ORGANIZERS: Jennifer Steeden, Jennifer Keegan
Wednesday Parallel 1 Live Q&A |
Wednesday, 12 August 2020, 14:30 - 15:15 UTC |
Moderators: Eric Gibbons & Pedro Ferreira |
Skill Level: Basic to Advanced
Session Number: C-Tu-03
Overview
This session will provide an overview of current and future applications of machine learning in cardiovascular MRI.
Target Audience
Physicists and clinicians who wish to understand how machine learning is being applied to cardiovascular acquisition, reconstruction, and processing.
Educational Objectives
As a result of attending this course, participants should be able to:
- Explain how machine learning can be used in cardiovascular imaging;
- Describe applications of machine learning in current clinical cardiovascular practice;
- Recognize the requirements for machine learning and its limitations; and
- Describe ways in which machine learning will impact future cardiovascular practice.
|
|
Applications of Machine Learning in Clinical Cardiovascular MRI
Albert Hsiao Watch the Video
|
|
|
Machine Learning & Future Clinical Practice in Cardiovascular MRI
Claudia Prieto Watch the Video
|
|
0769.
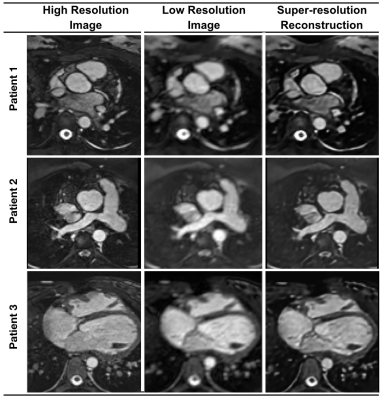 |
Rapid Whole-Heart CMR with Single Volume Super-Resolution
Jennifer Steeden1, Michael Quail2, Alexander Gotschy2,3, Andreas Hauptmann1,4, Rodney Jones1, and Vivek Muthurangu1
1University College London, London, United Kingdom, 2Great Ormond Street Hospital, London, United Kingdom, 3University and ETH Zurich, Institute for Biomedical Engineering, Zurich, Switzerland, 4University of Oulu, Oulu, Finland
Watch the Video
Three-dimensional (3D), whole heart, balanced steady state free precession (WH-bSSFP) sequences provides excellent delineation of both intra-cardiac and vascular anatomy. However, they are usually cardiac triggered and respiratory navigated, resulting in long acquisition times (10-15minutes). Here, we propose a machine-learning single-volume super-resolution reconstruction (SRR), to recover high-resolution features from rapidly acquired low-resolution WH-bSSFP data. We show that it is possible to train a network using synthetically down-sampled WH-bSSFP data. We tested the network on synthetic test data and 40 prospective data sets, showing ~3x speed-up in acquisition time, with excellent agreement with reference standard high resolution WH-bSSFP images.
|
|
0770.
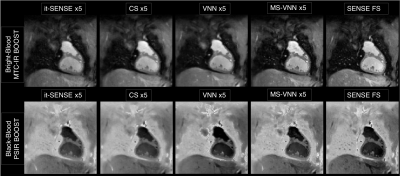 |
A Multi-Scale Variational Neural Network for accelerating bright- and black-blood 3D whole-heart MRI in patients with congenital heart disease
Niccolo Fuin1, Giovanna Nordio1, Thomas Kuestner1, Radhouene Neji2, Karl Kunze2, Yaso Emmanuel3, Alessandra Frigiola1,3, Rene Botnar1,4, and Claudia Prieto1,4
1Biomedical Engineering Department, School of Biomedical Engineering and Imaging Sciences, King's College London, London, United Kingdom, 2MR Research Collaborations, Siemens Healthcare Limited, Frimley, United Kingdom, 3Guy’s and St Thomas’ Hospital, NHS Foundation Trust, London, United Kingdom, 4Pontificia Universidad Católica de Chile, Santiago, Chile
Watch the Video
Bright- and black-blood MRI sequences provide complementary diagnostic information in patients with congenital heart disease (CHD). A free-breathing 3D whole-heart sequence (MTC-BOOST) has been recently proposed for contrast-free simultaneous bright- and black-blood MRI, demonstrating high-quality depiction of arterial and venous structures. However, high-resolution fully-sampled MTC-BOOST acquisitions require long scan times of ~12min. Here we propose a joint Multi-Scale Variational Neural Network (MS-VNN) which enables the acquisition of high-quality bright- and black blood MTC-BOOST images in ~2-4 minutes, and their joint reconstruction in ~20s. The technique is compared with Compressed-Sensing reconstruction for 5x acceleration, in CHD patients.
|
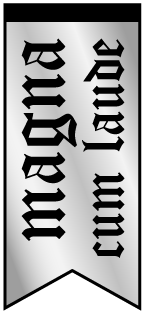 |
0771.
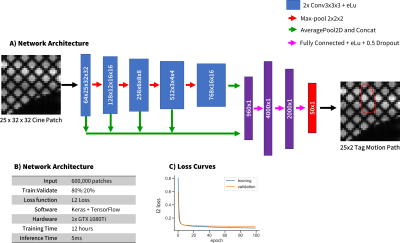 |
Cardiac Tag Tracking with Deep Learning Trained with Comprehensive Synthetic Data Generation
Michael Loecher1, Luigi E Perotti2, and Daniel B Ennis1,3,4,5
1Radiology, Stanford, Palo Alto, CA, United States, 2Mechanical Engineering, University of Central Florida, Orlando, FL, United States, 3Radiology, Veterans Administration Health Care System, Palo Alto, CA, United States, 4Cardiovascular Institute, Stanford, Palo Alto, CA, United States, 5Center for Artificial Intelligence in Medicine & Imaging, Stanford, Palo Alto, CA, United States
Watch the Video
A convolutional neural network based tag tracking method for cardiac grid-tagged data was developed and validated. An extensive synthetic data simulator was created to generate large amounts of training data from natural images with analytically known ground-truth motion. The method was validated using both a digital cardiac deforming phantom and tested using in vivo data. Very good agreement was seen in tag locations (<1.0mm) and calculated strain measures (<0.02 midwall Ecc)
|
|
0772.
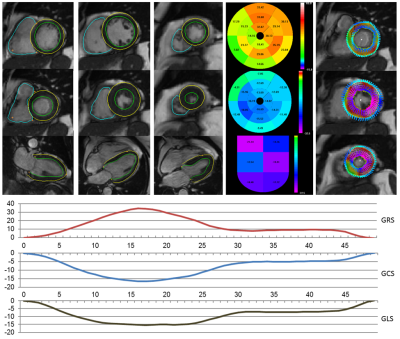 |
Deep Learning-based Strain Quantification from CINE Cardiac MRI
Teodora Chitiboi1, Bogdan Georgescu1, Jens Wetzl2, Indraneel Borgohain1, Christian Geppert2, Stefan K Piechnik3, Stefan Neubauer3, Steffen Petersen4, and Puneet Sharma1
1Siemens Healthineers, Princeton, NJ, United States, 2Magnetic Resonance, Siemens Healthcare, Erlangen, Germany, 3Division of Cardiovascular Medicine, Radcliffe Department of Medicine, University of Oxford, Oxford, United Kingdom, 4NIHR Biomedical Research Centre at Barts, Queen Mary University of London, London, United Kingdom
Watch the Video
Deep learning enables fully automatic strain analysis from CINE MRI on large subject cohorts. Deep learning neural nets were trained to segment the heart chambers from CINE MRI using manually annotated ground truth. After validation on more than 1700 different patient datasets, the models were used to generate segmentations as the first step of a fully automatic strain analysis pipeline for 460 subjects. We found significant differences associated with gender (strain magnitude smaller for males), height (lower strain magnitude for patients taller than 170 cm) and age (lower circumferential and longitudinal strain for subjects older than 60 years).
|
|
0773.
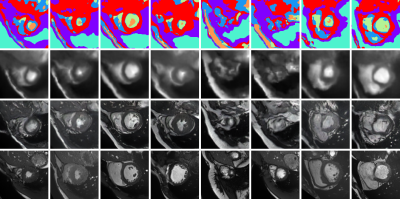 |
Leveraging Anatomical Similarity for Unsupervised Model Learning and Synthetic MR Data Generation
Thomas Joyce1 and Sebastian Kozerke1
1Institute for Biomedical Engineering, University and ETH Zurich, Zurich, Switzerland
Watch the Video
We present a method for the controllable synthesis of 3D (volumetric) MRI data. The model is comprised of three components which are learnt simultaneously from unlabelled data through self-supervision: i) a multi-tissue anatomical model, ii) a probability distribution over deformations of this anatomical model, and, iii) a probability distribution over ‘renderings’ of the anatomical model (where a rendering defines the relationship between anatomy and resulting pixel intensities). After training, synthetic data can be generated by sampling the deformation and rendering distributions.
|
|