Image Processing & Analysis
Oral
Acquisition, Reconstruction & Analysis
Wednesday, 15 May 2019
Room 510A-D |
13:30 - 15:30 |
Moderators: Konstantinos Arfanakis, Marion Smits |
13:30
|
0831.
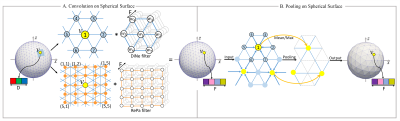 |
Spherical U-Net for Infant Cortical Surface Parcellation

Fenqiang Zhao, Shunren Xia, Zhengwang Wu, Li Wang, Weili Lin, John Gilmore, Dinggang Shen, Gang Li
In neuroimaging studies, it is of great importance to accurately parcellate cortical surfaces (with a spherical topology) into meaningful regions. In this work, we propose a novel end-to-end deep learning method by formulating surface parcellation as a semantic segmentation task on the spherical space. To extend the convolutional neural networks (CNNs) to the spherical space, a set of corresponding operations are first developed, and then spherical CNNs are constructed accordingly. Specifically, the U-Net and SegNet architectures are transformed to parcellate infant cortical surfaces. Experiments on 90 neonates indicate the superiority of our proposed spherical U-Net in comparison with other methods.
|
13:42
|
0832.
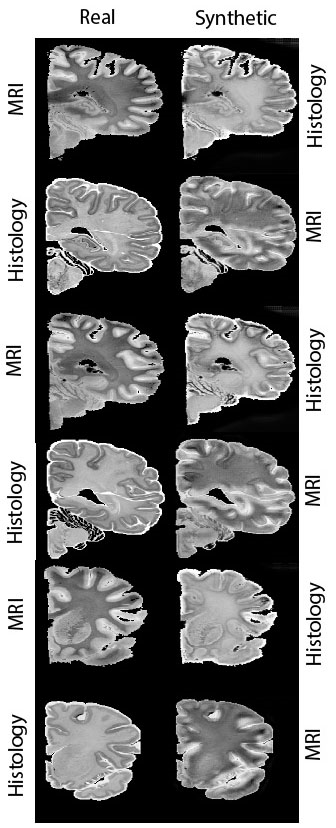 |
Segmentation-aware adversarial synthesis for registration of histology to MRI

Matteo Mancini, Yuankai Huo, Bennett Landman, Juan Eugenio Iglesias
MRI-histology registration lays the ground for a new generation of high-resolution brain atlases. The task is challenging given the different contrast and the histology-related artifacts. We propose a dataset-specific, synthesis-based approach that uses a generative adversarial network to reduce the problem to intra-modality registration. Exploiting automatic segmentation data and cycle-consistency, the proposed architecture is suitable for small-size datasets. We show the advantages of this approach compared to canonical registration both in quantitative and qualitative terms using data from the Allen Institute’s Human Brain Atlas.
|
13:54
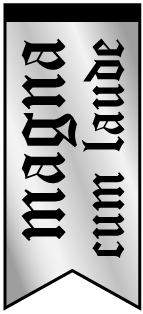 |
0833.
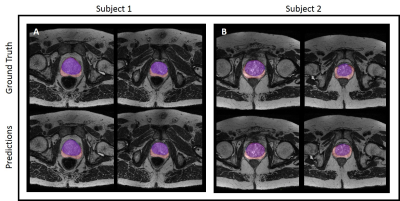 |
A Cascaded Residual UNET for Fully Automated Segmentation of prostate and peripheral zone in T2-weighted 3D Fast Spin Echo Images

Lavanya Umapathy, Wyatt Unger, Faryal Shareef, Hina Arif, Diego Martin, Maria Altbach, Ali Bilgin
Multi-parametric MR images have been shown to be effective in the non-invasive diagnosis of prostate cancer. Automated segmentation of the prostate eliminates the need for manual annotation by a radiologist which is time consuming. This improves efficiency in the extraction of imaging features for the characterization of prostate tissues. In this work, we propose a fully automated cascaded deep learning architecture with residual blocks (Cascaded MRes-UNET) for segmentation of the prostate gland and the peripheral zone in one pass through the network. The network yields high dice scores (mean=0.91) with manual annotations from an experienced radiologist. The average difference in volume estimation is around 6% in the prostate and 3% in the peripheral zone.
|
14:06
|
0834.
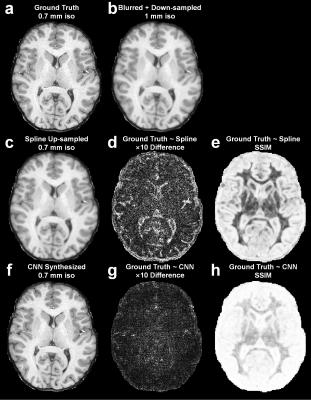 |
SuperSurfer: Cortical surface reconstruction using super-resolution anatomical MR images synthesized by deep learning

Qiyuan Tian, Berkin Bilgic, Qiuyun Fan, Chanon Ngamsombat, Akshay Chaudhari, Ned Ohringer, Yuxin Hu, Thomas Witzel, Kawin Setsompop, Jonathan Polimeni, Susie Huang
Recent studies have shown that anatomical MR images with sub-millimeter resolution can improve the accuracy of cortical surface and thickness estimation compared to the standard 1-millimeter isotropic resolution. Here we propose a new method, entitled SuperSurfer, that synthesizes sub-millimeter anatomical MR images from standard 1-mm isotropic anatomical images using a convolutional neural network-based super-resolution approach intended for improved cortical surface reconstruction. We quantified the displacement of the reconstructed surfaces and difference in cortical thickness derived from the super-resolution and standard-resolution data and demonstrated that SuperSurfer provides improved cortical surfaces that are similar to those obtained from native sub-millimeter resolution data.
|
14:18
|
0835.
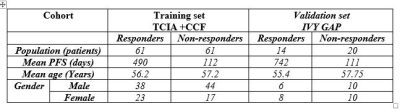 |
Radiomic features from enhancing tumor on pre-treatment multiparametric MRI scans are predictive of response to chemo-radiation therapy in Glioblastoma and are associated with histological phenotypes

Ruchika Verma, Ramon Correa, Virginia Hill, Niha Beig, Abdelkader Mahammedi, Marwa Ismail, Anant Madabhushi, Pallavi Tiwari
We presented the initial results of employing 3D radiomic descriptors from pre-treatment MP-MRI scans for tumor risk stratification based on patient’s response to chemo-radiation treatment. We demonstrated that the CoLlAGe (captures tumor heterogeneity) and Laws (captures Levels, waves, and ripple appearances) features from the enhancing region were most predictive of response to CRT. These features were also found to be associated with histologic attributes including cellular tumor, infiltrating tumor, and hyperplastic blood vessels, each of which is known to contribute to treatment resistance in the tumor microenvironment
|
14:30
|
0836.
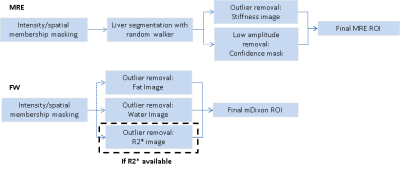 |
Automated Analysis of MR Elastography and Quantitative Fat-Water Imaging

Bogdan Dzyubak, Jiahui Li, Sudhakar Venkatesh, Kevin Glaser, Alina Allen, Meng Yin, Richard Ehman
Evaluation of liver health with quantitative MRI addresses an issue of major global importance. This work extends ALEC, a previously validated automated method for defining ROIs and reporting liver stiffness from MR elastography images, to reporting fat fraction and R2* from multipoint Dixon images. The tool achieves excellent correlation with an expert reader in 102 clinical exams and allows multiparametric quantitative liver MRI exams to be analyzed in a highly reproducible way within 5 minutes.
|
14:42
|
0837.
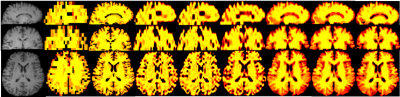 |
Super-resolution 3D MRSI for Mapping 2HG and Tumor Metabolism in Patients with Mutant IDH Glioma

Xianqi Li, Bernhard Strasser, Kourosh Jafari-Khouzani, Bijaya Thapa, Julia Small, Daniel P Cahill, Jorg Dietrich, Tracy T Batchelor, Ovidiu Andronesi
To improve the spatial resolution of 3D MRSI, a feature-based nonlocal means approach utilizing the structural information of high-resolution MR images is proposed. By estimating similarity between voxels using a feature vector that characterizes the laminar pattern of brain structures, a more accurate similarity measure is achieved compared to conventional upsampling methods. The preliminary results on simulated and in vivo data indicate the proposed method has great potential for clinically neuroimaging applications.
|
14:54
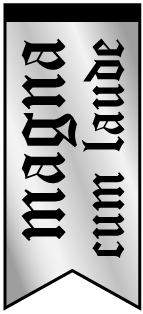 |
0838.
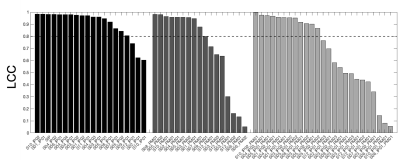 |
Evaluating multi-site rCBV consistency from DSC-MRI imaging protocols and post-processing software across the NCI Quantitative Imaging Network sites using a Digital Reference Object

Laura Bell, Natenael Semmineh, Hongyu An, Cihat Eldeniz, Richard Wahl, Kathleen Schmainda, Melissa Prah, Bradley Erickson, Panagiotis Korfiatis, Chengyue Wu, Anna Sorace, Neal Rutledge, Thomas Yankeelov, Thomas Chenevert, Dariya Malyarenko, Yichu Liu, Andrew Brenner, Leland Hu, Yuxiang Zhou, Jerrold Boxerman, Yi-Fen Yen, Jayashree Kalpathy-Cramer, Andrew Beers, Mark Muzi, Ananth Madhuranthakam, Marco Pinho, Brian Johnson, C. Quarles
Differences in imaging protocols (IP) and post-processing methods (PM) may influence relative cerebral blood volume (rCBV). Our goal was to leverage a dynamic susceptibility contrast (DSC) DRO to characterize rCBV consistency across 12 sites, focusing on differences due to site-specific IPs and/or PMs. Our results indicate high agreement when one center processes rCBV despite slight variations in the IP. However, substantial disagreement was observed when site-specific software was applied for rCBV measurements. These results have important implications for comparing DSC-MRI data across sites/trials, where PM variability could confound the use of rCBV as a biomarker of therapy response.
|
15:06
|
0839.
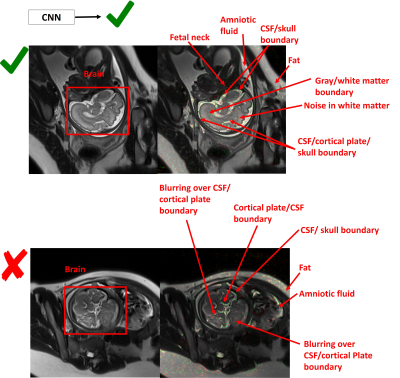 |
A Deep Learning Approach for Image Quality Assessment of Fetal Brain MRI

Sayeri Lala, Nalini Singh, Borjan Gagoski, Esra Turk, P. Grant, Polina Golland, Elfar Adalsteinsson
Fetal MRI plays a critical role in diagnosing brain abnormalities but routinely suffers from artifacts resulting in nondiagnostic images. We aim to automatically identify nondiagnostic images during acquisition so they can be immediately flagged for reacquisition. As a first step, we trained a neural network to classify T2-weighted single-shot fast spin-echo (HASTE) images as diagnostic or nondiagnostic. With novel data, the average Area Under Receiver Operator Characteristic Curve was 0.84 (σ = 0.04). The neural network learned relevant criteria, identifying high contrast boundaries between areas like cerebral spinal fluid and cortical plate as relevant to determining image quality.
|
15:18
|
0840.
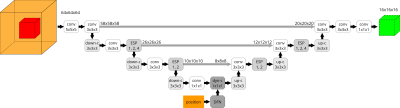 |
Automated Deep Learning based 3D Hip Segmentation in PD-weighted MR images of a large-scale cohort study

Marc Fischer, Sven Walter, Christian Klinger, Thomas Küstner, Bin Yang, Mike Notohamiprodjo, Fritz Schick
Analysis of geometrical and structural properties of the hip is of great importance to allow for meaningful comparison of significant findings. Especially with regard to large cohort studies manual processing of large 3D volumes becomes infeasible and thus automated processing is required. In this work, a Deep Learning driven algorithm is proposed which performs automated hip segmentation of 3D MRI datasets, requiring few training data and being able to perform accurate semantic bone segmentation in spite of complex anatomical structures sharing similar tissue characteristics.
|
|