Machine Learning & Post-Processing in MSK
Oral
Musculoskeletal
Thursday, 16 May 2019
Room 511BCEF |
13:45 - 15:45 |
Moderators: Margaret Hall-Craggs, Feliks Kogan |
13:45
|
1135.
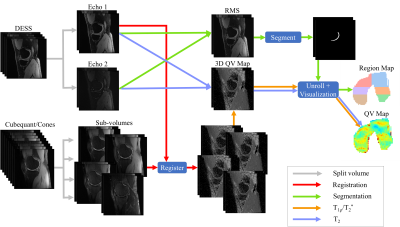 |
DOSMA: A deep-learning, open-source framework for musculoskeletal MRI analysis

Arjun Desai, Marco Barbieri, Valentina Mazzoli, Elka Rubin, Marianne Black, Lauren Watkins, Garry Gold, Brian Hargreaves, Akshay Chaudhari
With the onset of deep learning, many novel post-processing networks are emerging. However, these algorithms are decentralized, and thus are difficult to consolidate and implement in a clinical setting. To this end, we have developed a Python-based deep-learning, open-source musculoskeletal MR analysis framework termed DOSMA to reduce the complexity of tissue segmentation, registration, and quantitative analysis. We hope that by encouraging contributions from many researchers, this pipeline will facilitate automating traditionally laborious, manual, decentralized MR image processing tasks and will provide a standardized comparison framework for different quantitative estimation techniques.
|
13:57
|
1136.
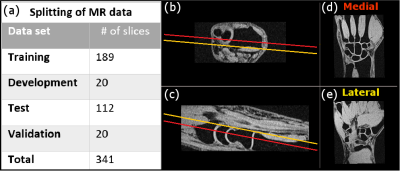 |
Fully automatic segmentation of wrist cartilage on MR images by convolutional neural network

Ekaterina Brui, Aleksandr Efimtcev, Vladimir Fokin, Remi Fernandez, Anatoliy Levchuk, Augustin Ogier, Irina Melchakova, David Bendahan, Anna Andreychenko
A fully automatic, wrist cartilage segmentation method on magnetic resonance images is developed and validated. The method is based on convolutional neural networks (CNN). Cartilage segmentations obtained with the CNN showed a substantial agreement with manual segmentations for the full 3D wrist images and a good agreement for central coronal slices. The proposed method provided cartilage masks having a high concordance with manually obtained ones.
|
14:09
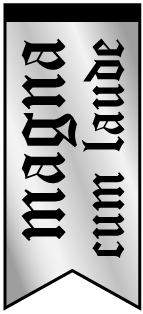 |
1137.
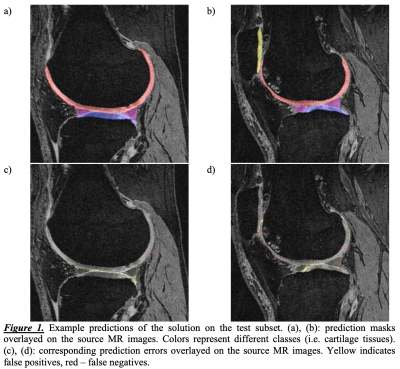 |
Automatic Segmentation of Knee Cartilage and Menisci from MRI Data: Efficient Multiclass Solution Based on Deep Learning

Egor Panfilov, Aleksei Tiulpin, Miika Nieminen, Simo Saarakkala
Manual segmentation of articular cartilage and menisci from magnetic resonance data is time-consuming and can be challenging. Recently, deep learning has shown promising results in medical image segmentation. The aim of this study was to develop a method for automatic segmentation of articular cartilage and menisci that performs more accurately and efficiently than the previously published methods. On OAI/iMorphics dataset the method achieves Dice score of 90.7±1.9 for femoral cartilage, 89.7±2.8 for tibial, 87.1±4.7 for patellar, and 86.3±3.4 for menisci. The presented results could facilitate the osteoarthritis research and enhance clinical practice. Source codes and pretrained models will be open-sourced.
|
14:21
|
1138.
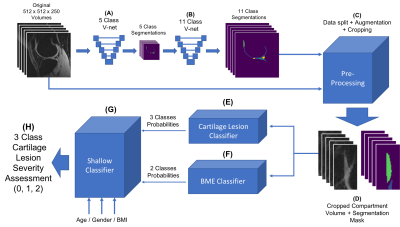 |
MRI-Based Multi-Task Deep Learning for Cartilage Lesion Severity Staging in Knee Osteoarthritis

Bruno Astuto, Io Flament, James Mitrani, Rutwik Shah, Matthew Bucknor, Thomas Link, Valentina Pedoia, Sharmila Majumdar
The automation of the grading task for the knee MRI scoring is appealing. The goal of this study is to leverage recent developments in Deep Learning (DL) applied to medical imaging in order to (i) identify cartilage lesions and assess severity (ii) identify the presence of BMELs, (ii)combine the two models in a multi-task automated and scalable fashion. We were able to boost performance of our final classifiers by not simply focusing on what the fine tuning of a single purpose model could offer, but rather broadly considering related tasks that could bring additional information to our classification problem.
|
14:33
|
1139.
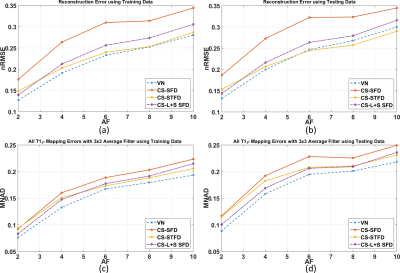 |
Variational Networks for Accelerating Biexponential 3D-T1rho Mapping of Knee Cartilage

Marcelo Zibetti, Azadeh Sharafi, Kerstin Hammernik, Florian Knoll, Ravinder Regatte
Quantitative T1ρ imaging using biexponential models usually requires multiple spin-lock times, which makes the acquisition time demanding. Recently, Compressed Sensing (CS) has demonstrated significant reduction in data acquisition time in MRI applications in general, and T1ρ relaxation mapping of knee cartilage in particular. However, biexponential T1ρ mapping error using CS is much higher than that of monoexponential T1ρ mapping error for the same acceleration factor. One possible approach to reduce artifacts, improving image and mapping quality, is to learn a Variational Network (VN) for image reconstruction. Here, we compare a VN, trained with real knee cartilage images, against the best CS approaches known for biexponential T1ρ mapping. Our results show that the VN produced biexponential maps superior to CS, with lower T1ρ mapping error.
|
14:45
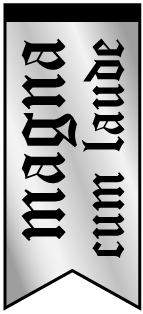 |
1140.
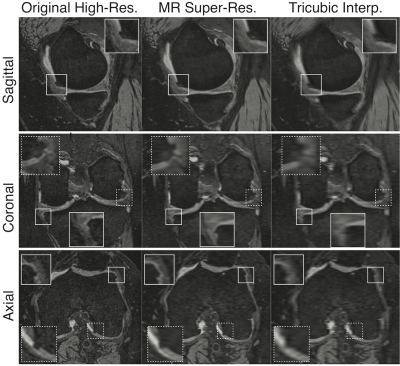 |
Evaluating the Use of Deep-Learning Super-Resolution for Obtaining Osteoarthritis Biomarkers

Akshay Chaudhari, Jeff Wood, Kathryn Stevens, Zhongnan Fang, Jin Lee, Garry Gold, Brian Hargreaves
The use high-resolution magnetic resolution imaging (MRI) is beneficial for acquiring quantitative biomarkers corresponding to osteoarthritis (OA) severity and progression. However, the long scan times of high-resolution sequences, such as double-echo steady-state (DESS) that was included in the Osteoarthritis Initiative, precludes their widespread adoption. Deep-learning-based super-resolution has the potential to transform low-resolution MRI that can be acquired faster, into high-resolution images. Using qualitative cartilage image quality, and quantitative cartilage morphometry and osteophyte detection, we have shown that deep-learning-based super-resolution can enhance DESS slice-resolution threefold and offer the same utility as the original high-resolution acquisition for obtaining OA biomarkers.
|
14:57
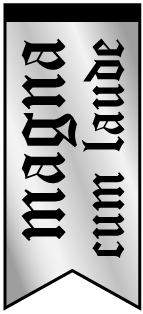 |
1141.
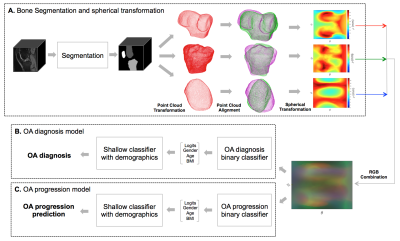 |
Large-scale Knee Osteoarthritis Prediction from Spherical Coordinate Encoding of Bone Shape with Deep Learning Classifier

Alejandro Morales Martinez, Io Flament, Felix Liu, Jinhee Lee , Peng Cao, Sharmila Majumdar, Valentina Pedoia
Osteoarthritis (OA) is a degenerative joint disease which affects 27 million U.S. adults. Previous studies have shown a relationship between bone shape and OA. In this study, the femur, tibia, and patella from the Osteoarthritis (OA) Initiative data set were segmented and converted into a spherical coordinate plane. The 2D spherical images for each bone were combined into RGB images and used to train a knee OA diagnosis and a knee OA progression binary classifier, while taking into account demographic data such as age, gender, and BMI. The model achieved an OA diagnosis average accuracy of 78.15%.
|
15:09
|
1142.
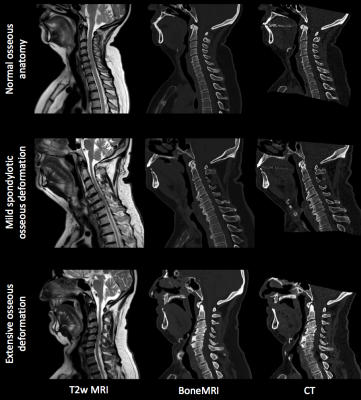 |
BoneMRI of the cervical spine: Deep learning-based radiodensity contrast generation for selective visualization of osseous structures

Marijn van Stralen, Britt van der Kolk, Frank Zijlstra, Mateusz Florkow, Elco Oost, Jorik Slotman, Jochen van Osch, Martin Podlogar, Jeroen Hendrikse, Pim de Jong, Rene Castelein, Max Viergever, Mario Maas, Martijn Boomsma, Peter Seevinck
In this work BoneMRI is presented, a deep learning approach aiming at visualisation of radiodensity contrast by learning a mapping from MRI to CT data from 25 patients. Normal as well as pathological osseous structures in the cervical spine were clearly depicted. Quantitatively, radiodensity contrast similarity and high geometrical accuracy of vertebral dimensions was demonstrated. As BoneMRI is a 3D method it facilitates multiplanar reformatting in any desired direction. As such, BoneMRI is a promising tool for efficient morphological assessment of osseous structures without the need for ionizing radiation, simultaneously providing soft tissue contrasts in a single examination.
|
15:21
|
1143
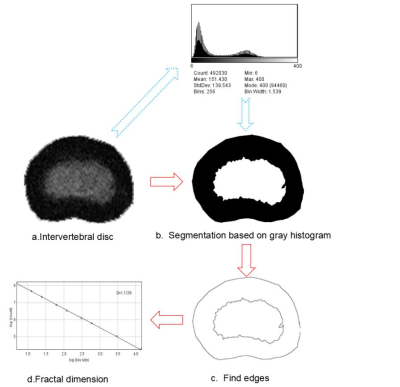 |
Computer-aided classification of intervertebral disc degeneration based on fractal dimension Video Permission Withheld
Ma Junchao, YU Nan, Wang Shaoyu, Wang Ruifeng
Magnetic resonance imaging (MRI) is considered to be the best imaging method to evaluate the IVD degeneration. However, grading systems for degeneration severity evaluation are based on qualitative descriptions of disc image, which impairs the detection of small changes in intervertebral discs. In order to obtain a tool for objective and continuous grading of IVD degeneration. Fractal method was used to analyze the spatial distribution of intervertebral disc signals. We found that fractal dimension value displayed the strongest association with the clinical grading of disc degeneration severity, suggesting that IVD fractal analysis is a suitable tool for objective and continuous IVD degeneration classification.
|
15:33
|
1144.
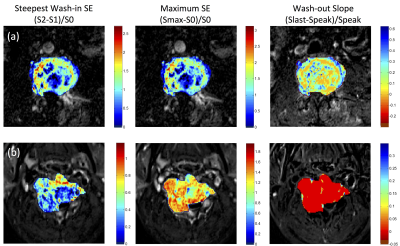 |
Comparison of Radiomics and Deep Learning for Differentiation of Spinal Metastases Coming from Lung Cancer and Other Primary Cancers

Yang Zhang, Ning Lang, Enlong Zhang, Jiahui Zhang, Daniel Chow, Peter Chang, Hon Yu, Huishu Yuan, Min-Ying Su
For patients found to have spinal metastasis, a confirmed pathological diagnosis is needed to proceed with appropriate treatment. This study compared ROI analysis, radiomics, and deep learning for differentiation of primary cancer coming from 30 lung and 31 other tumors. Radiomics using GLCM texture and histogram parameters from the segmented 3D tumor achieved accuracy of 0.71, while the deep learning using recurrent CLSTM network with the entire 12 sets of DCE images reached an accuracy of 0.81. The wash-out slope in DCE kinetics measured from hot-spot was the best diagnostic parameter, which could be easily performed in a clinical setting.
|
|