ORGANIZERS: Meiyun Wang, John Port
Wednesday Parallel 2 Live Q&A |
Wednesday, 12 August 2020, 14:30 - 15:15 UTC |
Moderators: John Port & Liesbeth Reneman |
Skill Level: Basic to Advanced
Session Number: C-Tu-01
Overview
MR, in conjunction with the application of artificial intelligence, has been a powerful tool for psychoradiology, a new branch of radiology. These advancements are not only providing a insightful understanding of the underlying pathological mechanism, but also expediting the translation of psychoradiological discoveries into clinical care for the patients with neuropsychiatric disorders.
Target Audience
Clinicians and researchers interested in the imaging of neuropsychiatric disorders.
Educational Objectives
As a result of attending this course, participants should be able to:
- Explain the basics and current state of psychoradiology;
- Describe the key MR features in major neuropsychiatric disorders;
- Describe the AI techniques for identifying psychoradiological markers and subtyping the pathology for patients with major neuropsychiatric disorders; and
- Evaluate the emerging imaging techniques that could impact clinical applications to psychiatric illnesses.
|
|
Frontiers in Psychoradiology
Qiyong Gong
Watch the Video
With the AI technical development, psychoradiology is primed to assist clinician for improving the clinical care of the psychiatric patients.
|
|
|
Machine Learning: Methods and Applications to Brain Disorders
Conor Liston Watch the Video
|
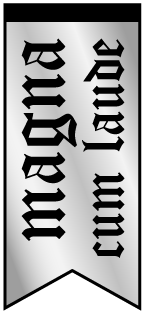 |
0794.
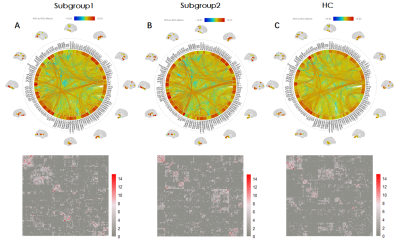 |
Clustering analysis differentiates clinical subtypes of major depressive disorder that identify symptom-specific brain connectivity
Shi Tang1, Yanlin Wang1, Yongbo Hu1, Lu Lu1, Lianqing Zhang1, Xuan Bu1, Hailong Li1, Yingxue Gao1, Lingxiao Cao1, Xinyue Hu1, Jing Liu1, Xinyu Hu1, Weihong Kuang2, Qiyong Gong1,
and Xiaoqi Huang1
1Huaxi MR Research Center (HMRRC), Functional and molecular imaging Key Laboratory of Sichuan Province, Department of Radiology, West China Hospital, Sichuan University, Chengdu 610041, China, Chengdu, China, 2Department of psychiatry, West China Hospital, Sichuan University, Chengdu 610041, China, Chengdu, China
Watch the Video
Functional connectivity/network analyses using fMRI data have been applied to characterize diagnostic biomarkers in MDD. However, the association between brain connection and dimensional symptoms of this heterogeneous syndrome still remains unclear. In this work, we focused on first-episode and unmedicated MDD patients, firstly using unsupervised clustering analysis differentiated them into two subgroups on the basis of clinical features. Also, we compared the brain connectivity among subgroups plus healthy people. Then we used multivariate methods identified which clinical symptoms are significantly influenced by which brain connectivity. Our results may provide neurobiological mechanisms of MDD symptoms and serve as effective diagnostic biomarkers.
|
|
0795.
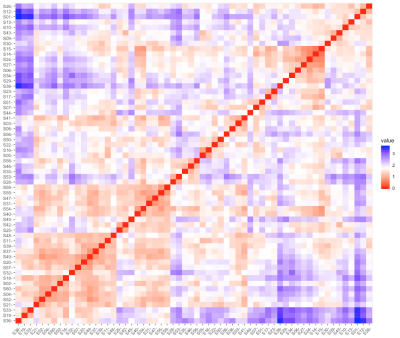 |
Relapse Risk Revealed by Degree Centrality and Cluster Analysis in Heroin Addicts Undergoing Methadone Maintenance Treatment
Yarong Wang1 and Lei Wang2
1Department of Radiology, The First Affiliated Hospital of Xi'an Jiaotong University, Xi'an, China, 2Department of nuclear medicine, Tangdu Hospital of Air Force Medical University of PLA, Xi'an, China
Watch the Video
The objective of this study is to identify the heroin dependents undertaking stable methadone maintenance treatment (MMT patients) at high risk for opioid relapse prospectively. First, a self-defined addiction-related brain network was constructed with 10 hubs of several circuits associated with addiction and their degree centrality. Next, sixty male MMT patients was classified into different subgroups through grouping their addiction-related network into distinct neuronal activity patterns by K-means clustering algorithm. By comparing relapse rate between subgroups with distinct network pattern, the one at high risk for relapse was identified. This finding implicated a novel strategy for improving MMT therapeutic effect.
|
|
0796.
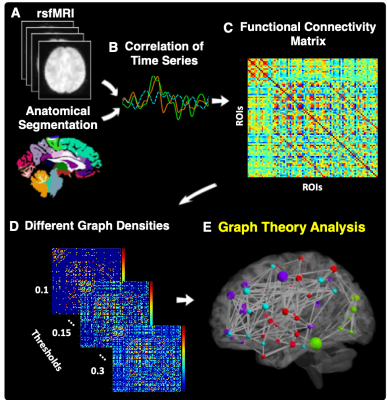 |
Aberrant Functional Brain Network Topology for Classification between Major Depressive Disorder and Healthy Controls
Yael Jacob1, Laurel S Morris1, Kuang-Han Huang1, Molly Schneider1, Gaurav Verma1, James W Murrough1, and Priti Balchandani1
1Icahn School of Medicine at Mount Sinai, New York, NY, United States
Watch the Video
Currently, diagnosis for major depressive disorder (MDD) is largely reliant on self-reported symptoms. The ability to identify MDD without self-report is greatly needed. Implementing a graph-theoretical analysis on resting state fMRI (rsfMRI), we tested whether whole-brain network topology can be used as predictors of MDD using a machine learning algorithm. We found that MDD patients exhibit aberrant network centrality measures within the right hippocampus, supramarginal and parsopercularis. Using these as predictors in a machine learning algorithm we were able to classify MDD and controls with total accuracy of 81%, demonstrating the applicability of rsfMRI for diagnostics of MDD.
|
|
0797.
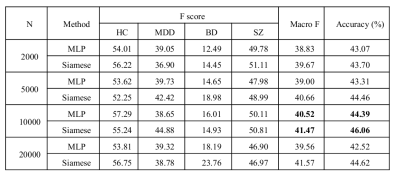 |
Multi-class identification for major depression, bipolar disorder and schizophrenia based on Siamese Network
Chao Li1,2,3, Yue Cui1,2,3, Yongfeng Yang4, Jing Sui1,2,3, Luxian Lv4, and Tianzi Jiang1,2,3
1Brainnetome Center, Institute of Automation, Chinese Academy of Sciences, Beijing, China, 2National Laboratory of Pattern Recognition, Institute of Automation, Chinese Academy of Sciences, Beijing, China, 3University of Chinese Academy of Sciences, Beijing, China, 4Department of Psychiatry, Henan Mental Hospital, The Second Affiliated Hospital of Xinxiang Medical University, Xinxiang, China
Siamese Network is an artificial neural network that has been used in small sample sets multi-class classification studies. This study identified major depressive disorder (MDD), bipolar disorder (BD), and schizophrenia (SZ) based on combined gray matter, white matter and cerebrospinal fluid using Siamese Network. The participants included four groups: MDD (n = 102), BD (n = 44), SZ (n = 114), and healthy controls (n = 103). We found Siamese Network achieved improved performance than the multilayer perception network with different numbers of features. We achieved a classification accuracy of 46.06% and Macro F1 of 41.47% for this multi-class identification.
|
|