Digital Poster Session
Musculoskeletal: Machine Learning and Emerging Methods in MSK
Back to Program-at-a-Glance
|
Digital Posters Musculoskeletal
2650 -2664 Machine Learning and Emerging Methods in MSK - Machine Learning in Musculoskeletal 1
2665 -2679 Machine Learning and Emerging Methods in MSK - Musculoskeletal Emerging Methods/Machine Learning 2
2680 -2695 Machine Learning and Emerging Methods in MSK - Musculoskeletal Emerging Methods
|
|
|
2650.
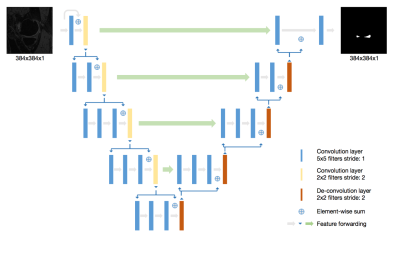 |
Deep-learning Methods for Meniscus Segmentation in Knee MRI
Siyue Li1, Shutian Zhao1, Sheheryark Khan1, and Weitian Chen1
1CUHK lab of AI in radiology (CLAIR), Department of imaging and interventional radiology , The Chinese university of Hong Kong, HongKong, Hong Kong
View the Poster
Meniscus segmentation in MR images has important clinical applications. Due to its large shape variation and low contrast with surrounding pixels, it is challenging to perform robust and reliable meniscus segmentation automatically. We investigated deep-learning network V-Net approaches for meniscus segmentation using MR images.
|
|
2651.
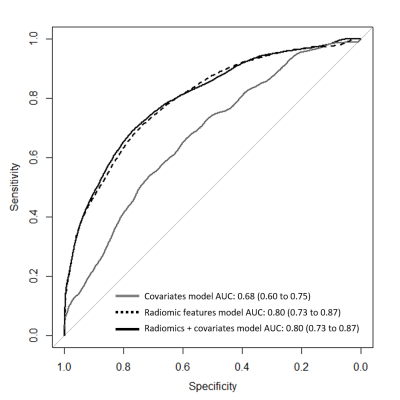 |
Discrimination of subjects with and without knee osteoarthritis using a radiomics approach on tibial bone
Jukka Hirvasniemi1, Stefan Klein2, Dieuwke Schiphof3, and Edwin Oei1
1Department of Radiology & Nuclear Medicine, Erasmus MC, Rotterdam, Netherlands, 2Department of Radiology & Nuclear Medicine and Department of Medical Informatics, Erasmus MC, Rotterdam, Netherlands, 3Department of General Practice, Erasmus MC, Rotterdam, Netherlands
View the Poster
Radiomic features were automatically extracted from tibial bone using knee MRI data of 665 women and the ability of the features to discriminate subjects with and without osteoarthritis was assessed. An area under the receiver operating characteristics curve of 0.80 was obtained for classifying subjects with and without osteoarthritis using an elastic net regression model that included radiomic features and covariates. Our results indicate that tibial bone characteristics are different between subjects with and without knee osteoarthritis.
|
|
2652.
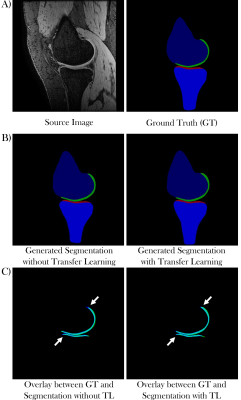 |
Automated Segmentation of Knee Articular Cartilage on MRI Data: Increasing Network Capacity with Transfer Learning
Dimitri A Kessler1, James W MacKay1,2, Fiona J Gilbert1, Martin J Graves1, and Joshua D Kaggie1
1Department of Radiology, University of Cambridge, Cambridge, United Kingdom, 2Norwich Medical School, University of East Anglia, Norwich, United Kingdom
View the Poster
In this study we evaluated the possibility of using transfer learning to improve the segmentation accuracy of femoral and tibial knee articular cartilage of a small locally acquired and annotated dataset. Two conditional Generative Adversarial Networks were trained - one with pretraining on the much larger SKI10 (Segmentation of Knee Images 2010) dataset and the other with random weight initialisation and no pretraining. Pretraining not only increased cartilage segmentation accuracy of the fine-tuned dataset, but also increased the network’s capacity to preserve segmentation capabilities for the pretrained dataset.
|
|
2653.
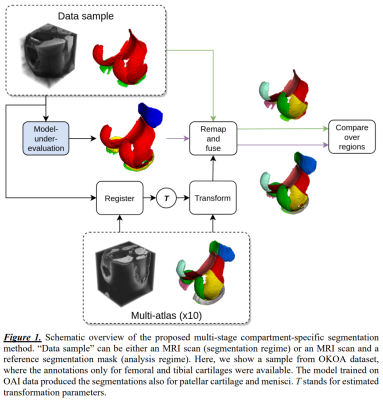 |
Compartment-specific Knee Cartilage Segmentation using Deep Learning
Egor Panfilov1, Aleksei Tiulpin1,2, Victor Casula1, Simo Saarakkala1,2, and Miika T. Nieminen1,2
1Research Unit of Medical Imaging, Physics and Technology, University of Oulu, Oulu, Finland, 2Department of Diagnostic Radiology, Oulu University Hospital, Oulu, Finland
View the Poster
In this study, we developed a method for compartment-specific segmentation of knee cartilage from 3D-DESS MR images which jointly utilizes deep learning and atlas-based approaches. The method was applied to compare the performance of two deep learning-based segmentation models on two independent datasets. One of the models achieved new state-of-the-art in knee cartilage segmentation on the Osteoarthritis Initiative data and was more robust to the changes in MRI protocol. Detailed analysis performed using our method showed how the performance improvements are localized compartment-wise. The method can be used to select the most accurate segmentation model for the considered clinical problem.
|
|
2654.
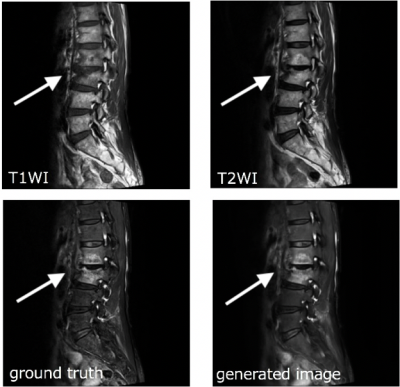 |
Deep learning fat-suppressed images for musculoskeletal diseases
Shimpei Kato1,2, Akihiko Wada1, Yuya Saito1,3, Christina Andica1, Shohei Fujita1,2, Kotaro Fujimoto1,2, Yutaka Ikenouchi1, Akifumi Hagiwara1, Junko Kikuta4, Kanako Sato1,2, Michimasa Suzuki1, Toshiaki Akashi1, Maki Amano1,
Koji Kamagata1, Kanako Kumamaru1,2, Masaaki Hori1,5, Atsushi Nakanishi1, Osamu Abe2, and Shigeki Aoki1
1Department of Radiology, Juntendo University, Tokyo, Japan, 2Department of Radiology, The University of Tokyo, Tokyo, Japan, 3Faculty of Health Sciences Graduate School of Human Health Sciences, Tokyo Metropolitan University, Tokyo, Japan, 4Department of Radiology, Juntendo University Nerima Hospital, Tokyo, Japan, 5Department of Radiology, Toho University, Tokyo, Japan
View the Poster
Fat-suppressed MR images of the musculoskeletal system help the visualization of T2-prolonged lesions, such as tumors, infections/inflammations, and trauma, with better contrast, while also contributing to the qualitative diagnosis of fatty lesions.However, the addition of a fat-suppressing sequence to clinical routine is time-consuming. Increasing the imaging time may lead to deterioration of the image quality due to body movement.In this study, we generated fat-suppressed images through post-processing by using deep learning. The images were generated using U-Net, with T1WIs and T2WIs as input. The generated images were very similar to Dixon images that were used as targets.
|
|
2655.
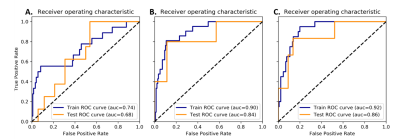 |
Texture Analysis of T2-weighted Lumbar Spine MRI Predicts Presence of Low Back Pain
Juuso Heikki Jalmari Ketola1, Satu Irene Inkinen1, Jaro Karppinen2, Jaakko Niinimäki1,2,3, Osmo Tervonen1,2,3, and Miika Tapio Nieminen1,2,3
1Research Unit of Medical Imaging, Physics and Technology, University of Oulu, Oulu, Finland, 2Medical Research Center, University of Oulu and Oulu University Hospital, Oulu, Finland, 3Department of Diagnostic Radiology, Oulu University Hospital, Oulu, Finland
View the Poster
We applied texture analysis to T2-weighted MRI of the lumbar spine in a population-based sample. The extracted features were used in a logistic regression pipeline to predict whether the subjects (N=200) suffered from clinically relevant low back pain. Best results were obtained by combining features from intervertebral discs and vertebrae with receiver operating characteristics area under curve of 0.86, accuracy of 0.84, and recall of 0.83. This preliminary work shows that texture analysis and machine learning may be used to predict pain from T2-weighted images. Thus, a connection between MRI textural features and clinically relevant low back pain may exist.
|
|
2656.
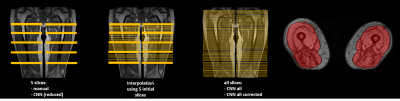 |
Convolutional neural network automatic global segmentation of thigh muscle water-fat images in neuromuscular diseases
Harmen Reyngoudt1,2, Eduard Snezhko3, Pierre-Yves Baudin4, and Pierre G. Carlier1,2
1NMR Laboratory, Neuromuscular Investigation Center, Institute of Myology, Paris, France, 2NMR Laboratory, CEA/DRF/IBFJ/MIRCen, Paris, France, 3United Institute for Informatics Problems, National Academy of Sciences, Minsk, Belarus, 4Consultants for Research in Imaging and Spectroscopy, Tournai, Belgium
View the Poster
Manual segmentation of skeletal muscles in quantitative NMRI studies is a laborious task. In this work, deep learning using a convolutional neural network (CNN) was applied for segmenting the global thigh segment and assessing the muscle fatty replacement over 1 year in patients with several neuromuscular pathologies. A series of 425 Dixon data sets, obtained at 3 T, were used for this purpose. Dice coefficients of 0.97 were obtained when comparing manual and CNN based segmentation. Standardized response means for the fat fraction evolution over 1 year using CNN were at least as high as results obtained with manual segmentation.
|
|
2657.
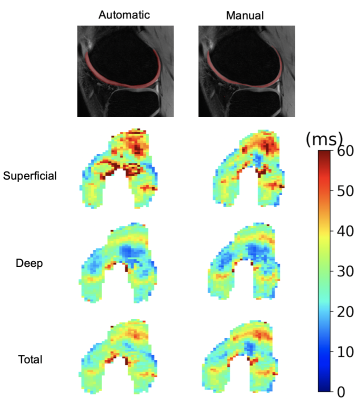 |
Generalizability of Deep-Learning Segmentation Algorithms on Independent Datasets for Measuring T2 Relaxation Times
Andrew Schmidt1, Arjun D Desai1, Lauren E Watkins2, Hollis Crowder3, Elka Rubin1, Valentina Mazzoli1, Quin Lu4, Marianne S Black1,3, Feliks Kogan1, Garry E Gold1,2, Brian A Hargreaves1,5, and Akshay S Chaudhari1
1Radiology, Stanford University, Palo Alto, CA, United States, 2Bioengineering, Stanford University, Palo Alto, CA, United States, 3Mechanical Engineering, Stanford University, Palo Alto, CA, United States, 4Philips Healthcare North America, Gainesville, FL, United States, 5Electrical Engineering, Stanford University, Palo Alto, CA, United States
Watch the Video
Automated segmentation using deep learning can potentially expedite segmentation tasks. However, the generalizability of such algorithms on new unseen datasets is unknown. To test this generalizability, we used a knee segmentation algorithm trained on Osteoarthritis Initiative double-echo steady-state (DESS) datasets to segment cartilage from quantitative DESS datasets from three independent studies. We compared manual-automatic segmentation accuracy and the resultant qDESS T2 map variations. These results quantitatively demonstrate that a deep learning network trained on a single dataset does not generalize with a high accuracy to additional datasets even with similar image characteristics, and that additional fine-tuning may be needed.
|
|
2658.
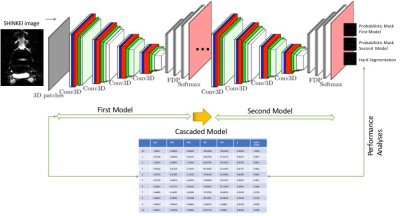 |
A 3D Convolutional Deep Neural Network for lumbar plexus segmentation
Kevin Bronik1, Marios Yiannakas2, Claudia A. M. Gandini Wheeler-Kingshott3, Daniel Alexander1, and Ferran Prados Carrasco4
1Center for Medical Image Computing, Department of Medical Physics and Biomedical Engineering, UCL, London, United Kingdom, 2Queen Square Multiple Sclerosis Centre, Department of Neuroinflammation, UCL Queen Square Institute of Neurology, UCL, London, United Kingdom, 3Department of Brain and Behavioural Sciences, University of Pavia, Pavia, Italy, Brain MRI 3T Research Center, IRCCS Mondino Foundation, Pavia, Italy, NMR Research Unit, Queen Square MS Centre, Department of Neuroinflammation, UCL Queen Square Institute of Neurology, Faculty of Brain Sciences, UCL, London, United Kingdom, 4Universitat Oberta de Catalunya, Barcelona, Spain, Center for Medical Image Computing, Department of Medical Physics and Biomedical Engineering, NMR Research Unit, Queen Square MS Centre, Department of Neuroinflammation, UCL Queen Square Institute of Neurology, Faculty of Brain Sciences, UCL, London, United Kingdom
View the Poster
A fully automated approach for lumbar plexus segmentation that could facilitate quantitative MRI assessments is presented. The approach is based on a 3D cascaded Convolutional Deep Neural Network (CNN) with concatenated loss function and optimized data augmentation policy. The method offers single modality segmentation and uses as input a commonly used 3D acquisition for peripheral nerve imaging. The performance analysis of the predicted segmentation results in comparison to manually segmented masks revealed 68% agreement. Future improvements in the predictive performance of the proposed method are anticipated by involving much larger datasets to reduce overfitting and improve CNN generalization ability.
|
|
2659.
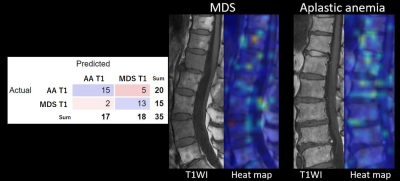 |
External validation of a machine learning algorithm for differentiating between myelodysplastic syndromes and aplastic anemia
Miyuki Takasu1, Takashi Abe2, Shogo Maeda1, Yasutaka Baba1, Yuji Akiyama1, Yuji Takahashi1, Hideaki Kakizawa3, and Kazuo Awai1
1Department of Diagnostic Radiology, Hiroshima University Hospital, Hiroshima, Japan, 2Department of Radiology, Tokushima University, Tokushima, Japan, 3Department of Radiology, Hiroshima Red Cross Hospital, Hiroshima, Japan
Poster Permission Withheld
An MRI-based predictive model was built to differentiate between myelodysplastic syndrome (MDS) and aplastic anemia (AA). The conventional multiparametric MRI provided correct diagnosis with a support vector machine model at accuracies up to 78.0% with a combination of age, fat fraction, and platelet count. In an external validation, the LeNet model achieved an accuracy of 80.0%, sensitivity of 80.0%, specificity of 81.7%, and AUC of 0.860 for T1WI and an accuracy of 65.6%, sensitivity of 65.6%, specificity of 65.3%, and AUC of 0.667 for STIR images. The machine learning algorithm proved effective for differentiating MDS from AA.
|
|
2660.
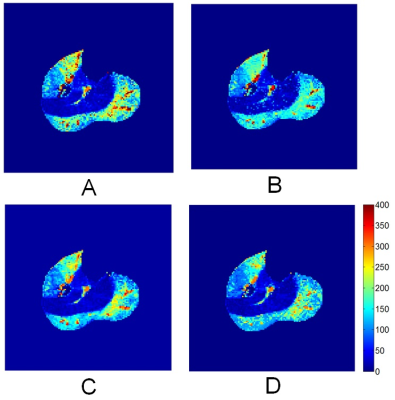 |
Mapping of exercise-stimulated muscle perfusion using DCE-MRI and an artificial neural network approach
Jeff L Zhang1, Christopher C Conlin2, Xiaowan Li2, Gwenael Layec3,4, Ken Chang1, Jayashree Kalpathy-Cramer1,5, and Vivian S Lee6
1A.A. Martinos Center for Biomedical Imaging; Radiology, Massachusetts General Hospital, Charlestown, MA, United States, 2Radiology and Imaging Science, University of Utah, Salt Lake City, UT, United States, 3Department of Kinesiology, University of Massachusetts, Amherst, MA, United States, 4Institute for Applied Life Sciences, University of Massachusetts, Amherst, MA, United States, 5MGH and BWH Center for Clinical Data Science, Massachusetts General Hospital, Charlestown, MA, United States, 6Verily Life Sciences, Cambridge, MA, United States
View the Poster
We tested the feasibility of using artificial neural network (NN) to rapidly map calf-muscle perfusion, and assessed the importance of data diversity in NN training. Forty-eight DCE MRI data were collected from healthy and diseased subjects stimulated by plantar flexion. Results: the NN method was much faster than model fitting. The NN trained with diverse data gave estimates with mean absolute error (MAE) of 15.9 ml/min/100g, significantly more accurate than regular model fitting or NN trained with homogeneous data (MAE 22.3 and 24.9 ml/min/100g, P<0.001). Conclusion: properly trained NN is capable of estimating muscle perfusion with high accuracy and speed.
|
|
2661.
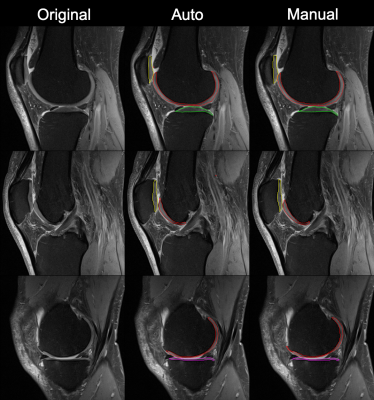 |
Automated Cartilage Segmentation for Clinical Knee MR Images using Transfer Learning
Mingrui Yang1, Ceylan Colak2, Andreas Nanavati1, Sibaji Gaj1, Carl Winalski2, Naveen Subhas2, and Xiaojuan Li1
1Program of Advanced Musculoskeletal Imaging, Cleveland Clinic, Cleveland, OH, United States, 2Radiology, Cleveland Clinic, Cleveland, OH, United States
View the Poster
Laborious and time-consuming manual or semi-automatic cartilage and meniscus segmentation, which in addition suffers from intra and inter reader variability, has been one of the major hurdles of developing and applying techniques such as quantitative magnetic resonance imaging in routine clinical practice for improved osteoarthritis patient treatment and management plans. In addition, effective and robust deep learning based automatic cartilage and meniscus segmentation models are still lacking in heterogenous clinical settings. The purpose of this study is to assess the feasibility of building an automatic cartilage segmentation model using transfer learning with limited and heterogenous clinical MR scans.
|
|
2662.
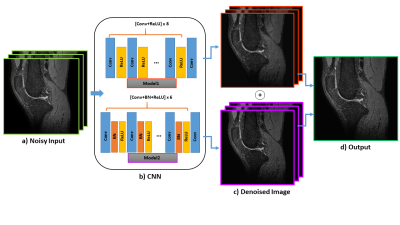 |
Noise Reduction of 3D Fast Spin Echo Knee Imaging Using Fusion of Convolutional Neural Networks
Shutian Zhao1, Siyue Li1, Xiaorui Xu1, Chun Ki Franklin Au1, Huimin Zhang1, and Weitian Chen1
1CUHK lab of AI in radiology (CLAIR), Department of Imaging and Interventional Radiology, The Chinese University of Hong Kong, Hong Kong, Hong Kong
View the Poster
3D FSE can be acquired with isotropic resolution and reformatted into an arbitrary plane for visualizing complex anatomic structures. However, image blurring can occur on short T2 tissues when long echo trains are used. Image deblurring may result in loss of signal-to-noise ratio (SNR). In this work, we proposed a fusion network based on CNNs to address this problem. We demonstrate this network has potential to suppress the noise and reserve structure details at the same time.
|
|
2663.
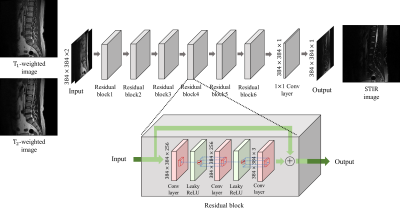 |
Are Two MR Images Enough to Generate the Third One Accurately? - Clinically Feasible Fat Suppression of Lumbar Spine MRI from T1w and T2w Images Only
Sewon Kim1, Hanbyol Jang1, Kyungwon Kim1, Hyeon Gyu Kim1, Young Han Lee2, Sungjun Kim*2,3, and Dosik Hwang*1
1Yonsei University, Seoul, Republic of Korea, 2Yonsei University College of Medicine, Seoul, Republic of Korea, 3Gangnam Severance Hospital, Seoul, Republic of Korea
Watch the Video
This study aims to generate T2-weighted fat suppression (T2 FS) magnetic resonance (MR) lumbar spine image from T1-weighted (T1-w) and T2-weighted (T2-w) images using fully convolutional neural networks. We trained our model that uses multimodal images (T1-w, and T2-w images) to generate synthetic T2FS images close to the acquired T2FS images. The results of our study show that our deep learning model can properly generate bone marrow edema of the vertebral bodies which significantly impact on diagnosis.
|
|
2664.
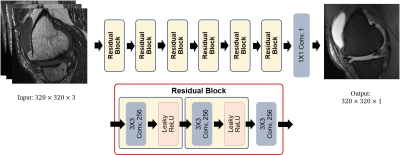 |
Knee MR Image Translation from Multi Contrast Images for Fat Suppression via Deep Learning with Perceptual Loss
Sewon Kim1, Hanbyol Jang1, Kaustubh Lall2, Armin Jamshidi2, Sheronda Statum2,3, Christine B. Chung2,3, Won C. Bae*2,3, and Dosik Hwang*1
1Yonsei University, Seoul, Republic of Korea, 2University of California-San Diego, San Diego, CA, United States, 3VA San Diego Healthcare System, San Diego, CA, United States
View the Poster
The goal of this study is to generate PD Fat Suppression (PD FS) knee magnetic resonance (MR) image using deep neural network with three multi-contrast MR images (T1-weighted, T2-weighted, PD-weighted images). The results of our study show that our deep learning model can learn the relations between the input multi-contrast images and PD FS image and demonstrate the feasibility of deep learning can generate the features which have an effect on diagnosis.
|
|
Back to Top |
|
2665.
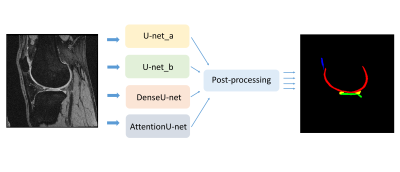 |
Fully Automatic Multi-label Segmentation of Knee joint MRI using Deep Learning Neural Networks
Siyue Li1, Xiaorui Xu1, Chun Ki Franklin Au2, and Weitian Chen1
1CUHK lab of AI in radiology (CLAIR), Department of imaging and interventional radiology , The Chinese university of Hong Kong, HongKong, Hong Kong, 2Department of imaging and interventional radiology, The Chinese university of Hong Kong, Hong Kong, Hong Kong
View the Poster
Accurate segmentation of the cartilage and meniscus is highly desirable for diagnosis and treatment of knee joint diseases. We implemented and compared four deep learning neural networks for fully automated simultaneous segmentation of cartilage and meniscus. Using the Osteoarthritis Initiative (OAI) data sets, we demonstrated the U-net combined with specific post-processing achieved the best performance on femoral cartilage, tibial cartilage, patellar cartilage, and meniscus in terms of dice score.
|
|
2666.
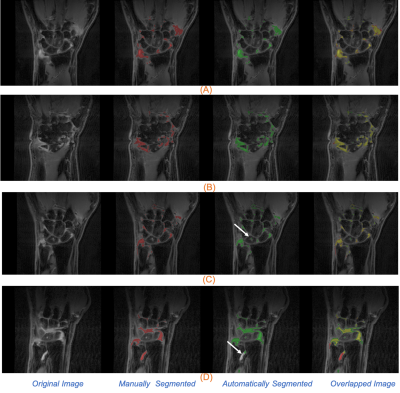 |
Automated Synovitis Segmentation in Patients with Rheumatoid Arthritis
Sibaji Gaj1, Ceylan Colak2, Mingrui Yang1, Kunio Nakamura1, and Xiaojuan Li1
1Department of Biomedical Engineering, Cleveland Clinic, Cleveland, OH, United States, 2Department of Radiology, Cleveland Clinic, Cleveland, OH, United States
View the Poster
Synovitis is a very common finding in joints of RA patients, which may serve as biomarkers for early diagnosis and for early treatment response evaluation. However, synovitis quantification is challenging because manual segmentation of such irregular lesions is tedious and prone to inter reader variation. In this work, we implemented a fully automatic segmentation algorithm for synovitis lesions in wrist Magnetic Resonance images in subjects with RA using deep learning based conditional generative adversarial networks and U-Net. Using a small number of training data, the proposed model demonstrated feasibility of fully automatically synovitis segmentation with reasonable accuracy (Dice coefficient 0.78).
|
|
2667.
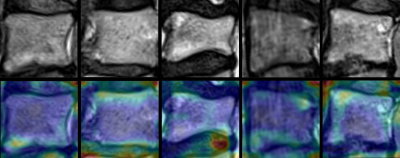 |
Interaction between Modic changes and intervertebral discs revealed using deep learning and attention maps.
Christian Waldenberg1, Hanna Hebelka1, Helena Brisby1, and Kerstin Magdalena Lagerstrand1
1Institute of Clinical Sciences, Sahlgrenska Academy, University of Gothenburg, Gothenburg, Sweden, Gothenburg, Sweden
View the Poster
Observation of crosstalk between inflammatory IVD and vertebral Modic changes (MCs) has been reported. This study aims to further evaluate possible interaction between MCs and intervertebral discs by utilizing a variety of MR image contrasts and visually displaying the relation using attention maps. The attention maps displayed that both the MC in the vertebra and the surrounding tissue, mainly the intervertebral discs, are important for the classifier. This indicates that there is possible interaction between MC and the surrounding intervertebral discs. The choice of contrast in the images used to train the CNN affected the distribution of the attention maps.
|
|
2668.
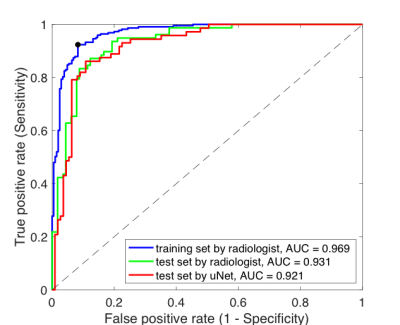 |
Deep learning based fully automated screening pipeline for abnormal bone density using a short lumbar Dixon sequence
Shenglan Chen1, Yinxia Zhao2, Xintao Zhang2, Tianyun Zhao1, Mario Serrano-Sosa1, Xiaodong Zhang2, and Chuan Huang1,3,4
1Biomedical Engineering, Stony Brook University, Stony Brook, NY, United States, 2Radiology, The Third Affiliated Hospital of Southern Medical University (Orthopaedic Hospital of Guangdong Province), Guangzhou, China, 3Radiology, Stony Brook Medicine, Stony Brook, NY, United States, 4Psychiatry, Stony Brook Medicine, Stony Brook, NY, United States
View the Poster
Bone marrow fat fraction (BMFF) has been recognized as one of the quantitative image biomarkers to identify abnormal bone density using modified Dixon sequence. However, this method requires manual segmentation which limits its adoption in clinical practice. In this study, we developed a fully automated radiomics pipeline using deep learning based segmentation and validated its performance comparable to manual segmentation. This finding will facilitate the clinical utility of the entire pipeline as a screening tool for early detection of abnormal bone density.
|
|
2669.
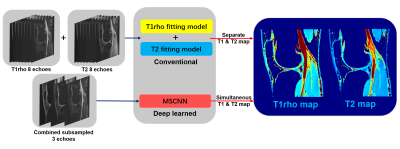 |
Ultra-Fast Simultaneous T1rho and T2 Mapping Using Deep Learning
Hongyu Li1, Mingrui Yang2, Jeehun Kim2, Ruiying Liu1, Chaoyi Zhang1, Peizhou Huang1, Sunil Kumar Gaire1, Dong Liang3, Xiaojuan Li2, and Leslie Ying1
1Department of Biomedical Engineering, Department of Electrical Engineering, The State University of New York at Buffalo, Buffalo, NY, United States, 2Program of Advanced Musculoskeletal Imaging (PAMI), Cleveland Clinic, Cleveland, OH, United States, 3Paul C. Lauterbur Research Center for Biomedical Imaging, Medical AI research center, SIAT, CAS, Shenzhen, China
View the Poster
This abstract presents a deep learning method to generate T1rho and T2 relaxation maps simultaneously within one scan. The method uses 3D deep convolutional neural networks to exploit the nonlinear relationship between and within the combined subsampled T1rho and T2-weighted images and the combined T1rho and T2 maps, bypassing conventional fitting models. Compare with separated trained relaxation maps, this new method also exploits the autocorrelation and cross-correlation between subsampled echoes. Experiments show that the proposed method is capable of generating T1rho and T2 maps simultaneously from only 3 subsampled echoes within one scan with quantification results comparable to reference maps.
|
|
2670.
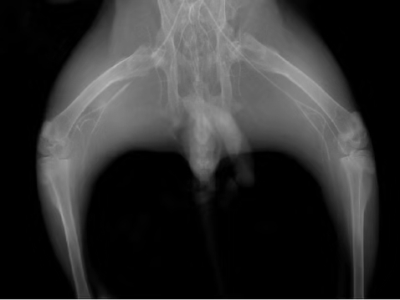 |
Value of texture analysis on the Ktrans map of dynamic contrast enhancement MRI for assessment of critical limb ischemia in diabetic rabbits
Liu Yang1, Yunfei Zha1, and Weiyin Vivian Liu 2
1Renmin Hospital of Wuhan University, WuHan, China, 2GE Healthcare, Beijing, China
Critical limb ischemia (CLI) is a severe complication of diabetes which dramatically increases the risk of limb amputation and mortality. A greater degree of information may be derived by including an assessment of microvascular permeability in proximal femur by the transfer constant (Ktrans) values and MR texture analysis. Twelve diabetic rabbits (6 without CLI, 6 with occluded vasculature of the lower extremities) were examined by dynamic contrast‐enhanced magnetic resonance imaging at fixed time points.We found that Ktrans reached the minimum on one day after ischemia induction, and then recovered along with neoangiogenesis. Three texture features (mean value, MPP, sumAverage) were significantly different between two groups. Ktrans and three TA feature parameters were correlated with capillary density. Overall, Ktrans can be used to quantitative evaluation of changes in femur microvascular permeability in diabetic rabbits with CLI.. Texture analysis can provide more quantification information, which can be more accurately detecting alterations in bone marrow in diabetic rabbits
|
|
2671.
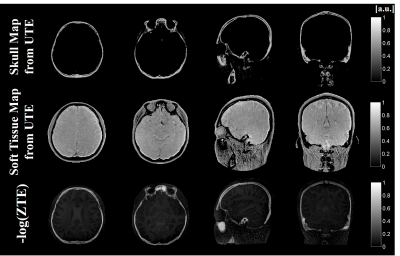 |
Short T2* Components Separation Based on Hyperbolic Tangent R2* Model Using Dual-Echo UTE Imaging
You-Jin Jeong1,2,3, Seung-Nam Baek4,5, Han-Jae Chung1,2,3, Jong-Min Kim1,2,3, Jun-Sik Yoon1,2,3, Chulhyun Lee6, and Chang-Hyun Oh1,2,3,7
1Electronics and Information Engineering, Korea University, Seoul, Korea, Republic of, 2Korea Artificial Organ Center, Korea University, Seoul, Korea, Republic of, 3ICT Convergence Technology for Health and Safety, Korea University, Sejong, Korea, Republic of, 4Medical Image Engineering, Korea University, Sejong, Korea, Republic of, 5GE Healthcare Korea, Seoul, Korea, Republic of, 6Korea Basic Science Institute, Chungcheongbuk-do, Korea, Republic of, 7Corresponding Author, ohch@korea.ac.kr, Korea, Republic of
View the Poster
Ultrashort Echo-Time (UTE) imaging techniques can visualize short T2* components (i.e., bone). Dual-echo UTE imaging was performed with hyperbolic tangent based R2* model to separate the bone and soft tissue. This work demonstrated that cortical bone and bone marrow of skull are well separated with soft tissue using dual-echo UTE images. The proposed method can potentially be used for MR-only transcranial HIFU planning and PET attenuation correction.
|
|
2672.
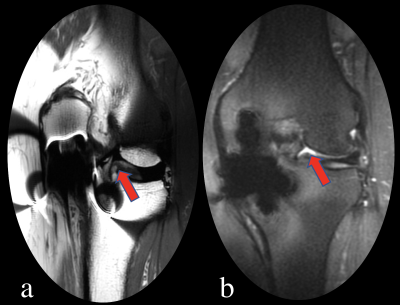 |
The value of MAVRIC-SL technique in evaluation of unicompartment knee arthroplasty (UKA) at 3.0TMR
Qiang Zhao1 and Lizhi Xie2
1Radiology Department, Peking University Third Hospital, Beijing, China, 2GE Healthcare, China, Beijing, China
MAVRIC-SL is a new metal artifact suppression technology that mitigates this type of aliasing by combining the slice-selectivity of SEMAC with the overlapped excitation and combination properties of MAVRIC. In this study, 24 patients with the unicompartment knee arthroplasty (UKA) were performed MAVRIC-SL and conventional sequence scan at 3.0T MR. The effect of MAVRIC-SL on metal artifact subtraction was analyzed, the results show that MAVRIC-SL can significantly improve image quality and reduce image artifacts compared to conventional 2D-FSE.
|
|
2673.
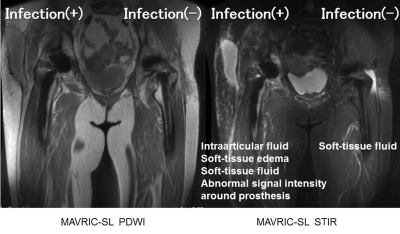 |
Assessment of infection after joint replacement by MAVRIC-SL MR imaging
Tsutomu Inaoka1, Masayuki Sugeta1, Masahiro Sogawa1, Hisanori Tomobe1, Ryosuke Sakai1, Akinori Yamamoto1, Takamistu Uchi1, Sayuri Kato1, Rumiko Ishikawa1, Tomoya Nakatsuka1, Noriko Kitamura1, Shusuke Kasuya1, and Hitoshi Terada1
1Radiology, Toho University Sakura Medical Center, Sakura, Japan
Poster Permission Withheld
MAVRIC-SL sequence can improve the quality of images by decreasing metal artifacts and assess infection around prosthetic implants after joint replacement. Presence of intraarticular fluid is important for the diagnosis of infection after joint replacement. The risk of additional surgical intervention due to infection after joint replacement would be higher when there are more than three of the four findings including intraarticular fluid, soft-tissue edema, soft-tissue fluid, and abnormal signal intensity around prosthesis or both intraarticular fluid and abnormal signal intensity around prosthesis. MAVRIC-SL MR imaging must be a powerful tool for the assessment of infection around prosthetic joint implants.
|
|
2674.
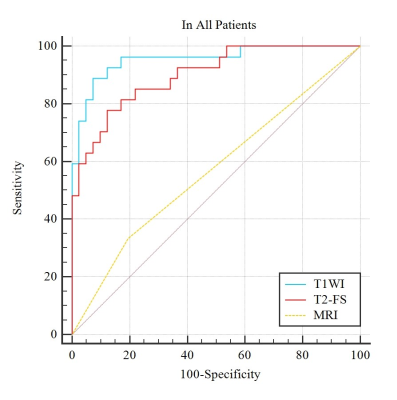 |
Diagnostic value of radiomics model based on non-enhanced MR imaging for differentiation of chondrosarcoma from enchondroma
Jielin Pan1, Yunping Jiang1, Wenjuan Li1, Yijie Fang1, Shaolin Li1, and Guobin Hong1
1the Fifth Affiliated Hospital of Sun Yat-Sen University, Zhuhai, China
View the Poster
Imaging differential diagnosis between Chondrosarcoma and enchondroma is still a challenge because of their similar characteristic. Radiomics1 is a concept that images contain information reflecting underlying pathophysiology and reveal relationship between lesions through quantitative image analyses. Out study aimed to develop radiomics models based on non-enhanced MRI to differentiate chondrosarcoma from enchondroma. Sixty-eight patients were retrospectively studied. The AUC of radiomics model based on TIWI , T2WI-FS were higher than that of conventional MRI (P<0.01, 0.955, 0.901 and 0.569, respectively). Our preliminary study showed radiomics models can be used in differentiation of chondrosarcoma from enchondroma.
|
|
2675.
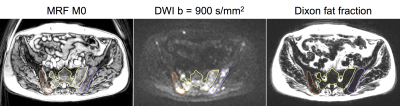 |
Short-term repeatability of magnetic resonance fingerprinting for T1 and T2 measurements of metastatic bone lesions in prostate cancer patients
Matthew R Orton1, Mihaela Rata2, Nina Tunariu2, Andra Curcean2, Julie Hughes2, Erica Scurr2, James D'arcy1, Matthew D Blackledge1, and Dow-Mu Koh2
1Division of Radiotherapy and Imaging, Institute of Cancer Research, Sutton, United Kingdom, 2Department of Radiology, Royal Marsden NHS Foundation Trust, Sutton, United Kingdom
View the Poster
Accurate characterisation of bone metastases and their response to treatment remains an unmet need in oncology. Magnetic resonance fingerprinting (MRF) is an imaging technique that yields inherently co-registered quantitative images from a single acquisition, so this work aims to evaluate measurement repeatability of MRF when measuring T1 and T2 relaxation times in metastatic bone disease. We found good measurement repeatability of 6% for lesion mean T1 and 23% for lesion mean T2.
|
|
2676. |
Survival prediction in patients with osteosarcoma based on MRI Radiomics Features
lu zhang1
1Henan Provincial People's Hospital, zhengzhou, China
View the Poster
The poor 5-year survival rate in high-grade osteosarcoma (HOS) has not been increased significantly over the past 30 years. This work aimed to develop a radiomics nomogram for survival prediction at the time of diagnosis in HOS.
|
|
2677.
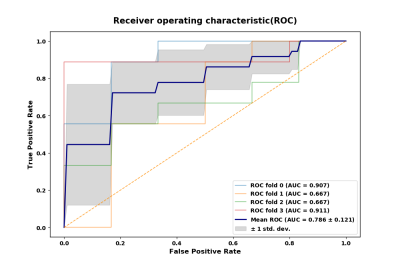 |
Preoperative Pathological Differentiation of Grade 1 and Grade 2/3 Soft Tissue Sarcomas based on Radiomics of ADC maps
Yu Zhang1, Shaowu Wang1, Yuwei Xia2, and Kai Zhang1
1The second hospital of Dalian Medical University, Dalian, China, 2Huiying Medical Technology Inc, Beijing, China
View the Poster
Radiomics based on ADC maps provides a new evaluation method for the preoperative pathological differentiation of Grade 1 and Grade 2/3 soft tissue sarcomas. By comparing the performance of five classifiers (random forests, logistic regression, Multi-Layer Perceptron, k-nearest neighbor, and support vector machine), we found that random forests model achieved the best result (AUC: 0.802 (95% CI: 0.659-0.881), sensitivity:0.722, specificity:0.875) on ADC maps, that can serve as a quantitative tool to differentiation of Grade 1 and Grade 2/3 soft tissue sarcomas. And the radiomics features have the capability in reflecting the Ki67 index
|
|
2678.
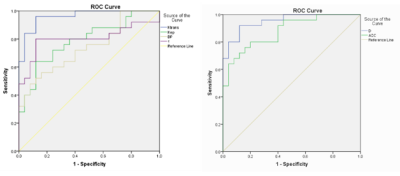 |
A Comparison study between IVIM-DWI and DCE-MRI derived parameters in rabbit VX2 bone tumor model
Tian Yang1, Changjun Liu1, Xiang Feng2, Mengxiao Liu3, Fu He1, Xiaoli Li1, Ruizhi Zhou1, and Wenjian Xu1
1Department of Radiology, the Affiliated Hospital of Qingdao University, Qingdao, China, 2MR Scientific Marketing, Siemens Healthcare, Beijing, China, 3MR Scientific Marketing, Siemens Healthcare, Shanghai, China
View the Poster
The aim of this study was to evaluate the diagnostic efficiency of the parameters of DCE-MRI and IVIM-DWI between solid area of tumor and normal bone marrow in the model of rabbit VX2 bone tumor. The results showed that DCE-DWI and IVIM-DWI were equally important in the differential diagnosis in the solid area of tumor and normal bone marrow.
|
|
2679.
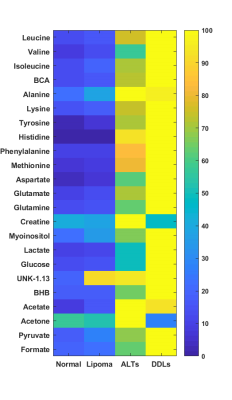 |
Differentiating ALTs/DDLs from benign lipomas using high-resolution 1H MR Spectroscopy
Santosh K Bharti1, Brett A. Shannon2, Raj Kumar Sharma1, Adam Levin2, Carol Morris2, Laura Fayad2,3, and Zaver M. Bhujwalla1,4,5
1Div. of Cancer Imaging Research, The Russell H. Morgan Dept of Radiology and Radiological Science, The Johns Hopkins University School of Medicine,, Baltimore, MD, United States, 2Department of Orthopaedic Surgery, The Johns Hopkins University School of Medicine, Baltimore, MD, United States, 3Musculoskeletal Radiology, The Russell H. Morgan Department of Radiology and Radiological Science, The Johns Hopkins University School of Medicine, Baltimore, MD, United States, 4Sidney Kimmel Comprehensive Cancer Center, The Johns Hopkins University School of Medicine, Baltimore, MD, United States, 53Radiation Oncology and Molecular Radiation Sciences, The Johns Hopkins University School of Medicine, Baltimore, MD, United States
View the Poster
Adipocytic tumors present a spectrum of neoplastic disease including benign lipomas, atypical lipomatous tumors (ALTs), and malignant liposarcomas DDLs. Distinguishing high-grade liposarcomas (DDLs-dedifferentiated liposarcomas) and premalignant ALTs from benign lipomas can be a diagnostic challenge with implications for surgical and clinical management. Our study demonstrate that high-resolution 1H magnetic resonance spectroscopy (MRS) may be used as an additional method for classification and differential diagnosis of ALTs from benign lipomas. Metabolic analysis clearly reflects the metabolic changes associated with malignant progression.
|
|
Back to Top |
|
2680.
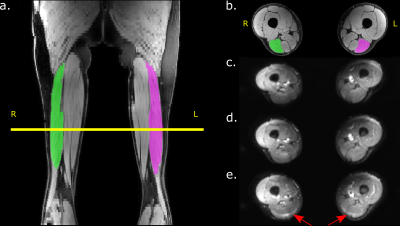 |
A 64-channel receive coil for accelerated diffusion imaging of the upper legs
Susanne S. Rauh1, Melissa T. Hooijmans1, Lars Bannink2, Laura Secondulfo1, Martijn Froeling3, Aart J. Nederveen2, and Gustav J. Strijkers1
1Department of Biomedical Engineering and Physics, Amsterdam UMC, location AMC, Amsterdam, Netherlands, 2Department of Radiology and Nuclear Medicine, Amsterdam UMC, location AMC, Amsterdam, Netherlands, 3Department of Radiology, Utrecht UMC, Utrecht, Netherlands
Watch the Video
In elite sports, hamstring injuries are very common and show high recurrence rates. Diffusion tensor imaging is a promising technique to obtain detailed information like return to play time about the injury. Due to the requirement of long scan times and high signal to noise ratio (SNR), a 64-channel upper leg receive coil was developed and compared to a conventional coil in healthy volunteers. Both coils show similar B0 offset and variance. The upper leg coil showed an SNR increase of more than 45% in the biceps femoris long head muscle. This allows for further acceleration of the sequence.
|
|
2681.
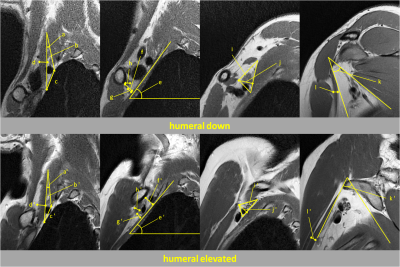 |
Evaluation of Kinematics and Image Quality of Thoracic Outlet during Humeral Elevation in Normal Volunteers Using 3D-MRI with Compressed Sensing
Manabu Arai1,2, Taiki Nozaki2, Saya Horiuchi2, Takahiro Sato2, Miki Hirano2, Shigekazu Funada2, Takeshi Hara3, Atsushi Tasaki4, Nobuto Kitamura4, Masahiro Jinzaki1, and Yasuyuki Kurihara2
1Department of Radiology, Keio University School of Medicine, Tokyo, Japan, 2Department of Radiology, St. Luke's International Hospital, Tokyo, Japan, 3Department of Intelligent Image Information, Gifu University School of Medicine, Gifu, Japan, 4Department of Orthopaedic Surgery, St. Luke's International Hospital, Tokyo, Japan
View the Poster
We performed 3D-MR imaging with compressed sensing on the thoracic outlet of 23 normal volunteers, comparing it with conventional 2D-MR imaging. The image quality score of 3D-PDWI with compressed sensing was significantly lower than that of 2D-PDWI. However, no significant difference in the image quality score of MRA with compressed sensing due to arm position was demonstrated. Subclavian arterial stenosis during humeral elevation was shown in 30.4% of asymptomatic subjects without gender predominance. 3D-MR imaging using compressed sensing enables us to evaluate the kinematics of the thoracic outlet, and may contribute to the diagnosis of TOS in clinical settings.
|
|
2682.
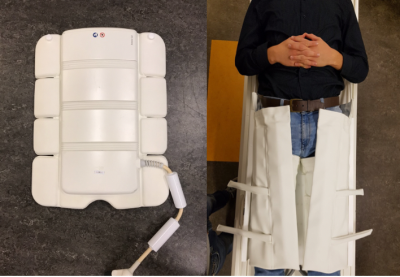 |
Validation of a novel 64-channel coil for clinical high-resolution T2-weighted imaging of the upper leg
Lars S. Bannink1,2, Susanne S. Rauh3, Melissa T. Hooijmans3, Robert Hemke1, Frank F. Smithuis1,4, J. Michiel Den Harder1, Josien C. Douw - van den Noort1, Johannes L. Tol4, Frank F.J. Simonis5, Aart J. Nederveen1, Gustav J. Strijkers3, and Mario Maas1,4
1Department of Radiology and Nuclear Medicine, Amsterdam UMC, location AMC, Amsterdam, Netherlands, 2University of Twente, Enschede, Netherlands, 3Department of Biomedical Engineering and Physics, Amsterdam UMC, location AMC, Amsterdam, Netherlands, 4Academic Center for Evidence-based Sports medicine (ACES), Amsterdam UMC, location, Amsterdam, Netherlands, 5Magnetic Detection and Imaging (MD&I), University of Twente, Enschede, Netherlands
View the Poster
Hamstring strains are the most frequent injuries in elite football. Currently, it is not possible to accurately predict recovery or recurrence risk, which motivates the development of improved leg muscle imaging methods and hardware. A novel 64-channel upper leg coil was designed and compared to a conventional coil in healthy volunteers using two clinical high-resolution muscle T2-weighted sequences on 3T. The visibility of the anatomy and image quality were comparable to a conventional coil and scored clinically acceptable by experienced radiologists. The novel coil displayed an increased SNR of 31% which could be exploited to accelerate the imaging protocols.
|
|
2683.
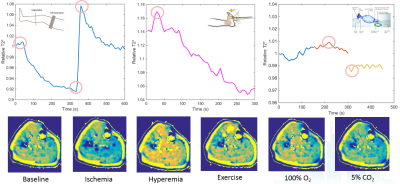 |
Evaluation of Three Different Paradigms for Provoking Blood Oxygenation Level-Dependent MRI Signal Changes in Skeletal Muscles
Shiteng Suo1, Hui Tang1, Qing Lu1, and Jianrong Xu1
1Renji Hospital, School of Medicine, Shanghai Jiao Tong University, Shanghai, China
Poster Permission Withheld
Stress BOLD MRI allows for quantitative evaluation of blood flow reserve in skeletal muscles. In this study, we conducted a head-to-head comparison between three commonly used stressors including gas inhalation, cuff compression induced ischemia and postocclusive reactive hyperemia, and exercise. Results showed that different paradigms could produce different BOLD signal variation profiles. Both correlation and discrepancy can be noted regarding to the relationship between BOLD MRI and TcPO2 measurements. Ischemia and reactive hyperemia paradigm had better interobserver/interscan reproducibility, exercise had larger signal alterations, and both can reveal the age-dependent differences in BOLD signal and may be preferred in further applications.
|
|
2684. |
Characterization of the Microenvironment of the Intervertebral Disc in Patients with Chronic Low Back Pain by Z-spectral imaging
Li Li1, Jicheng Fang1, Alessandro Scotti2, Mehran Shaghaghi2, Wenzhen Zhu1, and Kejia Cai2
1Radiology, Tongji Hospital, Tongji Medical College,HUST, Wuhan, China, Wuhan, China, 2Radiology, 1.Radiology Dept., 2. Bioengineering Dept., College of Medicine, University of Illinois at Chicago, Chicago, IL, United States, Chicago, IL, United States
Poster Permission Withheld
To assess the microenvironment changes of intervertebral disc degeneration, ZSI data were collected from the lumbar spine of 26 patients with CLBP and 21 AC and produced maps of NOE, Water, GAG, APT, MTC. By comparing such parameters between the two groups, it was revealed that the microenvironment of the discs in AC group was different from the discs in the CLBP group. And, the microenvironment of the discs in the sore and non-sore subgroup was also different. ZSI is a noninvasive technique that can be used to test the changes of the microenvironment of the intervertebral discs.
|
|
2685.
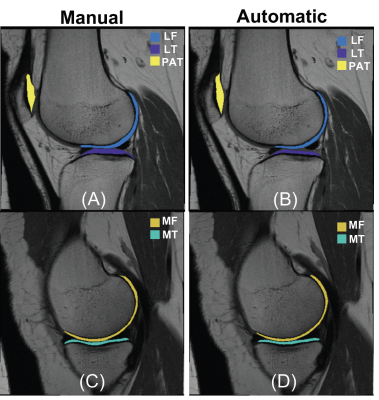 |
T2 Analysis of the Entire Osteoarthritis Initiative Dataset (N=25,729)
Alaleh Razmjoo1, Francesco Caliva1, Jinhee Lee1, Felix Liu2, Gabby B. Joseph1, Thomas M. Link 1, Sharmila Majumdar 1,3, and Valentina Pedoia 1,3
1Department of Radiology and Biomedical Imaging, University of California, San Francisco, San Francisco, CA, United States, 2Department of Epidemiology and Biostatistics, University of California, San Francisco, San Francisco, CA, United States, 3Center of Digital Health Innovation (CDHI), University of California, San Francisco, San Francisco, CA, United States
Watch the Video
Cartilage T2 relaxometry values are previously shown to be correlated to incidence OA, however prognostic ability of T2 is not yet established. In this study, an automatic deep learning method is built using 3921 manually segmented images and T2 was evaluated on entire Osteoarthritis Initiative Dataset (N=25,729). The proposed automatic T2 quantification was shown to be interchangeable with human process and significant association between elevated T2 and future incidence of OA was observed. The results of this study prove the prognostic ability of this compositional MRI technique on the larger sample ever analyzed.
|
|
2686.
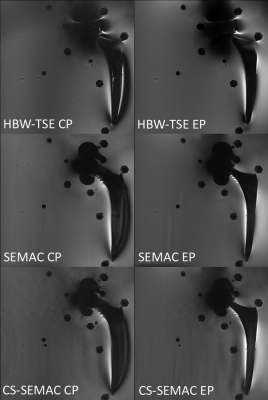 |
Optimizing Metal Related Artifact Reduction MRI at 3T: Circular versus Elliptical Radiofrequency Pulse Polarization
Iman Khodarahmi1, John Kirsch2, Gregory Chang1, and Jan Fritz3
1New York University School of Medicine, New York, NY, United States, 2Massachusetts General Hospital, Boston, MA, United States, 3Johns Hopkins Hospital, Baltimore, MD, United States
Video Permission Withheld
Clinical metal artifact reduction techniques address B0 inhomogeneity-induced artifacts; whereas other source of artifacts, such as B1 inhomogeneity remain unaddressed. However, B1 inhomogeneity can be reduced by implementing an elliptical polarization of the radiofrequency pulse. In an in-vitro setup of hip arthroplasty implants, we show that MRI with elliptical pulse polarization results in stronger metal artifact reduction and overall superior image quality than circular polarization without increase in SAR values.
|
|
2687.
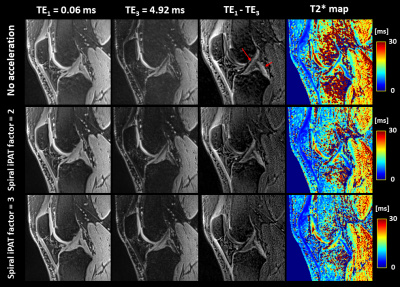 |
Short T2* quantification of knee structures based on accelerated UTE Spiral VIBE MRI with SPIRiT reconstruction
Céline Smekens1, Floris Vanhevel2, Ben Jeurissen3, Pieter Van Dyck2, Jan Sijbers3, and Thomas Janssens1
1Siemens Healthcare NV/SA, Beersel, Belgium, 2Department of Radiology, Antwerp University Hospital and University of Antwerp, Edegem, Belgium, 3imec-Vision Lab, Department of Physics, University of Antwerp, Wilrijk, Belgium
View the Poster
Clinical validation of quantitative UTE MRI techniques in musculoskeletal studies remains limited, despite their potential to unveil short T2* information. As these techniques require considerably more scan time than conventional imaging, there is a need for integration of acceleration methods. This study investigated the use of the UTE Spiral VIBE sequence with in-plane acceleration and SPIRiT reconstruction for short T2* mapping of knee structures. As similar image quality and T2* values were obtained for the non-accelerated and accelerated acquisitions, the UTE Spiral VIBE technique shows great promise for fast UTE T2* MRI of the knee.
|
|
2688.
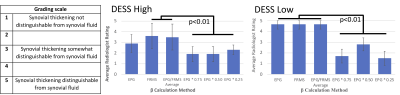 |
Ideal Weighting Parameter for Non-Contrast Detection of Synovitis Using Diffusion-Weighted DESS
Jacob Thoenen1, James W MacKay2, Akshay Chaudhari1, Lauren E Watkins1, Emily McWalter3, Brian Hargreaves1, Feliks Kogan1, and Garry E Gold1
1Radiology, Stanford University, Stanford, CA, United States, 2Department of Radiology, University of Cambridge, Cambridge, United Kingdom, 3Department of Mechanical Engineering, University of Saskatchewan, Saskatoon, SK, Canada
Watch the Video
Non-contrast diffusion-weighted double echo steady state (DESS) imaging has been proposed as an alternative to T1-weighted contrast-enhanced MRI for the evaluation of synovitis. We investigated various weighting values (β) for hybrid image creation from the weighted difference between echos of the DESS sequence in order to null joint fluid and enhance visualization of the synovium. We found that the extended phase graph (EPG) model provided the best β for low diffusion weighted DESS and fluid ROI mean suppression (FRMS) provided the best β for high diffusion DESS. Both methods showed potential for non-contrast evaluation of synovitis for routine knee scanning.
|
|
2689.
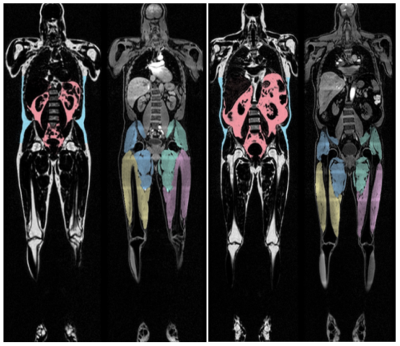 |
Whole Body Composition in Patients Living with HIV
Ravi Chachad1, Gabby B Joseph1, Jiamin Zhou1, Phyllis Tien2,3, Thomas M Link1, Galateia Kazakia1, and Roland Krug1
1Department of Radiology and Biomedical Imaging, University of California San Francisco, San Francisco, CA, United States, 2Department of Medicine, University of California San Francisco, San Francisco, CA, United States, 3Department of Veterans Affairs, University of California San Francisco, San Francisco, CA, United States
Watch the Video
HIV infection is associated with loss of subcutaneous adipose tissue, and the initiation of effective antiretroviral therapy has been associated with increased visceral adipose tissue (VAT). To quantify these changes in the body, MRI was used to image and conduct whole-body composition analysis. VAT was significantly higher in men with HIV infection than control males; there was little difference in females. In general, VAT ratio, the ratio of VAT to subcutaneous adipose tissue, was higher in patients living with HIV (PLWH) than controls. MRI is a quick, radiation-free alternative to DXA and CT in measuring whole-body composition in PLWH.
|
|
2690.
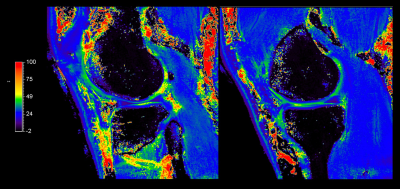 |
Accelerated high-resolution (0.5mm2) T1ρ mapping of the knee joint using interleaved spin-lock-prepared 3D gradient echo with Compressed SENSE
Keita Nagawa1, Suzuki Masashi1, Masami Yoneyama2, Kaiji Inoue1, Eito Kozawa1, and Mamoru Niitsu1
1Saitama Medical University, Saitama, Japan, 2Philips Japan, Tokyo, Japan
View the Poster
3D T1ρ mapping sequence allows rapid acquisition of the entire volume data of each anatomic region, including major joint tissues. In this work, we propose the accelerated high-resolution 3D T1ρ-mapping of the knee-joint using motion-robust interleaved spin-lock acquisition with Compressed SENSE. We compared the T1p mapping with in-plane resolution of 0.5mm2 with different C-SENSE reduction factors (3 and 4.2). There are no clear differences between two image datasets, hence we applied C-SENSE reduction factor of 4.2 (5min48s) for clinical scans, acquiring motion-insensitive high-quality isotropic images less than 6 minutes.
|
|
2691.
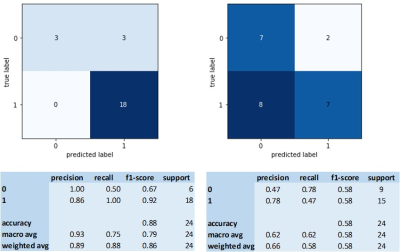 |
Classification of annular fissures and pain-positive discograms using multiple MRI-features with Machine Learning
Kerstin Lagerstrand1,2, Hanna Hebelka1,3, Leif Thorén1,3, Christian Waldenberg1,2, and Helena Brisby1,4
1Institute of Clinical Sciences, Gothenburg University, Gothenburg, Sweden, 2Medical Physics and Biomedical Engineering, Sahlgrenska University Hospital, Gothenburg, Sweden, 3Radiology, Sahlgrenska University Hospital, Gothenburg, Sweden, 4Orthopaedics, Sahlgrenska University Hospital, Gothenburg, Sweden
Poster Permission Withheld
Imaging-based features are needed to improve the characterization of degenerative IVD-changes and possibility of finding a linkage between features and pain.
Multiple T2w-imaging-features and Machine-Learning was used for classification of fissures involving outer annulus and for pain-positive discograms.
Fissures were classified with high accuracy/precision using regional/heterogeneity features with/without axial loading of the spine. For pain-positive discograms, a larger number of such MRI-features contributed to the classification.
Findings suggest that multiple MRI-features, extracted from T2w-imaging, improve the classifications, and that regional/heterogeneity features extracted with both conventional imaging with the spine unloaded and with axial loading of the spine are of importance.
|
|
2692.
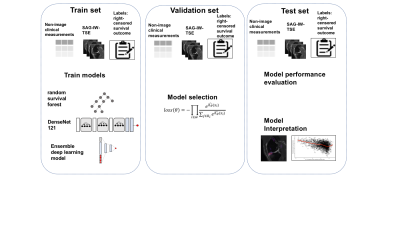 |
Discovering MRI Imaging Biomarkers Associated with Progression of Knee Pain Using Deep Learning for Survival Analysis
Jinhee Jenny Lee1, Chul Young Chung2, Felix Liu3, Sharmila Majumdar1, and Valentina Pedoia1
1Department of Radiology and Biomedical Engineering, UCSF, San Francisco, CA, United States, 2Bay Imaging Consultants, Walnut Creek, CA, United States, 3Department of Epidemiology and Biostatistics, UCSF, San Francisco, CA, United States
View the Poster
Medical attention for knee osteoarthritis (OA) is currently focused on symptomatic pain management in clinical setting. Associating future knee OA pain development with baseline characteristics of knee OA cohort is important in understanding of disease prognosis and designing treatment strategies. We built a predictive model of knee OA pain-free survival time using baseline MRI and other image-independent clinical measurements.
|
|
2693.
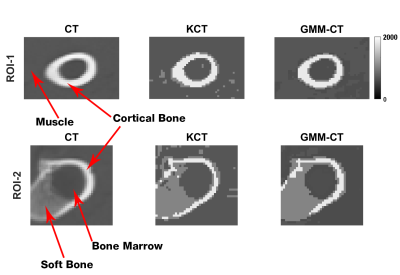 |
PSEUDO-CT IMAGE GENERATION FROM MULTIPLE ECHO TIME 7 TESLA MR DATA VIA GAUSSIAN MIXTURE MODELLING
Shaeez Usman Abdulla1, David Reutens1, and Viktor Vegh1
1University of Queensland, Brisbane, Australia
View the Poster
With the increasing introduction of new PET-MR systems, MRI-based methods for pseudo-CT image generation for PET attenuation correction have to be made widely applicable. Existing methods requiring prior anatomical information for estimating CT values form MR signals have been shown to perform poorly, especially in patients. Ultrashort echo time (UTE) MR data has shown some promise, as it is sensitive to tissue classes captured in CT images. We applied existing MR-based methods and a new Gaussian mixture model approach relying on multi-echo MR magnitude and phase data for pseudo-CT image generation. The Gaussian mixture modelling method outperformed other methods investigated.
|
|
2694.
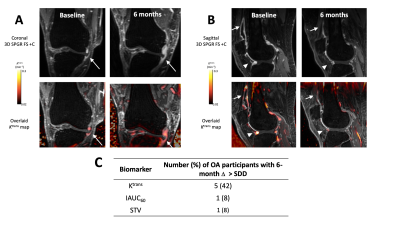 |
Dynamic Contrast Enhanced MR Imaging of Synovitis: Repeatability, Discriminative Validity and Responsiveness in Knee Osteoarthritis
James MacKay1,2, Faezeh Sanaei Nehad3, Josh Kaggie2, Jo Naish3, Caleb Roberts3, Martin Graves2, Fiona Gilbert2, Andrew McCaskie4, John Waterton3,5, Rob Janiczek6, Alexandra Roberts6, and Geoff Parker3
1Radiology, University of East Anglia, Norwich, United Kingdom, 2Radiology, University of Cambridge, Cambridge, United Kingdom, 3BiOxyDyn Ltd, Manchester, United Kingdom, 4Surgery, University of Cambridge, Cambridge, United Kingdom, 5Informatics, Imaging & Data Sciences, University of Manchester, Manchester, United Kingdom, 6Clinical Imaging, GlaxoSmithKline, Stevenage, United Kingdom
Watch the Video
Synovitis is common in knee osteoarthritis (OA) and can be quantified using dynamic contrast-enhanced MR imaging (DCE-MRI). There is a strong rationale for therapeutic targeting of synovitis in OA, and imaging biomarkers derived from DCE-MRI may help evaluate response in clinical trials.
Here we evaluate the test-retest repeatability, ability to discriminate between OA (n = 14) and age-matched controls (n = 6) and 6-month responsiveness to change of three DCE-MRI biomarkers: Ktrans, IAUC60, and synovial tissue volume.
Ktrans demonstrated the best repeatability, discrimination and sensitivity to change suggesting that it is the optimal DCE-MRI biomarker for use in clinical trials.
|
|
2695.
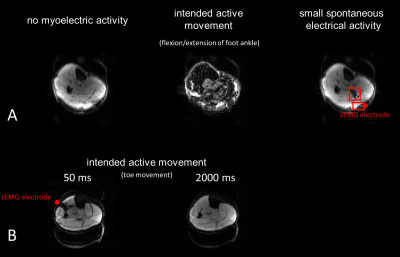 |
Myoelectric Triggered Diffusion-Weighted Imaging for Muscle Contraction Analysis
Martin Schwartz1,2, Petros Martirosian1, Günter Steidle1, Michael Erb3, Bin Yang2, and Fritz Schick1
1Section on Experimental Radiology, University Hospital of Tuebingen, Tuebingen, Germany, 2Institute of Signal Processing and System Theory, University of Stuttgart, Stuttgart, Germany, 3Biomedical Magnetic Resonance, University Hospital of Tuebingen, Tuebingen, Germany
Watch the Video
Retrospective measurements of muscular contraction in diffusion-weighted imaging are inherently asynchronous leading to an unknown time point of acquisition during the muscular motion. Therefore, prospective imaging is investigated based on surface electromyography signals derived during the measurement. Fast and more robust real-time activity detection is achieved by a neural network. Imaging during active muscular contraction can be prevented by analysis of the muscular state; however, sampling at different time points of the muscular contractions is also possible.
|
|