Machine Learning for Magnetic Resonance in Medicine
Combined Educational & Scientific Session
ORGANIZERS: Michael Lustig, Demian Wassermann
Wednesday, 20 June 2018
N01 |
13:45 - 15:45 |
Moderators: Leslie Ying, Demian Wassermann |
Skill Level: Basic to Intermediate
Session Number: W-05
Overview
This session will be focused on introductory techniques for machine learning in general. Specifically, it will address topics including regression and classification, and model selection and performance evaluation procedures. It will have two educational speakers: 1) Providing the nuts and bolts of the techniques; and 2) Providing applications in terms of disease classification or prediction from MR images.
Target Audience
ISMRM attendees looking to apply machine learning techniques in their common research pipelines will benefit from this session. The focus on performance evaluation and model selection will enable the attendees to efficiently evaluate the pertinence of machine learning approaches in their common pipelines and give them the tools to select specific techniques.
Educational Objectives
As a result of attending this course, participants should be able to:
-Identify main algorithmic families in machine learning for regression and classification;
-Apply these techniques to their data sets, evaluate their performance and compare performance across techniques; and
-Better interpret current literature using machine learning for MR problems and soundly evaluate such application.
13:45
|
|
Introduction to Machine Learning in MR Imaging
Gael Varoquaux
Machine learning builds predictive models from the data. It is massive used on medical images these days, for a variety of applications ranging from segmentation to diagnosis.I will give an introductory tutorial to machine learning from a statistical point of view. I will introduce the methodology, the concepts behind the central models, the validation framework and a variety of caveats to look for.I will also discuss some applications to drawing conclusions from brain imaging, and use these applications to highlight various technical issues to have in mind when running machine learning models and interpreting their results.
|
14:15
|
|
Machine Learning for Image Analysis
Ben Glocker
|
14:45
|
0773.
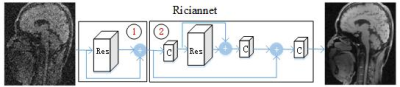 |
Progressively Distribution-based Rician Noise Removal for Magnetic Resonance Imaging
Qiegen Liu, Sanqian Li, Jiujie Lv, Dong Liang
Different from the existing MRI denoising methods that utilizing the spatial neighbor information around the pixels or patches, this work turns to capture the pixel-level distribution information by means of supervised network learning. A wide and progressive network learning strategy is proposed, via fitting the distribution at pixel-level and feature-level with large convolutional filters. The whole network is trained in a two-stage fashion, consisting of the residual network in pixel domain with batch normalization layer and in feature domain without batch normalization layer. Experiments demonstrate its great potential with substantially improved SNR and preserved edges and structures.
|
14:57
|
0774.
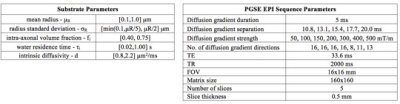 |
Deep neural network based framework for in-vivo axonal permeability estimation
Ioana Hill, Marco Palombo, Mathieu Santin, Francesca Branzoli, Anne-Charlotte Philippe, Demian Wassermann, Marie-Stephane Aigrot, Bruno Stankoff, Hui Zhang, Stephane Lehericy, Alexandra Petiet, Daniel C. Alexander, Olga Ciccarelli, Ivana Drobnjak
This study introduces a novel framework for estimating permeability from diffusion-weighted MRI data using deep learning. Recent work introduced a random forest (RF) regressor model that outperforms approximate mathematical models (Kärger model). Motivated by recent developments in machine learning, we propose a deep neural network (NN) approach to estimate the permeability associated with the water residence time. We show in simulations and in in-vivo mouse brain data that the NN outperforms the RF method. We further show that the performance of either ML method is unaffected by the choice of training data, i.e. raw diffusion signals or signal-derived features yield the same results.
|
15:09
|
0775.
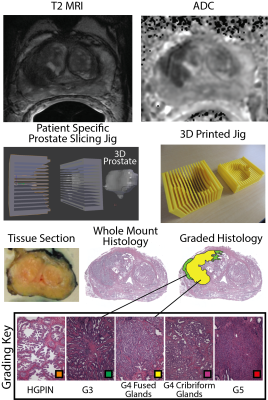 |
Predictive Cytological Topography (PiCT): a Radio-Pathomics Approach to Mapping Prostate Cancer
Sean McGarry, Sarah Hurrell, Kenneth Iczkowski, Amy Kaczmarowski, Anjishnu Banerjee, Tucker Keuter, Kenneth Jacobsohn, William Hall, Marja Nevalainen, Mark Hohenwalter, William See, Andrew Nencka, Peter LaViolette
We present a machine learning technique for mapping prostate cancer cellular features into MRI space. 39 patients were prospectively recruited for imaging prior to prostaectomy. Tissue was aligned with the MRI using a non-linear control point warping technique. Pathologist annotations were likewise transformed into MRI space. A partial least squares regression (PLS) algorithm was trained on two sets of 10 patients and applied to 19 test patients, using MRI values as the input to predict epithelial and lumen density. The output maps are new interpretable image contrasts predictive of prostate cancer presence.
|
15:21
|
0776.
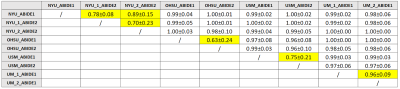 |
Common pitfalls in machine learning applications to multi-center data: tests on the ABIDE I and ABIDE II collections
Elisa Ferrari, Paolo Bosco, Giovanna Spera, Maria Fantacci, Alessandra Retico
Applying Machine Learning (ML) techniques on neuroanatomical MRI data, is becoming widespread for studying psychiatric disorders. However, such instruments require some precautions that, if not applied, may lead to inconsistent results that depend on the procedural choices made in the analysis. In this work, taking neuroimaging studies on Autism Spectrum Disorders as a reference, it is demonstrated that the strong dependency of the cerebral quantities extracted with the segmentation software FreeSurfer 6.0 on the MRI acquisition parameters can, in a multivariate analysis based on ML, obscure the differences due to medical conditions and give inconsistent and meaningless results.
|
15:33
|
0777.
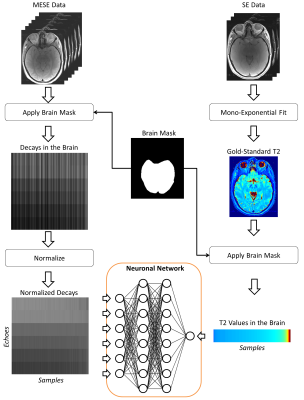 |
Quantitative Mapping by Data-Driven Signal-Model Learning
Tom Hilbert, Jean-Philippe Thiran, Reto Meuli, Tobias Kober
In quantitative MRI, tissue properties are typically estimated by fitting a signal model onto the acquired data. These models are derived from the underlying MR physics describing the signal behavior. The accuracy of the quantitative values heavily depends on the correctness of this model which is usually validated using gold-standard sequences with long acquisition times. Here, we suggest learning the signal model based on the values obtained from the gold-standard sequence with machine learning methods instead. The feasibility of the idea is shown using quantitative T2-mapping with a multi-echo spin-echo sequence and a classical single spin-echo as the gold standard.
|
15:45
|
|
Adjournment & Meet the Teachers |
|