Wednesday Parallel 5 Live Q&A |
Wednesday, 12 August 2020, 15:15 - 16:00 UTC |
Moderators: Li Feng & Shanshan Wang |
Session Number: O-61
|
0997.
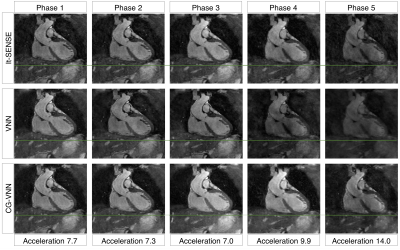 |
A Model-Based Variational Neural Network for Accelerated and Respiratory Motion-resolved 4D Cartesian Cardiac MRI
Niccolo Fuin1, Thomas Kuestner1, Gastao Cruz1, Aurelien Bustin1, René Botnar1,2, and Claudia Prieto1,2
1Biomedical Engineering Department, School of Biomedical Engineering and Imaging Sciences, King's College London, London, United Kingdom, 2Pontificia Universidad Católica de Chile, Santiago, Chile
Watch the Video
Long scan times and susceptibility to respiratory motion are major challenges in free-breathing 3D cardiac MRI. Respiratory-resolved 4D approaches deal with motion by assigning data to different respiratory bins and exploiting motion redundancies during reconstruction. However, for accelerated acquisitions this leads to highly undersampled respiratory bins, affecting image quality. Here we propose a novel unrolled VNN that reconstructs undersampled 4D cardiac MRI by exploiting motion redundancies and by using conjugate gradient to enforce data-consistency within every stage of the VNN, providing generalization of the network to the unpredictable sampling of each bin due to subject-specific respiratory motion.
|
|
0998.
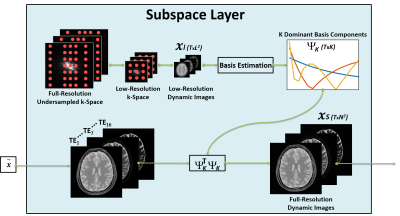 |
k-t SANTIS: Subspace Augmented Neural neTwork with Incoherent Sampling for dynamic image reconstruction
Fang Liu1,2 and Li Feng3
1Gordon Center for Medical Imaging, Massachusetts General Hospital, Harvard Medical School, Boston, MA, United States, 2Radiology, University of Wisconsin-Madison, Madison, WI, United States, 3Biomedical Engineering and Imaging Institute and Radiology, Icahn School of Medicine at Mount Sinai, New York, NY, United States
Watch the Video
A novel deep learning-based dynamic image reconstruction technique called k-t SANTIS (Subspace Augmented Neural neTwork with Incoherent Sampling) is presented in this study. Different from prior deep learning-based reconstruction approaches that rely primarily on data-driven learning, k-t SANTIS incorporates a low-rank subspace model into the deep-learning reconstruction architecture, which is implemented by adding a subspace layer to enforce an explicit subspace constraint during network training. k-t SANTIS represents a new deep image reconstruction framework with hybrid data-driven and physics-informing learning, taking additional prior knowledge available in the dataset into consideration during the training process to achieve better reconstruction performance.
|
|
0999.
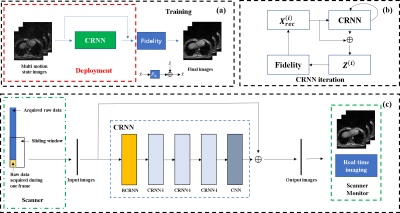 |
Dynamic Real-time MRI with Deep Convolutional Recurrent Neural Networks and Non-Cartesian Fidelity
Yufei Zhang1, Zhijun Wang1, Quan Chen1, Shuo Li1, Zekang Ding1, Chenfei Shen1, Xudong Chen1, Kang Yan1, Cong Zhang2, Xiaodong Zhou2, Yiping P. Du1, and Huajun She1
1Institute for Medical Imaging Technology, School of Biomedical Engineering, Shanghai Jiao Tong University, Shanghai, China, 2United Imaging Healthcare, Shanghai, China
Watch the Video
A convolutional recurrent neural networks (CRNN) with Non-Cartesian fidelity for 2D real-time imaging was proposed. 3D stack-of-star GRE radial sequence with self-navigator was used to acquire the data. Multiple respiratory phases were extracted from the navigator and the sliding window method was used to get the training data. The Fidelity constraints the reconstruction image to be consistent to the undersampled non-Cartesian k-space data. Convolution and recurrence improve the quality of the reconstructed images by using temporal dimension information. The reconstruction speed is around 10 frames/second, which fulfills the requirement of real-time imaging.
|
|
1000.
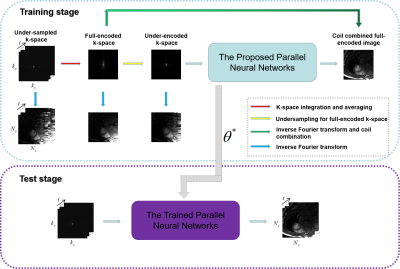 |
An Unsupervised Deep Learning Method for Parallel MR Cardiac Imaging via Time Interleaved Sampling
Ziwen Ke1,2, Yanjie Zhu3, Jing Cheng2,3, Leslie Ying4, Xin Liu3, Hairong Zheng3, and Dong Liang1,3
1Research Center for Medical AI, Shenzhen Institutes of Advanced Technology,Chinese Academy of Sciences, Shenzhen, China, 2Shenzhen College of Advanced Technology, University of Chinese Academy of Sciences, Shenzhen, China, 3Paul C. Lauterbur Research Center for Biomedical Imaging, Shenzhen Institutes of Advanced Technology,Chinese Academy of Sciences, Shenzhen, China, 4Department of Biomedical Engineering and Department of Electrical Engineering, The State University of New York, Buffalo, NY, United States
Watch the Video
Deep learning has achieved good success in cardiac MRI. However, these methods are all based on big data, and only deal with single-channel imaging. In this paper, we propose an unsupervised deep learning method for parallel MR cardiac imaging via time interleaved sampling. Specifically, a set of full-encoded reference data were built by merging the data from adjacent time frames, and used to train a network for reconstructing each coil image separately. Finally, coil images were combined via another CNN. The validation on in vivo data show that our method can achieve improved reconstruction compared with other competing methods.
|
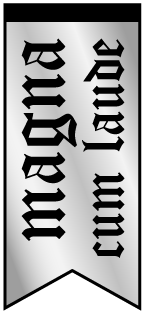 |
1001.
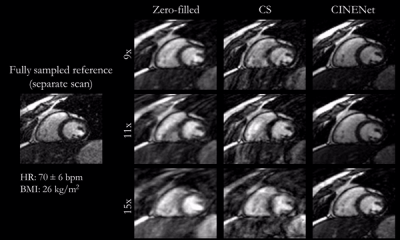 |
CINENet: Deep learning-based 3D Cardiac CINE Reconstruction with multi-coil complex 4D Spatio-Temporal Convolutions
Thomas Küstner1, Niccolo Fuin1, Kerstin Hammernik2, Aurelien Bustin1, Radhouene Neji1,3, Daniel Rueckert2, René M Botnar1, and Claudia Prieto1
1Biomedical Engineering Department, School of Biomedical Engineering and Imaging Sciences, King's College London, London, United Kingdom, 2Department of Computing, Imperial College London, London, United Kingdom, 3MR Research Collaborations, Siemens Healthcare Limited, Frimley, United Kingdom
Watch the Video
CINE MRI is the gold-standard for the assessment of cardiac function. Compressed Sensing (CS) reconstruction has enabled 3D CINE acquisition with left ventricular (LV) coverage in a single breath-hold. However, maximal achievable acceleration is limited by the performance of the selected reconstruction method. Deep learning has shown to provide good-quality reconstructions of highly accelerated 2D CINE imaging. In this work, we propose a novel 4D (3D+time) reconstruction network for prospectively undersampled 3D Cartesian cardiac CINE that utilizes complex-valued spatial-temporal convolutions. The proposed network outperforms CS in visual quality and shows good agreement for LV function to gold-standard 2D CINE.
|
|
1002.
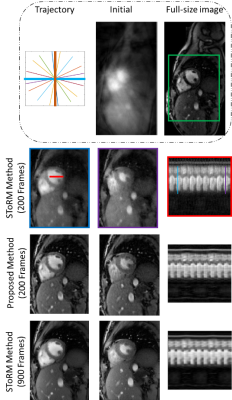 |
DYNAMIC MRI USING DEEP MANIFOLD SELF-LEARNING
Abdul Haseeb Ahmed1, Hemant Aggarwal1, Prashant Nagpal1, and Mathews Jacob1
1University of Iowa, Iowa City, IA, United States
Watch the Video
We propose a deep self-learning algorithm to learn the manifold structure of free-breathing and ungated cardiac data and to recover the cardiac CINE MRI from highly undersampled measurements. Our method learns the manifold structure in the dynamic data from navigators using autoencodernetwork. The trained autoencoder is then used as aprior in the image reconstruction framework. We have testedthe proposed method on free-breathing and ungated cardiacCINE data, which is acquired using a navigated golden-anglegradient-echo radial sequence. Results show the ability ofour method to better capture the manifold structure, thus providingus reduced spatial and temporal blurring as comparedto the SToRM reconstruction.
|
|
1003.
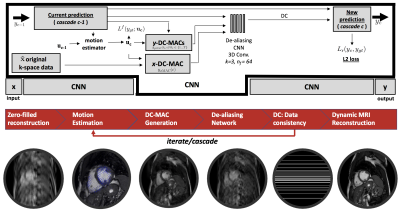 |
Deep Learning for Robust Accelerated Dynamic MRI Reconstruction for Active Acquisition Pipelines
Gavin Seegoolam1, Anthony Price2, Joseph V Hajnal2,3, and Daniel Rueckert1
1BioMedIA, Department of Computing, Imperial College London, London, United Kingdom, 2Biomedical Engineering Department, School of Biomedical Engineering and Imaging Sciences, Kings College London, London, United Kingdom, 3Centre for the Developing Brain, School of Biomedical Engineering and Imaging Sciences, Kings College London, London, United Kingdom
Watch the Video
With the advent of active acquisition-reconstruction pipelines, this study shows that by exploiting motion, robust intermediate reconstructions can be used to exploit the entire k-space budget and stabilise deep learning methods for accelerated dynamic MRI. The generated intermediate reconstructions are known as data-consistent motion-augmented cines (DC-MAC). A motion-exploiting convolutional neural network (ME-CNN), which incorporates the DC-MAC, is evaluated against a similar model to that used in a recent active acquisition-reconstruction study, the data-consistent convolutional neural network (DC-CNN). We find that the ME-CNN outperforms DC-CNN but also the DC-MAC offers better reconstructions at low acceleration rates.
|
|
1004.
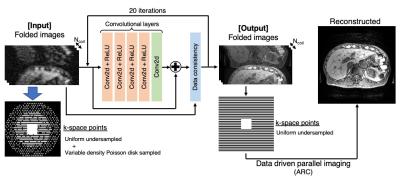 |
FITs-CNN: A Very Deep Cascaded Convolutional Neural Networks Using Folded Image Training Strategy for Abdominal MRI Reconstruction
Satoshi Funayama1, Tetsuya Wakayama2, Hiroshi Onishi1, and Utaroh Motosugi1
1Department of Radiology, University of Yamanashi, Yamanashi, Japan, 2GE Healthcare Japan, Tokyo, Japan
Watch the Video
For faster abdominal MR imaging, deep learning-based reconstruction is expected to be a powerful reconstruction method. One of the challenges in deep learning-based reconstruction is its memory consumption when it is combined with parallel imaging. To handle the problem, we propose a very deep cascaded convolutional neural networks (CNNs) using folded image training strategy (FITs). We also present that the network can be trained with FITs and shows good quality of reconstructed images.
|
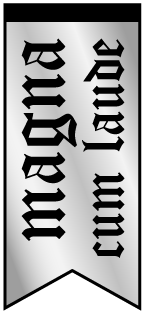 |
1005.
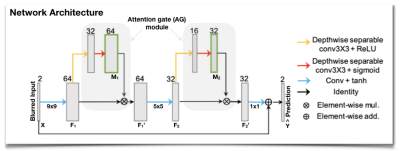 |
Attention-Gated Convolutional Neural Networks for Off-Resonance Correction of Spiral Real-Time Magnetic Resonance Imaging
Yongwan Lim1, Shrikanth S Narayanan1, and Krishna S Nayak1
1University of Southern California, Los Angeles, CA, United States
Watch the Video
Spiral acquisitions are preferred in real-time MRI because of their efficiency, which has made it possible to capture vocal tract dynamics during natural speech. A fundamental limitation of spirals is blurring and signal loss due to off-resonance, which degrades image quality at air-tissue boundaries. Here, we present a new CNN-based off-resonance correction method that incorporates an attention-gate mechanism. This leverages spatial and channel relationships of filtered outputs and improves the expressiveness of the networks. We demonstrate improved performance with the attention-gate, on 1.5T spiral speech RT-MRI, compared to existing off-resonance correction methods.
|
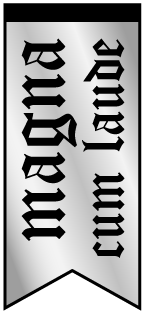 |
1006.
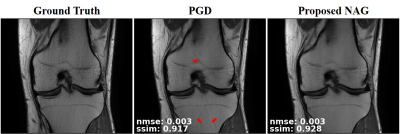 |
Unrolled Physics-Based Deep Learning MRI Reconstruction with Dense Connections using Nesterov Acceleration
Seyed Amir Hossein Hosseini1,2, Burhaneddin Yaman1,2, Steen Moeller2, Kamil Ugurbil2, Mingyi Hong1, and Mehmet Akcakaya1,2
1Electrical and Computer Engineering, University of Minnesota, Minneapolis, MN, United States, 2Center for Magnetic Resonance Research, University of Minnesota, Minneapolis, MN, United States
Watch the Video
Numerous studies have recently employed deep learning (DL) for accelerated MRI reconstruction. Physics-based DL-MRI techniques unroll an iterative optimization procedure into a recurrent neural network, by alternating between linear data consistency and neural network-based regularization units. Data consistency unit typically implements a gradient step. We hypothesize that further gains can be achieved by allowing dense connections within unrolled network, facilitating information flow. Thus, we propose to unroll a Nesterov-accelerated gradient descent that considers the history of previous iterations. Results indicate that this method considerably improves reconstruction over unrolled gradient descent schemes without skip connections.
|
|