Deep Learning for Image Reconstruction
Oral
Acquisition, Reconstruction & Analysis
Tuesday, 19 June 2018
N01 |
16:15 - 18:15 |
Moderators: Shanshan Wang, Florian Knoll |
16:15
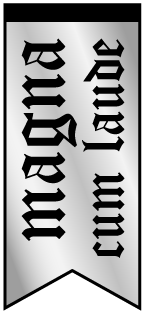 |
0568.
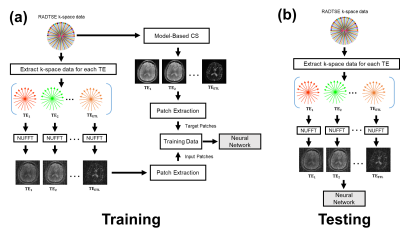 |
A Multi-scale Deep ResNet for Radial MR Parameter Mapping
Zhiyang Fu, Sagar Mandava, Mahesh Keerthivasan, Diego Martin, Maria Altbach, Ali Bilgin
Quantitative mapping of MR parameters has shown great potential for tissue characterization but long acquisition times required by conventional techniques limit their widespread adoption in the clinic. Recently, model-based compressive sensing (CS) reconstructions that produce accurate parameter maps from a limited amount of data have been proposed but these techniques require long reconstruction times making them impractical for routine clinical use. In this work, we propose a multi-scale deep ResNet for MR parameter mapping. Experimental results illustrate that the proposed method achieves reconstruction quality comparable to model-based CS approaches with orders of magnitude faster reconstruction times.
|
16:27
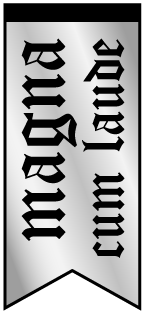 |
0569.
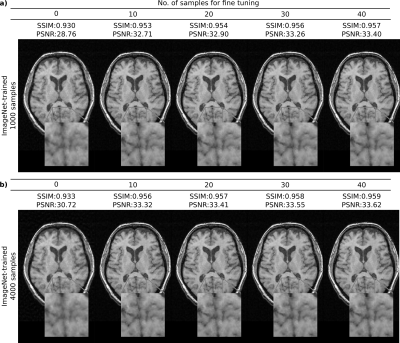 |
Transfer learning for reconstruction of accelerated MRI acquisitions via neural networks
Salman Ul Hassan Dar, Tolga Çukur
Neural network architectures have recently been proposed for reconstruction of undersampled MR acquisitions. These networks contain a large number of free parameters that typically have to be trained on orders-of-magnitude larger sets of fully-sampled MRI data. In practice, however, large datasets comprising thousands of images are rare. Here, we propose a transfer-learning approach to address the problem of data scarcity in training deep networks for accelerated MRI. Results show that networks obtained via transfer-learning using only tens of images in the testing domain achieve nearly identical performance to networks trained directly in the testing domain using thousands of MR images.
|
16:39
|
0570.
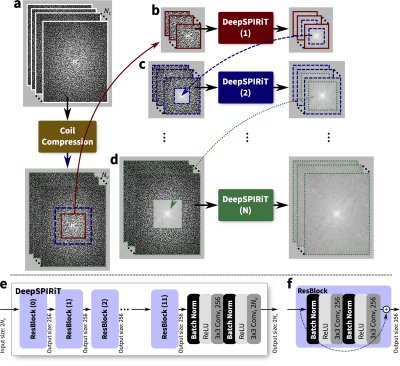 |
DeepSPIRiT: Generalized Parallel Imaging using Deep Convolutional Neural Networks
Joseph Cheng, Morteza Mardani, Marcus Alley, John Pauly, Shreyas Vasanawala
A parallel-imaging algorithm is proposed based on deep convolutional neural networks. This approach eliminates the need to collect calibration data and the need to estimate sensitivity maps or k-space interpolation kernels. The proposed network is applied entirely in the k-space domain to exploit known properties. Coil compression is introduced to generalize the method to different hardware configurations. Separate networks are trained for different k-space regions to account for the highly non-uniform energy. The network was trained and tested on both knee and abdomen volumetric Cartesian datasets. Results were comparable to L2-ESPIRiT and L1-ESPIRiT which required calibration data from the ground truth.
|
16:51
|
0571.
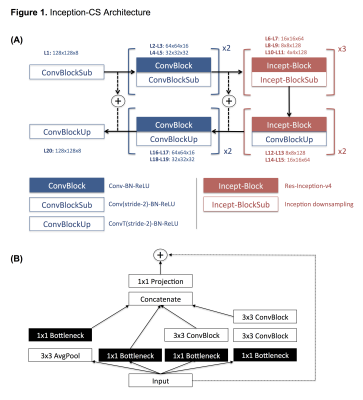 |
Inception-CS: Deep Learning For Sparse MR Reconstruction in Glioma Patients
Peter Chang, Michael Liu, Daniel Chow, Melissa Khy, Christopher Filippi, Janine Lupo, Christopher Hess
Sparse MR image reconstruction through deep learning represents a promising novel solution with early results suggesting improved performance compared to standard techniques. However, given that neural networks reconstruct using a learned manifold of rich image priors, it is unclear how the algorithm will perform when exposed to pathology not present during network training. In this study we: (1) present a novel Inception-CS architecture for reconstruction using extensive residual Inception-v4 modules; (2) demonstrate state-of-the-art reconstruction performance in glioma patients however only when representative pathology is available during algorithm training.
|
17:03
|
0572.
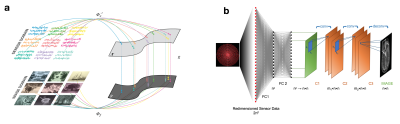 |
Deep learning MR reconstruction with Automated Transform by Manifold Approximation (AUTOMAP) in real-world acquisitions with imperfect training
Bo Zhu, Berkin Bilgic, Congyu Liao, Bruce Rosen, Matthew Rosen
Automated Transform by Manifold Approximation (AUTOMAP) is a generalized MR image reconstruction framework based on supervised manifold learning and universal function approximation implemented with a deep neural network architecture. Here we investigate the effect of significant sampling trajectory error in spiral acquisitions, where mismatch between training and runtime scanner trajectories may result in unpredictable reconstruction artifacts. We demonstrate through Monte Carlo analysis that the error in AUTOMAP reconstruction increases smoothly as a function of trajectory error, demonstrating reasonable robustness to trajectory deviation. We find these simulation results are consistent with reconstruction performance on real scanner data acquired from human subjects.
|
17:15
|
0573.
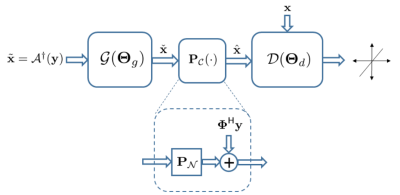 |
Deep Generative Adversarial Neural Networks for Compressed Sensing (GANCS) Automates MRI
Morteza Mardani, Enhao Gong, Joseph Cheng, Shreyas Vasanawala, Greg Zaharchuk, Lei Xing, John Pauly
MRI suffers from aliasing artifacts when undersampled for real-time imaging. Conventional compressed sensing (CS) is not however cognizant of image diagnostic quality, and substantially trade-off accuracy for speed in real-time imaging. To cope with these challenges we put forth a novel CS framework that permeates benefits from generative adversarial networks (GAN) to modeling a manifold of MR images from historical patients. Evaluations on a large abdominal MRI dataset of pediatric patients by expert radiologists corroborate that GANCS retrieves improved images with finer details relative to CS-MRI and deep learning schemes with pixel-wise costs, at 100 times faster speed than CS-MRI.
|
17:27
|
0574.
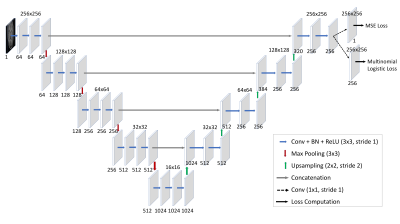 |
ReconNet: A Deep Learning Framework for Transforming Image Reconstruction into Pixel Classification
Kamlesh Pawar, Zhaolin Chen, N Shah, Gary Egan
A deep learning framework is presented that transforms the image reconstruction problem from under-sampled k-space data into pixel classification. The underlying target image is represented by a quantized image, which makes it possible to design a network that classifies each pixel to a quantized level. We have compared two deep learning encoder-decoder networks with the same complexity: one is a classification network and the other is a regression network. Even though the complexity of both the networks is the same, the images reconstructed using the classifier network have resulted in a six times improvement in the mean squared error compared to the regression network.
|
17:39
|
0575.
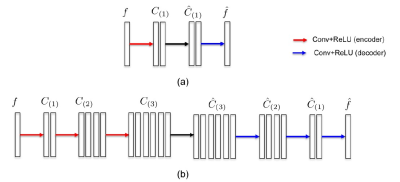 |
Deep convolutional framelet neural network for reference-free EPI ghost correction
Juyoung Lee, Jong Chul Ye
Annihilating filter-based low rank Hankel matrix approach (ALOHA) was recently used as a reference-free ghost artifact correction method. Inspired by another discovery that convolutional neural network can be represented by Hankel matrix decomposition, here we propose a deep CNN for reference-free EPI ghost correction. Using real EPI experiments, we demonstrate that the proposed method effectively removes the ghost artifacts with much faster reconstruction time compared to the existing reference-free approaches.
|
17:51
|
0576.
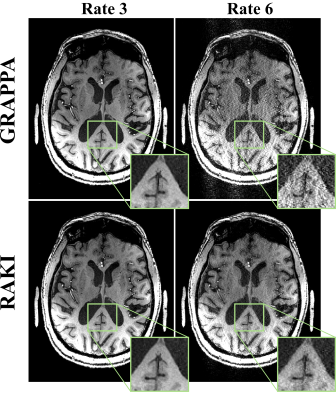 |
Scan-specific Robust Artificial-neural-networks for k-space Interpolation (RAKI): Database-free Deep Learning Reconstruction for Fast Imaging
Mehmet Akçakaya, Steen Moeller, Sebastian Weingärtner, Kâmil Ugurbil
Long scan times remain a limiting factor in MRI. Accelerated imaging is commonly required, with parallel imaging being the most clinically used approach. Recently, machine learning has also been applied to accelerated MRI reconstruction, where the focus has been on training regularizers on large datasets. In this work, we develop a scan-specific deep learning k-space method for reconstruction of undersampled data. The proposed method, Robust Artificial-neural-networks for k-space Interpolation (RAKI) learns a non-linear convolutional neural network from limited autocalibration signal. Phantom, cardiac and brain data show that RAKI improves upon the reconstruction quality of linear k-space interpolation-based parallel imaging methods.
|
18:03
|
0577.
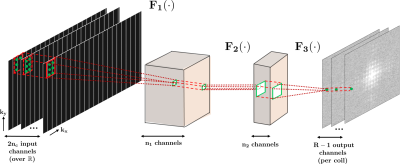 |
Application of a Scan-Specific Deep Learning Reconstruction to Multiband/SMS Imaging
Steen Moeller, Sebastian Weingärtner, Kamil Ugurbil, Mehmet Akcakaya
The use of convolutional neural networks (RAKI (Robust Artifical-neural-networks for k-space Interpolation)) trained for a scan specific acquisition is applied to Multiband/Simultaneous MultiSlice aliased k-space. With CNN’s of similar size as the RO-SENSE-GRAPPA kernels, reduced signal aliasing is obtained.
|
|