high resolution pixel-wise multi-class tissue classification. The 3D simplex deformable modeling refined output from CAE to preserve the overall shape and maintain a desirable smooth surface for structure. The fully-automated segmentation method was tested using a publicly available knee joint image dataset to compare with currently used state-of-the-art segmentation methods. The fully-automated method was also evaluated on morphological MR images with different tissue contrasts and image training datasets.
|
09:27
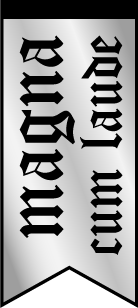 |
5663.
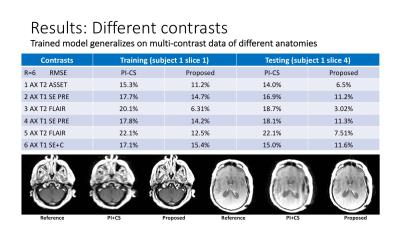 |
Improving the PI+CS Reconstruction for Highly Undersampled Multi-contrast MRI using Local Deep Network 
Enhao Gong, Greg Zaharchuk, John Pauly
A typical clinical MR protocol includes multiple scans with different contrasts for complementary diagnostic information. Various methods have been proposed to specifically accelerate multi-contrast scans by using more complicated sparsity regularization in PI+CS. Here we proposed a Non-iterative Deep-Learning approach to further improve existing methods for highly undersampled multi-contrast MRI reconstruction.
This method uses a Sequential+Joint+Local scheme, which takes fast PI+CS reconstruction as the initial input, uses a Deep-Network on local patches, and efficiently generates a better reconstruction for different contrasts with reduced noise and artifacts.
Experiments demonstrate the proposed method has superior performance compared with existing PI+CS methods.
|
09:39
|
5664.
 |
An Artificial Neural Network Framework for Early Prediction of Cognitive Deficits in Very Preterm Infants 
Lili He, Hailong Li, Weihong Yuan, Nehal Parikh
Annually, approximately 22,000 very preterm infants (i.e. ≤32 weeks gestational age) in the United States develop cognitive deficits. Infant brains are highly malleable, making it especially important to identify those at highest risk as early as possible to allow effective early interventions. Research supports the notion that cognitive deficits may result from a disturbance/breakdown in the connectome. We propose to develop a robust artificial neural network framework that can analyze integrated structural and functional brain connectome data obtained at term corrected age to predict long-term cognitive outcomes in very preterm infants.
|
09:51
|
5665.
 |
Deep learning: Utilizing the potential in data bases to predict individual outcome in acute stroke 
Anne Nielsen, Kim Mouridsen, Mikkel Hansen, Jens Boldsen
Acute ischemic stroke is a major disease and one of the leading causes of adult death and disability. Brain tissue infarcts permanently within hours after onset and rapid reperfusion treatment is therefore of utmost importance. Current methods to predict the tissue outcome are too simplistic. In this project a more advanced approach using deep neural networks to utilize the information from previous patient developments was established and compared to current state-of-the-art. The predictions from the deep neural networks were showed to be superior to the state-of-the-art method improving prediction accuracy and hence leading to better decision support.
|
10:03
|
5666.
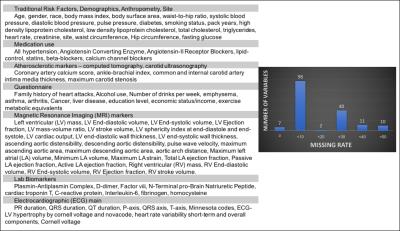 |
Cardiovascular Event Prediction by Machine Learning: The Multi-Ethnic Study of Atherosclerosis 
Bharath Ambale Venkatesh, Xiaoying Yang, Colin Wu, W. Gregory Hundley, Antoinette Gomes, Eliseo Guallar, David Bluemke, Joao Lima
Event prediction has been the cornerstone of cardiovascular epidemiology and have allowed us to characterize sub-clinical disease processes and target key risk factors for modification. Epidemiological studies used to derive such predictive models frequently contain hundreds of variables from multiple tests. Random survival forests may be an effective machine learning strategy for incident event prediction in large populations with large phenotypic datasets. These methods do not require a priori assumptions regarding causality and may thus be suitable to defining the role of novel biomarkers and tests (such as imaging, biomarker panels, ECG, etc) for cardiovascular disease prediction. We explore the role of MRI in the prediction of incident heart failure and all-cause death.
|
|