Tuesday Parallel 2 Live Q&A |
Tuesday, 11 August 2020, 13:45 - 14:30 UTC |
Moderators: Christopher Filippi |
Session Number: O-09
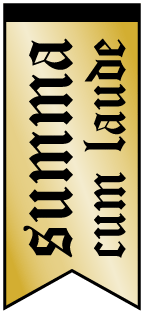 |
0418.
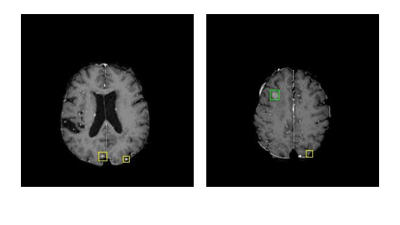 |
An RNN and Autoencoder-based Deep Learning Approach for Detecting Brain Metastases in MRI
Shuyang Zhang1, Min Zhang2, Xinhua Cao3, Geoffrey S Young2, and Xiaoyin Xu2
1University of Michigan-Ann Arbor, Ann Arbor, MI, United States, 2Brigham and Women's Hospital and Harvard Medical School, Boston, MA, United States, 3Boston Children’s Hospital, Boston, MA, United States
Cancer metastases to the brain is a major cause of fatality in patients. Finding all the metastases is crucial to clinical treatment planning as today’s radiation therapy can target up to 20 individual metastases, making it necessary for clinicians to detect and marking multiple metastases in practice. Detecting brain metastases, however, is very challenging because the objects are small and of low contrast. Computer-aided detection of metastases can be highly valuable to improve the accuracy and efficiency of a human reader. In this work, we developed a deep learning-based pipeline for finding metastases on brain MRI.
|
|
0419.
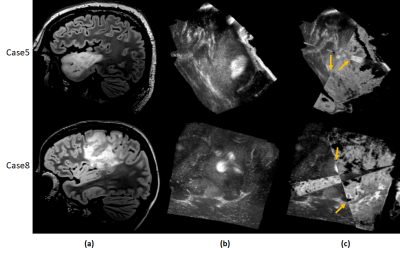 |
Fast multimodal image fusion with deep 3D convolutional networks for neurosurgical guidance – A preliminary study
Jhimli Mitra1, Soumya Ghose1, David Mills1, Lowell Scott Smith1, Sarah Frisken2, Alexandra Golby2, Thomas K. Foo1, and Desmond Teck-Beng Yeo1
1General Electric Research, Niskayuna, NY, United States, 2Brigham and Women's Hospital, Boston, MA, United States
Watch the Video
Multimodality fusion in neurosurgical guidance aids neurosurgeons in making critical clinical decisions regarding safe maximal resection of tumors. It is challenging to have registration methods that automatically update pre-surgical MRI on intra-operative ultrasound, adjusting for the brain-shift for surgical guidance. A 3D deep learning-based convolutional network was developed for fast, multimodal alignment of pre-surgical MRI and intra-operative ultrasound volumes. The neural network is a combination of some well-known deep-learning architectures like FlowNet, Spatial Transformer Networks and UNet to achieve fast alignment of multimodal images. The CuRIOUS 2018 challenge training data was used to evaluate the accuracy of the developed method.
|
|
0420.
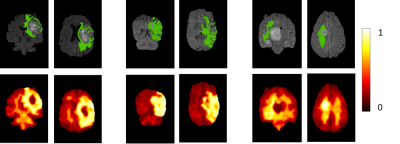 |
A radiomic signature for predicting recurrence of FLAIR abnormality in glioblastomas using multi-modal MRI
Tanay Chougule1, Rakesh Gupta2, Jitender Saini3, Shaleen Agarwal4, Rana Patir4, and Madhura Ingalhalikar1
1Symbiosis Center for Medical Image Analysis, Symbiosis International University, Pune, India, 2Department of Radiology, Fortis Hospital, Gurgaon, India, 3Department of Radiology, National Institute of Mental Health and Neurosciences, Bangalore, India, 4Radiation Oncology and Neurosurgery, Fortis Hospital, Gurgaon, India
Watch the Video
Standard post-operative radiation therapy in glioblastoma delivers radiation uniformly across the hyper-intense areas from pre-operative FLAIR images and does not account for the regions where the infiltration might relapse. This work creates a non-invasive prognostic signature of the extent of recurrent hyper-intense FLAIR using radiomics features extracted from multi-modal MRI. Results demonstrate that the area of recurrence can be accurately predicted earlier with some radiomic features as beacon of recurrence than others when tested temporally across multiple time-points.
|
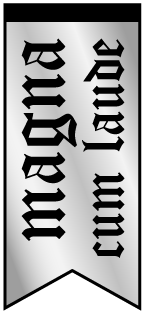 |
0421.
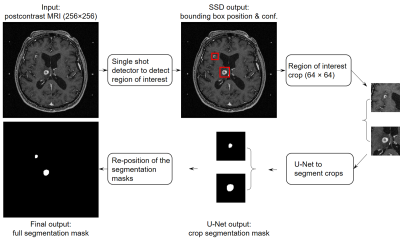 |
Computer-aided detection and segmentation of brain metastases in MRI for stereotactic radiosurgery via a deep learning ensemble
Zijian Zhou1, Jeremiah W. Sanders1, Jason M. Johnson2, Tina M. Briere3, Mark D. Pagel4, Jing Li5, and Jingfei Ma1
1Imaging Physics, The University of Texas MD Anderson Cancer Center, Houston, TX, United States, 2Diagnostic Radiology, The University of Texas MD Anderson Cancer Center, Houston, TX, United States, 3Radiation Physics, The University of Texas MD Anderson Cancer Center, Houston, TX, United States, 4Cancer Systems Imaging, The University of Texas MD Anderson Cancer Center, Houston, TX, United States, 5Radiation Oncology, The University of Texas MD Anderson Cancer Center, Houston, TX, United States
Watch the Video
Manual delineation of brain metastases for stereotactic radiosurgery (SRS) is time consuming and labor intensive. We successfully constructed a deep learning ensemble, including a single shot detector and U-Net, to detect and subsequently segment brain metastases in MRI for SRS treatment planning. Postcontrast 3D T1-weighted gradient echo MR images from 266 patients were randomly split by 212:54 for model training-validation and testing. For the testing group, an overall sensitivity of 80.4% (189/235 metastases) with 4 false positives per patient, and a median segmentation Dice of 77.9% (61.4% - 86.3%) for the detected metastases were achieved.
|
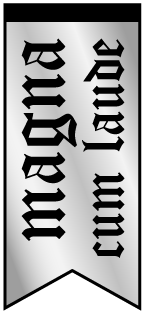 |
0422.
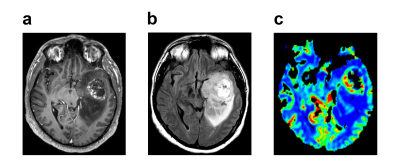 |
Identifying Overall Survival in Glioblastoma Patients Using VASARI Features at 3T
Banu Sacli-Bilmez1, Zeynep Firat2, Melih Topcuoglu2, C. Kaan Yaltirik3, Uğur Türe3, and Esin Ozturk-Isik1
1Institute of Biomedical Engineering, Bogazici University, Istanbul, Turkey, 2Department of Radiology, Yeditepe University, Istanbul, Turkey, 3Department of Neurosurgery, Yeditepe University, Istanbul, Turkey
Watch the Video
Glioblastoma (GBM) is the most common primary brain tumor in adults with 15 months median overall survival. The purpose of this study was to identify overall survival of GBM patients based on clinical and Visually AcceSAble Rembrandt Images (VASARI) features using machine learning. According to our results, a support vector machine (SVM) model worked better for categorical data classification. With the help of adaptive synthetic (ADASYN) oversampling, a fine Gaussian SVM model identified short overall survival at 12 and 24 months thresholds with 99.78% and 88.80% accuracies, respectively.
|
|
0423.
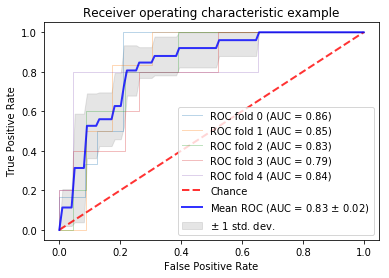 |
Using anatomic and diffusion MRI with deep convolutional neural networks to distinguish treatment-induced injury from recurrent glioblastoma
Julia Cluceru1,2, Paula Alcaide-Leon1, Valentina Pedoia1, Joanna Phillips3, Devika Nair1, Yannet Interian4, Susan Chang5, Javier E. Villanueva-Meyer1, Tracy Luks1, Annette Molinaro5, Mitchel Berger5, and Janine Lupo1,6
1Radiology and Biomedical Imaging, UCSF, San Francisco, CA, United States, 2Bioengineering and Therapeutic Sciences, UCSF, San Francisco, CA, United States, 3UCSF, Neurological Surgery, CA, United States, 4Data Science, USF, San Francisco, CA, United States, 5Neurological Surgery, UCSF, San Francisco, CA, United States, 6Graduate Program in Bioengineering, UCSF/UC Berkeley, San Francisco and Berkeley, CA, United States
Watch the Video
In this study, we leverage a promising new centrally restricted diffusion pattern1 together with modern advances in deep learning to create a novel method for detecting treatment-related injury in the context of suspected recurrent glioblastoma. We report a 5-fold cross-validation average AUC ROC of 0.83 +/- 0.2 for the classification of lesions into two categories: those induced by treatment, and those that are true incidences of recurrent glioblastoma.
|
|
0424.
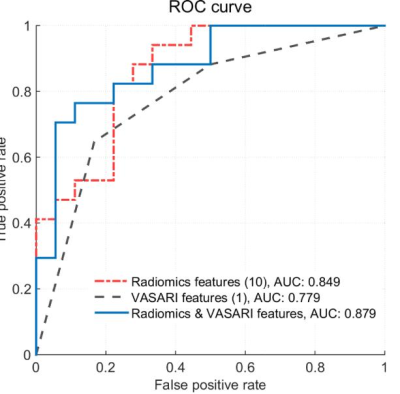 |
IDH1 genotype prediction in lower-grade gliomas: a machine learning study with VASARI and ADC radiomics
Shiteng Suo1, Mengqiu Cao1, Xiaoqing Wang1, Wei Yang2, Jianrong Xu1, and Yan Zhou1
1Renji Hospital, School of Medicine, Shanghai Jiao Tong University, Shanghai, China, 2Guangdong Provincial Key Laboratory of Medical Image Processing, School of Biomedical Engineering, Southern Medical University, Guangzhou, China
Video Permission Withheld
Preoperative noninvasive prediction of IDH mutation status is crucial for prognosis and therapeutic decision making. In this study, we evaluated the qualitative and quantitative MRI features, namely, Visually Accessible Rembrandt Images (VASARI) features and apparent diffusion coefficient radiomics features in identifying IDH1 mutation status in lower-grade gliomas (WHO grade II-III). Results by machine learning methods showed that the combination achieved a better prediction performance. Our model may have the potential to serve as an alternative to the conventional workflow for the noninvasive identification of the molecular profiles.
|
|
0425.
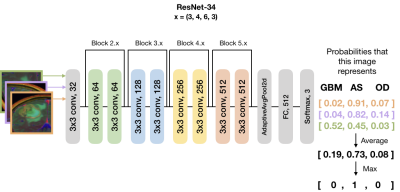 |
Automatic stratification of gliomas into WHO 2016 molecular subtypes using diffusion-weighted imaging and a pre-trained deep neural network
Julia Cluceru1,2, Yannet Interian3, Joanna Phillips4, Devika Nair1, Susan Chang4, Paula Alcaide-Leon1, Javier E. Villanueva-Meyer1, and Janine Lupo1,5
1Radiology and Biomedical Imaging, UCSF, San Francisco, CA, United States, 2Bioengineering and Therapeutic Sciences, UCSF, San Francisco, CA, United States, 3Data Science, USF, San Francisco, CA, United States, 4UCSF, Neurological Surgery, CA, United States, 5Graduate Program in Bioengineering, UCSF/UC Berkeley, San Francisco and Berkeley, CA, United States
Watch the Video
In this abstract, we use diffusion and anatomical MR imaging together with a pre-trained RGB ImageNet to classify patients into major genetic entities defined by the WHO. We achieved 91% accuracy on our validation set with high per-class accuracy, precision, and recall; and 81% accuracy on a separate test dataset.
|
|
0426.
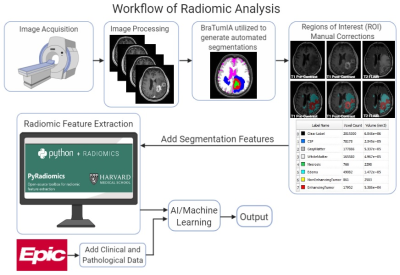 |
MRI-based Radiomics as a Predictive Biomarker of Survival in High Grade Gliomas Treated with Chimeric Antigen Receptor T-Cell Therapy
Sohaib Naim1, Chi Wah Wong2, Eemon Tizpa1, Hannah Jade Young1, Kimberly Jane Bonjoc1, Seth Michael Hilliard1, Aleksandr Filippov 1, Saman Tabassum Khan1, Christine Brown3, Behnam Badie4, and Ammar Ahmed Chaudhry1
1Diagnostic Radiology, City of Hope National Medical Center, Duarte, CA, United States, 2Applied AI and Data Science, City of Hope National Medical Center, Duarte, CA, United States, 3Hematology & Hematopoietic Cell Transplantation and Immuno-Oncology, City of Hope National Medical Center, Duarte, CA, United States, 4Surgery, City of Hope National Medical Center, Duarte, CA, United States
Watch the Video
High grade gliomas (HGG) is the most common malignant primary brain tumors in adults. In this study, 61 patients with recurrent HGGs underwent surgical resection and chimeric antigen receptor-T cell therapy. Volumetric segmentations of contrast-enhanced (CE) and non-enhanced tumors (NET) using T1-weighted CE MR images were used to identify shape- and texture-based features from these regions of interest. We evaluated radiomic characteristics of these HGGs to determine novel imaging biomarkers to predict treatment response. Exponentially-filtered textural radiomic features based on Neighboring Gray Tone Difference Matrix and Gray Level Co-occurrence Matrix derived from NET were the strongest predictors of overall survival.
|
|