Thursday Parallel 2 Live Q&A |
Thursday, 13 August 2020, 15:50 - 16:35 UTC |
Moderators: Christopher Filippi & Yunyan Zhang |
Session Number: O-10
|
1284.
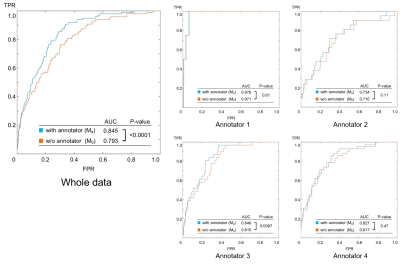 |
The neural network model that can consider the inhomogeneity of the judgements between different annotators: implementation for MRA diagnosis
Yasuhiko Tachibana1, Masataka Nishimori2, Masaaki Chiku3, Naoyuki Kitamura2, Kensuke Umehara4, Junko Ota4, Takayuki Obata1, and Tatsuya Higashi5
1Applied MRI Research, Department of Molecular Imaging and Theranostics, National Institute of Radiological Sciences, QST, Chiba, Japan, 2MNES corporation, Tokyo, Japan, 3Medical Check Studio Ginza Clinic, Tokyo, Japan, 4Medical Informatics Section, QST Hospital, QST, Chiba, Japan, 5Department of Molecular Imaging and Theranostics, National Institute of Radiological Sciences, QST, Chiba, Japan
Watch the Video
The neural network model was designed to judge the existence of aneurysms from brain MR angiography images. On the hypothesis that each radiologist (annotator) has a unique bias for decision, the network was designed so that it accepts input of who the annotator was as an additional information to compute the output. The hypothesis might be reasonable, and the model design might be useful because the accuracy of the trained model (area under the curve (AUC) in receiver operating characteristic (ROC) analysis) elevated significantly (P<.0001, DeLong test) by adding the information of who the annotator was.
|
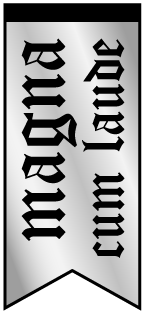 |
1285.
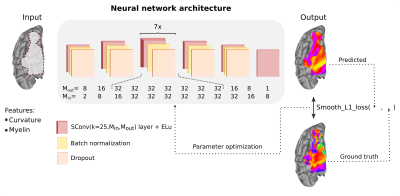 |
Predicting brain function from anatomy with geometric deep learning using high-resolution MRI data
Fernanda Lenita Ribeiro1,2, Steffen Bollmann3, and Alexander M Puckett1,2
1School of Psychology, University of Queensland, Brisbane, Australia, 2Queensland Brain Institute, University of Queensland, Brisbane, Australia, 3Centre for Advanced Imaging, University of Queensland, Brisbane, Australia
Watch the Video
Whether it be in a man-made machine or a biological system, form and function are often directly related. In the latter, however, this particular relationship is often unclear due to the intricate and involved nature of biology. Here we developed a geometric deep learning model capable of exploiting the actual structure of the cortex to learn the complex relationship between brain function and anatomy from structural and functional MRI data. Our model was not only able to predict the functional organization of human visual cortex from anatomical properties alone, but it was also able to predict nuanced variations across individuals.
|
|
1286.
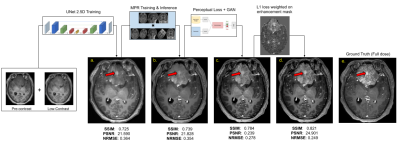 |
Toward a site and scanner-generic deep learning model for reduced gadolinium dose in contrast-enhanced brain MRI
Srivathsa Pasumarthi Venkata1, Jonathan Tamir1, Enhao Gong1, Greg Zaharchuk1, and Tao Zhang1
1Subtle Medical Inc., Menlo Park, CA, United States
Watch the Video
Gadolinium-based contrast agents (GBCAs) create unique image contrast to facilitate identification of various clinical findings. However, recent discovery of gadolinium deposition after contrast-enhanced MRI raises new safety concerns of GBCAs. Deep learning (DL) has recently been used to predict the contrast-enhanced images using only a fraction of the standard dose. However, challenges remain in generalizing the DL methods across different protocols/vendors/institutions. In this work, we propose comprehensive technical solutions to improve DL model robustness and obtain high quality low-dose contrast-enhanced MRI across multiple scanners and institutions.
|
|
1287.
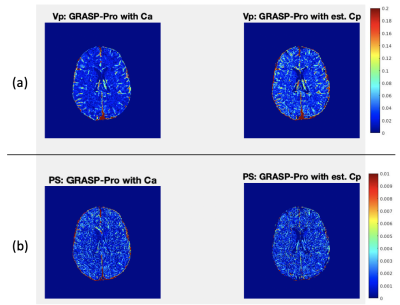 |
Estimating the capillary input function using deep learning approach for Dynamic Contrast-Enhanced MRI assessment of blood brain barrier
Jonghyun Bae1,2, Li Feng3, Krzysztof Geras4, Florian Knoll4, Yulin Ge4,5, and Sungheon Gene Kim4,5
1Sackler Institute of Graduate Biomedical Science,NYU School of Medicine, New York, NY, United States, 2Radiology, Center for Advanced Imaging Innovation and Research, New York, NY, United States, 3Icahn School of Medicine at Mount Sinai, New York, NY, United States, 4Center for Advanced Imaging Innovation and Research, New York, NY, United States, 5Center for Biomedical Imaging, NYU, New York, NY, United States
Watch the Video
This study proposes a deep learning approach of estimating the capillary level of input function for kinetic model analysis on dynamic contrast enhanced (DCE)-MRI data. Our deep-learning network was trained with the numerically synthesized data generated with a wide range of contrast kinetic dynamics with different arterial input function (AIF). We hypothesize that the voxel level capillary input functions would be more accurate input functions for pharmacokinetic analysis. This hypothesis was tested with the DCE-MRI data of healthy subjects.
|
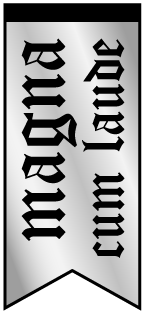 |
1288.
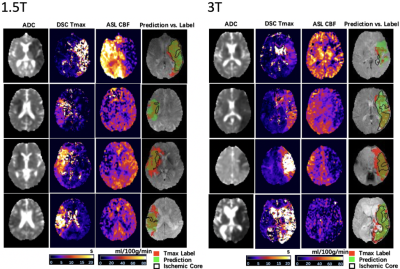 |
Deep Learning Detection of Penumbral Tissue on Arterial Spin Labeling in Stroke
Kai Wang1, Qinyang Shou1, Samantha Ma1, David Liebeskin2, Xin Qiao2, Jeffrey Saver2, Noriko Salamon2, Songlin Yu3, Hosung Kim1, Yannan Yu4, Yuan Xie4, Greg Zaharchuk4, Fabien Scalzo2, and Danny Wang1
1University of Southern California, Los Angeles, CA, United States, 2University of California, Los Angeles, Westwood, CA, United States, 3Beijing Tiantan Hospital, Capital Medical University, Beijing, China, 4Stanford University, Stanford, CA, United States
Watch the Video
A deep learning (DL)-based algorithm was developed to automatically identify the hypoperfusion lesion and penumbra in ASL images of arterial ischemic stroke (AIS) patients. A total of 167 3D pCASL datasets from 137 AIS patients on Siemens MR were used for training, using concurrently acquired DSC MRI as the label. The DL model achieved a voxel-wise area under the curve (AUC) of 0.958, and 92% accuracy for retrospective determination for subject-level endovascular treatment eligibility. The DL-model was cross validated on 12 GE pCASL data with 92% accuracy without fine-tuning of parameters.
|
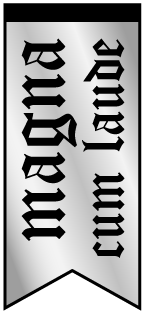 |
1289.
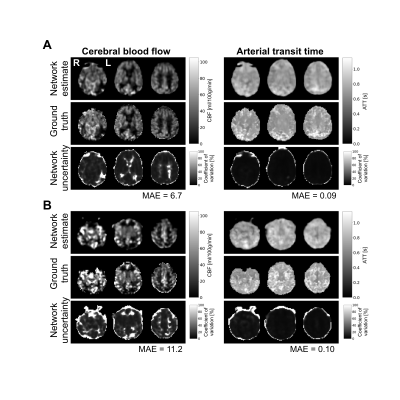 |
A deep learning approach for hemodynamic parameter estimation from multi-delay arterial spin-labelled MRI
Nicholas J. Luciw1,2, Zahra Shirzadi2, Sandra E. Black2, Maged Goubran2, and Bradley J. MacIntosh1,2
1Department of Medical Biophysics, University of Toronto, Toronto, ON, Canada, 2Sunnybrook Research Institute, Toronto, ON, Canada
Watch the Video
Arterial spin-labelled (ASL) MRI is used to quantify cerebral blood flow and arterial transit time. Currently, these parameters are not calculated at the scanner given the time-consuming processing required. Fast, automated parameter estimation is therefore desirable to radiology clinics. Here, we trained a convolutional neural network to estimate cerebral blood flow and arterial transit time from multiple post-label delay ASL. The network produces estimates comparable to other approaches and was designed to evaluate model uncertainty. This fast, automated method is suitable for scan-time generation of accurate hemodynamic maps, important in the assessment of neurological disorders and neurodegeneration.
|
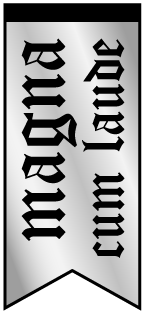 |
1290.
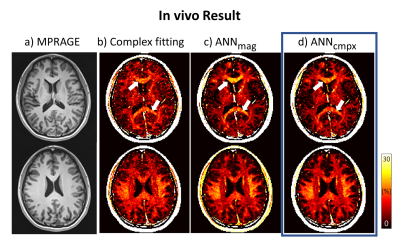 |
Fiber orientation robust myelin water fraction mapping using complex-valued neural network in multi-echo gradient echo
Soozy Jung1, Kanghyun Ryu1, Jae Eun Song1, Mina Park2, and Dong-Hyun Kim1
1Department of Electrical and Electronic Engineering, Yonsei University, Seoul, Korea, Republic of, 2Department of Radiology, Gangnam Severance Hospital, Seoul, Korea, Republic of
Watch the Video
Recently, magnitude-based artificial neural network (ANN) method was implemented to estimate myelin water fraction (MWF) mapping using multi-echo gradient-echo (mGRE) data. However, MWF mapping in mGRE data requires phase information with the demand of considering frequency shifts in white matter. Here, we developed a complex-valued ANN for MWF mapping which could learn the phase information of the mGRE signal. According to simulation and in vivo analysis, complex-valued ANN is more robust to fiber orientation and noise than magnitude-based ANN and conventional fitting method.
|
|
1291.
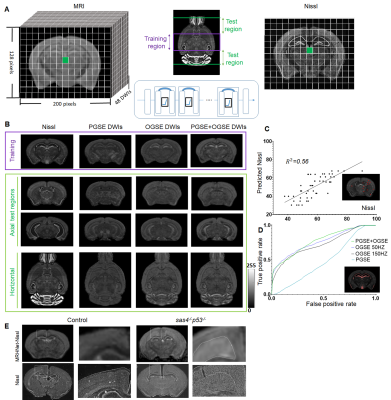 |
Connecting Histology and MRI using Deep Learning
Zifei Liang1, Choong Heon Lee1, Tanzil M. Arefin1, Piotr Walczak2, Song-Hai Shi3, Florian Knoll1, Yulin Ge1, Leslie Ying4, and Jiangyang Zhang1
1Radiology, NYU Langone Health, New York, NY, United States, 2Diagnostic Radiology & Nuclear Medicine, University of Maryland School of Medicine, Baltimore, MD, United States, 3Center for Molecular Imaging & Nanotechnology, Memorial Sloan Kettering Cancer Center, New York, NY, United States, 4Electrical Engineering, University at Buffalo, Buffalo, NY, United States
Watch the Video
We developed a deep learning network that can generate new tissue contrasts from MRI data to match the contrasts of several histological methods. The network was trained using the carefully curated histological data from the Allen Institute mouse brain atlas and co-registered MRI data. In our tests, the new contrasts, which resembled Nissl, neurofilament, and myelin-basic-protein stained histology, demonstrated higher sensitivity and specificity than commonly used diffusion MRI markers to characterize neuronal, axonal, and myelin structures in the mouse brain. The contrasts were further validated using two mouse models with abnormal neuronal structures and dysmyelination.
|
|
1292.
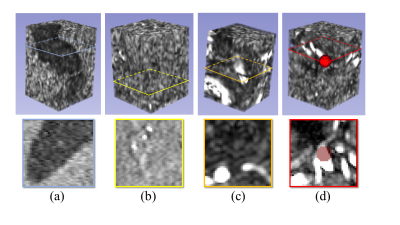 |
The substantial influence of negative sampling and prevalence when presenting classification results: case study with TOF-MRA
Tommaso Di Noto1, Guillaume Marie1, Sebastien Tourbier1, Guillaume Saliou1, Meritxell Bach Cuadra1,2,3, Patric Hagmann1, and Jonas Richiardi1,4
1Faculty of Biology and Medicine, Department of Radiology, Lausanne University Hospital (CHUV) and University of Lausanne (UNIL), Lausanne, Switzerland, 2Medical Image Analysis Laboratory (MIAL), Centre d’Imagerie BioMédicale (CIBM), Lausanne, Switzerland, 3Signal Processing Laboratory (LTS 5), Ecole Polytechnique Fédérale de Lausanne (EPFL), Lausanne, Switzerland, 4Advanced Clinical Imaging Technology, Siemens Healthcare, Lausanne, Switzerland
Watch the Video
One recurrent problem for applying deep learning models in medical imaging is the reduced availability of labelled training data. A common approach is therefore to focus on image patches rather than whole volumes, thus increasing the number of samples. However, for many diseases anomalous patches (positive samples) are outnumbered by negative patches showing no anomaly. Here, we explore different strategies for negative sampling in the context of brain aneurysm detection. We show that classification performances can vary drastically with respect to negative sampling, and that real-world disease or anomaly prevalence can further degrade performance estimates.
|
|
1293.
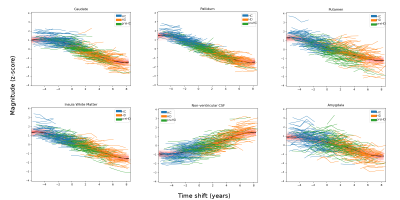 |
Gaussian Process Progression Modelling of structural MRI changes in Huntington’s disease
Peter A. Wijeratne1,2, Sara Garbarino3, Eileanoir B. Johnson2, Sarah Gregory2, Rachael I. Scahill2, Sarah J. Tabrizi2, Marco Lorenzi3, and Daniel C. Alexander1
1Department of Computer Science, University College London, London, United Kingdom, 2Department of Neurodegenerative Disease, University College London, London, United Kingdom, 3Université Côte d’Azur, Valbonne, France
Watch the Video
Longitudinal measurements of brain atrophy using structural T1-weighted MRI (sMRI) can provide powerful biomarkers for clinical trials in neurodegenerative diseases. Here we use the latest advances in disease progression modelling, specifically the Gaussian Process Progression Model (GPPM), to untangle the effects of inter-subject variability, measurement noise and individual disease stage on longitudinal sMRI measurements in Huntington’s disease (HD). We use GPPM to estimate, for the first time, the relative timescale of sub-cortical atrophy in HD, and identify when sMRI provides additional information to genetics. We conclude that GPPM could increase power over standard imaging biomarkers for clinical trials in HD.
|
|