Deep Learning: Acquisition, Uncertainty & Prediction
Oral
Acquisition, Reconstruction & Analysis
Thursday, 16 May 2019
Room 520A-F |
08:15 - 10:15 |
Moderators: Mariya Doneva, Tao Zhang |
08:15
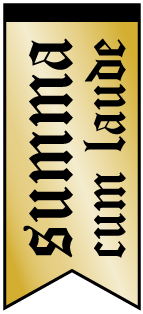 |
1090.
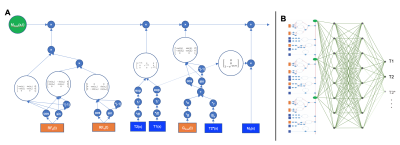 |
AUTOmated pulse SEQuence generation (AUTOSEQ) and neural network decoding for fast quantitative MR parameter measurement using continuous and simultaneous RF transmit and receive

Bo Zhu, Jeremiah Liu, Neha Koonjoo, Bruce Rosen, Matthew Rosen
Limited human intuition of the Bloch equations’ nonlinear dynamics, particularly over long periods of non-steady-state time evolution or in regimes such as off-resonance excitation, is an obstacle to fully exploiting the vast parameter space of potential MR pulse sequences. Our previous work introduced a computational graph approach to modeling the Bloch equations. In this work, we show the AUTOSEQ framework extended with a multilayer fully-connected neural network to perform fast quantitative MR parameter measurement. By employing continuous off-resonant excitation with simultaneous continuous receive, we demonstrate in simulated experiments the ability to quantify T1 and T2 parameters in a single TR.
|
08:27
|
1091.
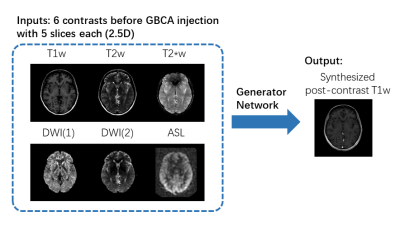 |
Contrast-free MRI Contrast Enhancement with Deep Attention Generative Adversarial Network

Jiang Liu, Enhao Gong, Thomas Christen, Greg Zaharchuk
While gadolinium-based contrast agents (GBCAs) have been dispensable for magnetic resonance imaging in clinic practice, recently there are rising concerns about the safety of GBCAs. In previous studies, we tested the feasibility of predicting contrast agents from pre-contrast images via deep convolutional neural networks. In this study, we further improved the results with deep attention generative adversarial network. The image similarity metrics show that the model can synthesize post-contrast T1w images with superior image quality and great resemblance to the ground truth images. Restoration of contrast uptake is clearly noted on the synthesized images even in small vessels and lesions.
|
08:39
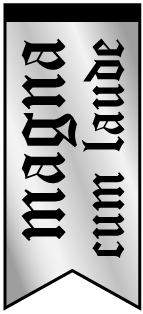 |
1092.
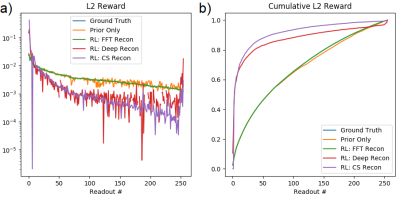 |
Reinforcement Learning for Online Undersampling Pattern Optimization

David Zeng, Christopher Sandino, Dwight Nishimura, Shreyas Vasanawala, Joseph Cheng
Magnetic resonance imaging (MRI) is an important but relatively slow imaging modality. MRI scan time can be reduced by undersampling the data and reconstructing the image using techniques such as compressed sensing or deep learning. However, the optimal undersampling pattern with respect to image quality and image reconstruction technique remains an open question. To approach this problem, our goal is to use reinforcement learning to train an agent to learn an optimal sampling policy. The image reconstruction technique is the environment and the reward is based upon an image metric.
|
08:51
|
1093.
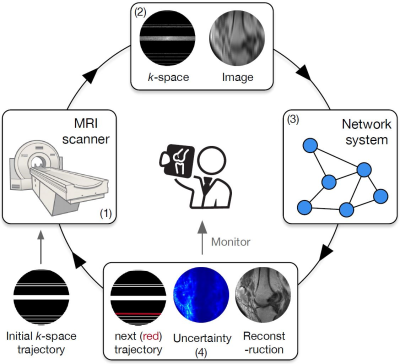 |
Active acquisition for MRI reconstruction

Zizhao Zhang, Adriana Romero, Matthew Muckley, Pascal Vincent, Michal Drozdzal
In this abstract, we introduce a novel pipeline for MRI reconstruction, which actively selects sampling trajectories in order to ensure high fidelity images, while speeding up the acquisition time. The pipeline is based on recent advances in deep learning and is composed of two networks interacting with each other in order to perform active acquisition. Results on a large scale knee dataset highlight the potential of the method when compared to standard acquisition heuristics. Moreover, we show that the learnt acquisition strategy efficiently reduces the reconstruction uncertainty and paves the way towards more applicable solutions for accelerating MRI.
|
09:03
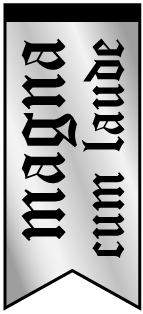 |
1094.
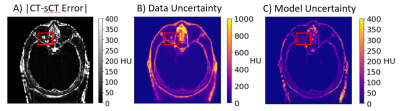 |
Estimating Model and Data Dependant Uncertainty for Synthetic-CTs obtained using Generative Adversarial Networks

Matt Hemsley, Brige Chugh, Mark Ruschin, Young Lee, Chia-Lin Tseng, Greg Stanisz, Angus Lau
The feasibility of using a neural network model to place uncertainty estimates on synthetic-CTs created with a generative adversarial network was investigated. Dropout-based variational inference was employed to account for the uncertainty on the trained model. The standard GAN loss function was also combined with an additional log-likelihood term, designed such that the network learns which regions of input data lead to highly variable output. On a dataset of n=105 brain patients, our results demonstrate that the predicted uncertainty can be interpreted as an upper bound on the true error with a confidence of approximately 95%.
|
09:15
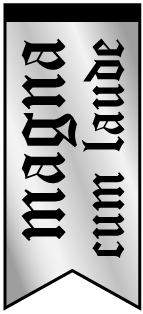 |
1095.
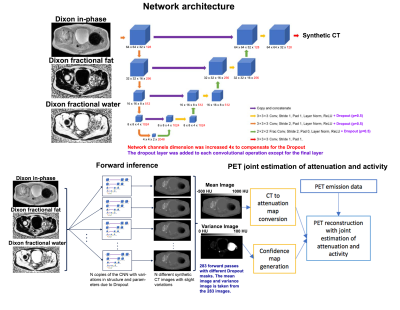 |
Deep learning-based uncertainty estimation: application in PET/MRI joint estimation of attenuation and activity for patients with metal implants

Andrew Leynes, Sangtae Ahn, Kristen Wangerin, Florian Wiesinger, Thomas Hope, Peder Larson
PET image reconstruction requires accurate estimates of attenuation coefficients. Metal implants corrupt both the MRI and CT images and thus are not suitable for use in image reconstruction. In particular, the metal implant appears as a large signal void in the MRI and is incorrectly estimated as having the attenuation coefficients of air. We proposed to use Bayesian deep learning to identify the location of the metal implant and use it to guide PET joint estimation of attenuation and activity. We found that the metal implant is recovered and lesion uptake near the implant agree well with our reference data.
|
09:27
|
1096.
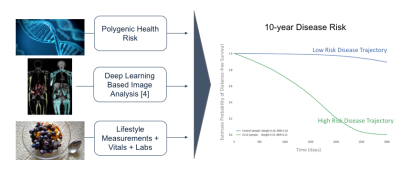 |
Predicting 10-Year Risk of Type 2 Diabetes Onset Using Lifestyle, Genomics, and Whole Body DIXON MR Imaging

Axel Bernal, Natalie Schenker-Ahmed, Alex Graff, Jian Wu, Dmitry Tkach, David Karow, Christine Swisher
Diabetes Mellitus is an important factor in the onset and progression of many related serious conditions. It is also very actionable and preventable thus the need for improved risk assessment to identify high-risk individuals early.
In this study we use lifestyle, genomic, MRI features and Cox Proportional Hazard models1 to improve DM risk assessment. To our knowledge, this is the first demonstration of integrating these features for assessing type 2 DM risk. Our final cross-validated concordance index is 84%, 4% of which is due to MRI features. On average our models can predict up to ten years into the future.
|
09:39
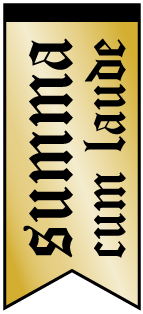 |
1097.
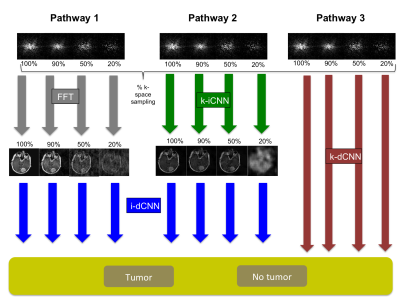 |
Convolutional neural network-based image reconstruction and image classification: atomization or amalgamation?

Sarah Eskreis-Winkler, Zhe Liu, Jinwei Zhang, Pascal Spincemaille, Thanh Nguyen, Ilhami Kovanlikaya, Yi Wang
Convolutional neural networks have emerged as a powerful tool for image reconstruction and image analysis. In this abstract, we evaluate whether image reconstruction and image classification tasks are best performed separately, or whether a combined CNN, performing image reconstruction and clinical diagnosis steps in tandem, delivers synergistic effects.
|
09:51
|
1098.
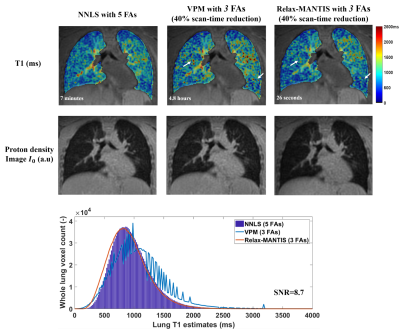 |
Relax-MANTIS: REference-free LAtent map-eXtracting MANTIS for efficient MR parametric mapping with unsupervised deep learning

Wei Zha, Sean Fain, Richard Kijowski, Fang Liu
The purpose of this work was to develop and evaluate a novel deep learning-based framework termed Reference-free Latent map-eXtracting MANTIS (Relax-MANTIS) for efficient MR parameter mapping. Our approach incorporated end-to-end CNN mapping, the concept of cyclic loss to enforce data fidelity and without the need of explicit training references. Our results demonstrated that the proposed framework produced accurate and robust T1 mapping in knee and low-SNR lung UTE MRI. The good quantitative agreement to the reference method suggests that Relax-MANTIS allows potentially accelerated quantitative mapping without modifications of scan protocol and sequence for high-resolution knee and whole lung T1 quantification.
|
10:03
|
1099.
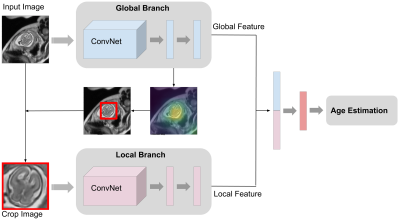 |
Deep Learning with Attention to Predict Gestational Age of the Fetal Brain Using MRI

Liyue Shen, Katie Shpanskaya, Edward Lee, Emily McKenna, Maryam Maleki, Quin Lu, John Pauly, Kristen Yeom
Fetal brain imaging is a cornerstone of prenatal screening and early diagnosis of congenital anomalies. Knowledge of fetal gestational age is the key to the accurate assessment of brain development. This study develops an attention-based deep learning model to predict gestational age of fetal brain. The proposed model is an end-to-end framework that combines key insights from multi-view MRI including axial, coronal, and sagittal views. The model uses age-activated weakly-supervised attention maps to enable rotation-invariant localization of fetal brain among background noise. We evaluate our method on a collected fetal brain MRI cohort and achieve promising age prediction performance.
|
|