Tuesday Parallel 4 Live Q&A |
Tuesday, 11 August 2020, 14:30 - 15:15 UTC |
Moderators: Jonathan Tamir & Julia Velikina |
Session Number: O-58
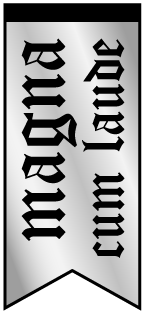 |
0594.
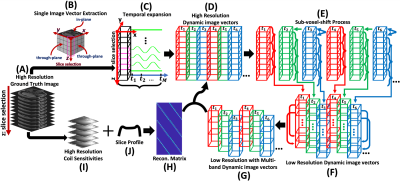 |
Complex-Valued Spatial-Temporal Super-Resolution Combined with Multi-Band Technique on T2*-Weighted Dynamic MRI
Duohua Sun1, Jean-Philippe Galons2, Chidi Ugonna1, Silu Han1, Mahesh Keerthivasan3, Marc Lindley4, and Nan-kuei Chen1
1Biomedical Engineering, The University of Arizona, Tucson, AZ, United States, 2Medical Imaging, The University of Arizona, Tucson, AZ, United States, 3Siemens healthineers, Tucson, AZ, United States, 4GE Healthcare, Waukesha, WI, United States
Watch the Video
We present an approach for improving spatial and temporal resolution of complex-valued T2*-weighted dynamic MRI. Compared with the conventional magnitude-valued super-resolution approaches, our technique utilizes phase information to better recover signal loss caused by susceptibility gradients and generate finer representations of temporal dynamic signal variation. Results from numerical and hybrid simulation show that promising improvements in image resolution, susceptibility artifact reduction and temporal signal variation representation can be achieved using our complex-valued super-resolution MRI scheme when compared to magnitude-valued super-resolution.
|
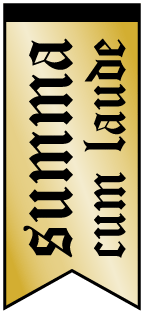 |
0595.
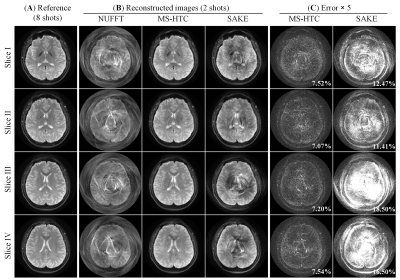 |
Calibrationless Multi-slice Spiral MRI Reconstruction via Low Rank Hankel Tensor Completion
Yilong Liu1,2, Zheyuan Yi1,2,3, Yujiao Zhao1,2, Hua Guo4, and Ed X. Wu1,2
1Laboratory of Biomedical Imaging and Signal Processing, The University of Hong Kong, Hong Kong, China, 2Department of Electrical and Electronic Engineering, The University of Hong Kong, Hong Kong, China, 3Department of Electrical and Electronic Engineering, Southern University of Science and Technology, Shenzhen, China, 4Center for Biomedical Imaging Research, Department of Biomedical Engineering, Tsinghua University, Beijing, China
Watch the Video
This study presents a calibrationless multi-slice spiral MRI reconstruction method based on low rank Hankel tensor completion (MS-HTC). In this study, the sampling pattern of adjacent slices complements each other by using the spiral trajectories of different rotation angles, and MS-HTC exploits the similarities of coil sensitivities, spatial support, and image content. The proposed method was evaluated with human brain spin-echo spiral MR data. The results show that MS-HTC can significantly reduce residual error compared to single-slice reconstruction with simultaneous autocalibrating and k-space estimation (SAKE).
|
|
0596.
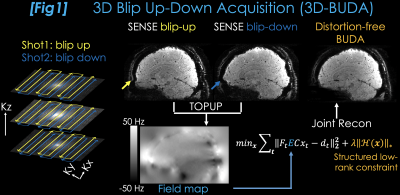 |
3D-BUDA Enables Rapid Distortion-Free QSM Acquisition
Berkin Bilgic1, Benedikt A Poser2, Christian Langkammer3, Kawin Setsompop1, and Congyu Liao1
1Martinos Center for Biomedical Imaging, Charlestown, MA, United States, 2Maastricht University, Maastricht, Netherlands, 3Department of Neurology, Medical University of Graz, Graz, Austria
Watch the Video
We introduce 3D blip-up and -down acquisition (3D-BUDA) for 3D echo planar imaging (3D-EPI). We acquire two-shots of 3D-EPI with alternating phase-encoding to estimate B0 information. Incorporating this into the joint reconstruction of the shots eliminates distortion and enables signal averaging, permitting a 22-second, high-SNR acquisition at 1 mm3 resolution. While shifted sampling between the shots provides complementary k-space coverage, using low-rank regularization eliminates shot-to-shot variations. SNR gain of 7T allows for additional partition acceleration, enabling a 9-second whole-brain scan at Rinplane×Rz=5×2. These are combined with a self-supervised dipole inversion algorithm for Quantitative Susceptibility Mapping (QSM) which outperforms state-of-the-art reconstructions.
|
|
0597.
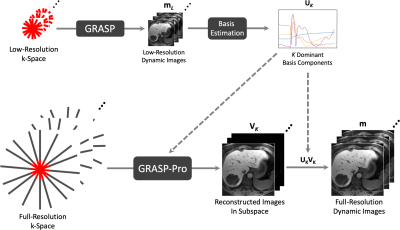 |
High Spatiotemporal Resolution Motion-Resolved MRI using XD-GRASP-Pro
Li Feng1 and Fang Liu2,3
1Biomedical Engineering and Imaging Institute and Department of Radiology, Icahn School of Medicine at Mount Sinai, New York, NY, United States, 2Gordon Center for Medical Imaging, Massachusetts General Hospital, Harvard Medical School, Boston, MA, United States, 3Department of Radiology, University of Wisconsin Madison, Madison, WI, United States
Watch the Video
This work presents a free-breathing motion-resolved golden-angle image reconstruction method called XD-GRASP-Pro, which extends the original XD-GRASP (eXtra-Dimensional Golden-angle RAdial Sparse Parallel MRI) method with imProved reconstruction performance through an additional self-estimated/calibrated low-rank subspace-constraint. The temporal basis used to construct the subspace is estimated from an intermediate reconstruction step on the low-resolution portion of radial k-space, which eliminates the need of using auxiliary data or a physical signal model that is not always available. XD-GRASP-Pro were tested for high spatiotemporal resolution motion-resolved liver MRI.
|
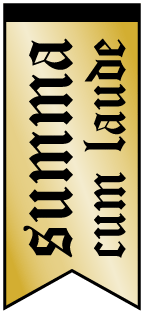 |
0598.
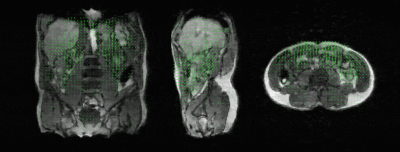 |
Real-time 3D respiratory motion estimation for MR-guided radiotherapy using low-rank MR-MOTUS
Niek R.F. Huttinga1,2, Tom Bruijnen1,2, Cornelis A.T. van den Berg1,2, and Alessandro Sbrizzi1,2
1Computational Imaging Group for MR diagnostics and Therapy, Center for Image Sciences, University Medical Center Utrecht, Utrecht, Netherlands, 2Department of Radiology, Division of Imaging and Oncology, University Medical Center Utrecht, Utrecht, Netherlands
Watch the Video
We propose low-rank MR-MOTUS, a framework for real-time reconstructions of 3D respiratory motion-fields for MR-guided radiotherapy. Low-rank MR-MOTUS factorizes space-time motion-fields into static spatial components and dynamic temporal components. This allows to 1) exploit spatial and temporal correlations in motion, and 2) split the reconstruction into a large-scale off-line training phase, and a small-scale on-line inference phase. Results show that in the on-line inference phase 3D respiratory motion can be estimated in 130ms, from data acquired in 24ms. This yields a total latency of 154ms, and low-rank MR-MOTUS thereby paves the way for real-time MR-guided radiotherapy on the MR-linac.
|
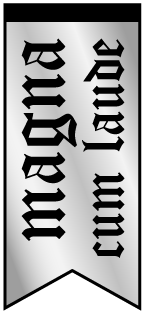 |
0599.
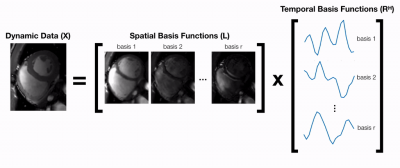 |
Deep subspace learning: Enhancing speed and scalability of deep learning-based reconstruction of dynamic imaging data
Christopher M. Sandino1, Frank Ong1, and Shreyas S. Vasanawala2
1Department of Electrical Engineering, Stanford University, Stanford, CA, United States, 2Department of Radiology, Stanford University, Stanford, CA, United States
Watch the Video
Unrolled neural networks (UNNs) have surpassed state-of-the-art methods for dynamic MR image reconstruction from undersampled k-space measurements. However, 3D UNNs suffer from high computational complexity and memory demands, which limit applicability to large-scale reconstruction problems. Previously, subspace learning methods have leveraged low-rank tensor models to reduce their memory footprint by reconstructing simpler spatial and temporal basis functions. Here, a deep subspace learning reconstruction (DSLR) framework is proposed to learn iterative procedures for estimating these basis functions. As proof of concept, we train DSLR to reconstruct undersampled cardiac cine data with 5X faster reconstruction time than a standard 3D UNN.
|
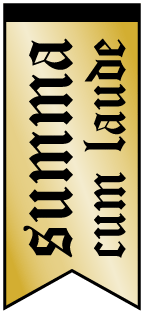 |
0600.
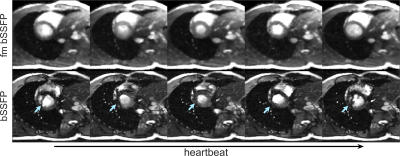 |
The total ellipse of the heart: Cardiac CINE imaging using frequency-modulated bSSFP and the elliptical signal model
Anne Slawig1 and Herbert Köstler1
1Departement of Diagnostic and Interventional Radiology, University Hospital Würzburg, Würzburg, Germany
Watch the Video
Balanced steady state free precession sequences are well suited for cardiac imaging as they are fast, yield high signal and provide excellent contrast between blood and myocardium. To avoid the typical banding artifacts in such sequences, conventionally, multiple phase-cycled acquisitions are performed and combined to one image. Using a frequency-modulated bSSFP sequence the acquisition of many off-resonances can be performed in one single scan and a model-based reconstruction using the elliptical signal model performs well to reconstruct such measurements. Therefore, it allows the reconstruction of multiple off-resonance states for multiple heart phases from a single frequency-modulated bSSFP measurement.
|
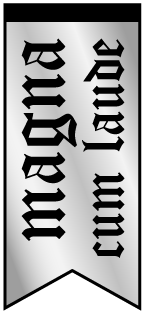 |
0601.
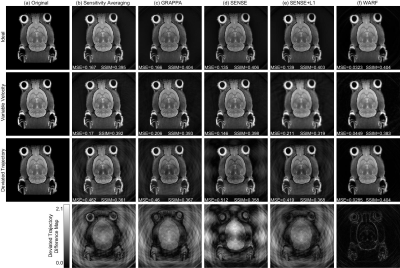 |
A Novel Image Reconstruction Algorithm for Radial MRI Data Acquired with a Rotating Radio-frequency Coil (RRFC)
Andrew Phair1, Michael Brideson1, Jin Jin2,3,4, Mingyan Li2, Stuart Crozier2, and Lawrence Forbes1
1School of Natural Sciences, University of Tasmania, Hobart, Australia, 2School of Information Technology and Electrical Engineering, University of Queensland, Brisbane, Australia, 3ARC Training Centre for Innovation in Biomedial Imaging Technology, University of Queensland, Brisbane, Australia, 4Mark and Mary Stevens Neuroimaging and Informatics Institute, University of Southern California, Los Angeles, CA, United States
Watch the Video
We present WARF, a novel reconstruction algorithm for radial MRI data acquired with a rotating radio-frequency coil (RRFC). The algorithm reconstructs each pixel as a weighted sum of all acquired data, with the weights determined by the k-space sampling pattern. The theory behind WARF leading to the derivation of appropriate weights is presented, and then WARF is applied to both simulated and experimental data sets. The results indicate WARF is achieving an improved robustness to RRFC angular velocity variability and k-space trajectory deviation compared with existing reconstruction methods.
|
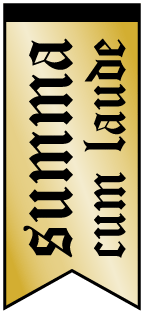 |
0602.
 |
On the Influence of Prior Knowledge in Learning Non-Cartesian 2D CINE Image Reconstruction
Kerstin Hammernik1, Gastao Cruz2, Thomas Kuestner2, Claudia Prieto2, and Daniel Rueckert1
1Department of Computing, Imperial College London, London, United Kingdom, 2School of Biomedical Engineering and Imaging Sciences, Kings College London, London, United Kingdom
Watch the Video
In this work, we study the influence of prior knowledge in learning-based non-Cartesian 2D CINE MR image reconstruction. The proposed approach uses a novel minimal deep learning setup to embed the acquired non-Cartesian multi-coil data and conventional spatio-temporal (3D and 2D+t) Fields-of-Experts regularization in a proximal gradient variational network, achieving promising results for up to 12-fold retrospectively undersampled tiny golden-angle radial CINE imaging.
|
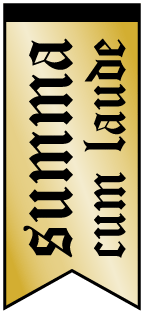 |
0603.
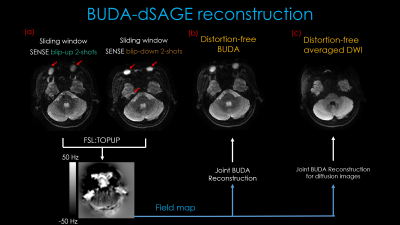 |
dSAGE enables distortion-free diffusion, spin and gradient echo imaging in 1 minute
Zijing Zhang1,2, Congyu Liao2, Jaejin Cho2, Mary Kate Manhard2, Wei-Ching Lo3, Jinmin Xu1,2, Kawin Setsomepop2,4,5, Huafeng Liu1, and Berkin Bilgic2,4
1State Key Laboratory of Modern Optical Instrumentation, College of Optical Science and Engineering, Zhejiang University, Hangzhou, China, 2Department of Radiology, A. A. Martinos Center for Biomedical Imaging, Massachusetts general hospital, Charlestown, MA, United States, 3Siemens Medical Solutions, Boston, MA, United States, 4Harvard Medical School, Boston, MA, United States, 5Harvard-MIT Health Sciences and Technology, Massachusetts Institute of Technology, Cambridge, MA, United States
Watch the Video
We propose dSAGE, a new EPI sequence for diffusion, Spin- and Gradient-echo imaging. We exploit unused sequence time during the b=0 acquisition in a diffusion experiment to collect additional T2*- and T2'-weighted contrasts with high in-plane resolution for free. We use a multi-shot acquisition with high in-plane acceleration to achieve 1x1 mm2 resolution, and alternate the phase-encoding polarities across the shots to eliminate geometric distortion using the navigator-free Blip Up-Down Acquisition(BUDA) technique(1). We demonstrate the ability of BUDA-dSAGE to provide whole-brain, distortion-free, high-SNR images with T2*-, T2'-, T2-weighted contrasts and 3-direction dMRI and apparent diffusion coefficient (ADC) maps in 1-minute.
|
|