Tuesday Parallel 3 Live Q&A |
Tuesday, 11 August 2020, 15:15 - 16:00 UTC |
Moderators: Tolga Cukur & Megan Poorman |
Session Number: O-66
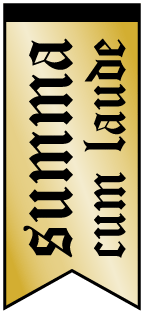 |
0663.
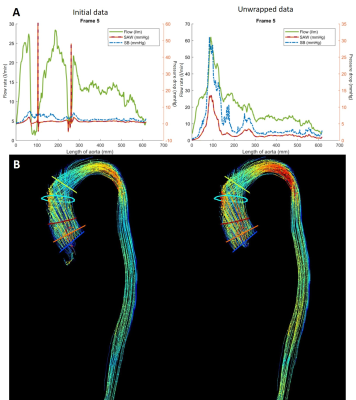 |
Multiple phase unwrapping of 4D-flow MRI in cardiovascular valves and vessels
Joao Filipe Fernandes1, Alessandro Faraci1, Marzia Rigolli2, Umar Shehzad1, Saul Myerson2, David Nordsletten1,3, and Pablo Lamata1
1School of Biomedical Engineering & Imaging Sciences, King's College London, London, United Kingdom, 2Division of Cardiovascular Medicine, Radcliffe Department of Medicine, University of Oxford, Oxford, United Kingdom, 3Department of Surgery and Biomedical Engineering, University of Michigan, Ann Arbor, MI, United States
Watch the Video
Accurate quantification of both high and low blood velocities is important for clinical decision-making in cardiovascular conditions like aortic valve stenosis. Multi-VENC acquisition is a potential solution. An alternative, without increasing acquisition time, is enabled here by correcting 4D-Flow MRI multi-aliasing along tubular structures. Our solution is based on continuity principles in successive cross-section planes, outperforming the state-of-the-art Laplacian-based solution which only performs single-wrap corrections. The accuracy of proposed method is verified in 4D-Flow MRI of 25 aortic stenotis patients with VENC of 1m/s, where double and triple unwrapping were needed to match velocity values measured by Doppler echocardiography.
|
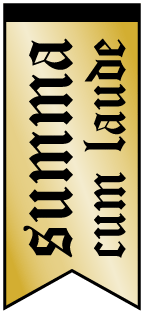 |
0664.
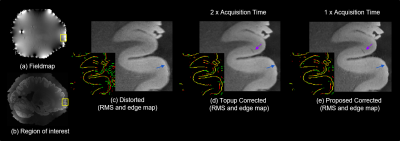 |
Edge-preserving B0 inhomogeneity distortion correction for high-resolution multi-echo ex vivo MRI at 7T
Divya Varadarajan1,2, Robert Frost1,2, Andre van der Kouwe1,2, Leah Morgan1, Bram Diamond1, Emma Boyd1, Morgan Fogarty1, Allison Stevens1, Bruce Fischl1,2, and Jonathan R Polimeni1,2,3
1Athinoula A. Martinos Center for Biomedical Imaging, Massachusetts General Hospital, Boston, MA, United States, 2Harvard Medical School, Boston, MA, United States, 3Harvard-MIT HST, Cambridge, MA, United States
Watch the Video
High-resolution multi-echo MRI at ultra-high field for ex vivo imaging is time consuming, SNR starved and suffers from B0 inhomogeneity induced geometric distortions due to low-bandwidth in the readout direction. Fieldmap-based correction cannot correct singularities in regions of severe distortion, and reversed gradient (RG) approaches double the scan time. We propose to combine an alternating-polarity acquisition scheme for multi-echo MRI with a low-resolution fieldmap based novel distortion correction algorithm that can correct singularities in half the scan time of RG and enhance SNR while preserving edges. We show several ex vivo corrected results and demonstrate generalizability to in vivo MRI.
|
|
0665.
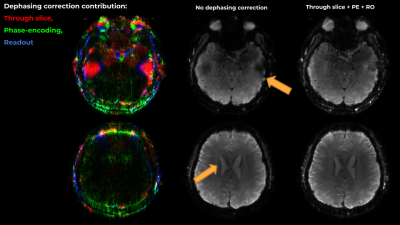 |
Accounting for B0 field-inhomogeneity-gradient induced dephasing in Cartesian and in time-resolved sequences.
Gilad Liberman1 and Kawin Setsompop1
1Department of Radiology, Massachusetts General Hospital, Charlestown, MA, United States
Watch the Video
The spatial derivative of the field inhomogeneity acts as a gradient, inducing growing intravoxel dephasing along the acquisition time, resulting in blurring, void regions (“signal loss”) and artifacts. We tackle this issue through modelling and through Cartesian time-segmentation. We show that under typical settings, field inhomogeneity gradients cause significant artifact both through-plane and in-plane, and that these artifacts can be greatly mitigated with improved reconstruction at minimal computational cost. We formalize the description of the effects of these additional gradients in k-t space in temporal units, allowing for quick apprehension of the consequences to trajectory design and reconstruction approach.
|
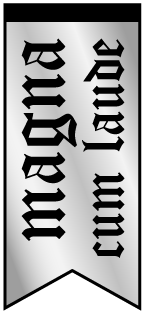 |
0666.
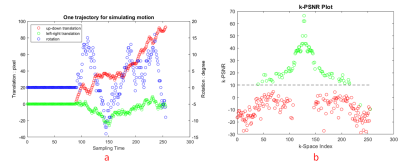 |
Alleviate motion artifacts in magnetic resonance imaging images using deep learning and compressed sensing
Long Cui1, Yang Song1, Yida Wang1, Haibin Xie1, Jianqi Li1, and Guang Yang1
1Shanghai Key Laboratory of Magnetic Resonance, Department of Physics, East China Normal University, Shanghai, China
Watch the Video
We proposed a data-driven approach to alleviate motion artifacts in Magnetic Resonance (MR) images. Firstly, MR images were acquired using a pseudo-random k-space sampling sequence. Then a convolutional network was trained to denoise MR images containing motion artifacts, before the k-space of the denoised images were compared with the raw k-space to find out k-space lines influenced by the motion. Finally, compressed sensing (CS) was applied to those unaffected lines to reconstruct the final image. Simulated experiments proved that this approach can accurately detect k-space lines influenced by motion and reconstruct images better than those reconstructed directly by CS.
|
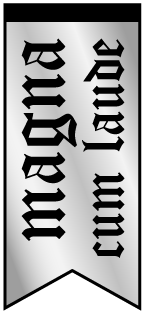 |
0667.
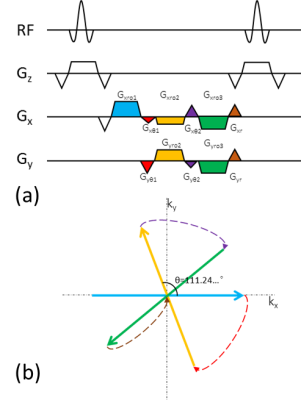 |
Echo-train radial SSFP with golden angle
Kaibao Sun1, Zheng Zhong1,2, and Xiaohong Joe Zhou1,2,3
1Center for MR Research, University of Illinois at Chicago, Chicago, IL, United States, 2Department of Bioengineering, University of Illinois at Chicago, Chicago, IL, United States, 3Departments of Radiology and Neurosurgery, University of Illinois at Chicago, Chicago, IL, United States
Watch the Video
Golden-angle radial sampling based on bSSFP is widely used in time-resolved imaging. The temporal resolution of this technique can be improved by extending a single-echo acquisition to echo-train acquisition per TR. In this study, we demonstrate an echo-train golden-angle radial bSSFP (ETGAR-bSSFP) sequence by acquiring multiple spokes in k-space to improve the temporal resolution. In addition, we introduce an integrated phase correction method and a variation of ETGAR-bSSFP to manage the image artifacts. Results from phantom and human brain have showed high quality images can be acquired from the ETGAR-bSSFP sequences and be potentially used for dynamic imaging studies.
|
|
0668.
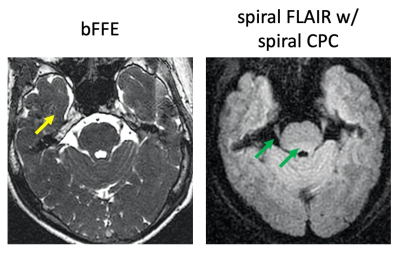 |
An improved spiral technique for imaging gamma knife subject with metal frame
Zhiqiang Li1, Sharmeen Maze1, Shiv Srivastava2, Stephen Sorensen2, and John P Karis1
1Neuroradiology, Barrow Neurological Institute, Phoenix, AZ, United States, 2St Joseph's Hospital and Medical Center, Phoenix, AZ, United States
Watch the Video
SSFP is widely used in gamma knife treatment planning in patients with trigeminal neuralgia. A metal frame worn by the patient often causes image artifacts. A spiral FLAIR technique is proposed with an improved approach for concomitant field induced phase error compensation, which is insensitive to the presence of the metal frame. Volunteer results demonstrate good delineation of the trigeminal nerve root entry zone, good adjacent CSF suppression, and good brain stem tissue contrast, therefore providing a potential alternative to SSFP for gamma knife treatment planning.
|
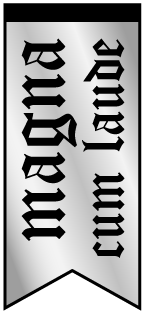 |
0669.
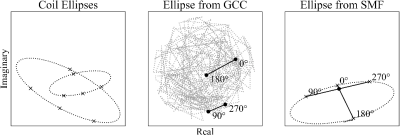 |
Robust Coil Combination for bSSFP Elliptical Signal Model
Nicholas McKibben1,2, Grayson Tarbox3, Edward V. DiBella1,2, and Neal K. Bangerter4
1Biomedical Engineering, University of Utah, Salt Lake City, UT, United States, 2Radiology and Imaging Sciences, University of Utah, Salt Lake City, UT, United States, 3Electrical and Computer Engineering, Brigham Young University, Provo, UT, United States, 4Bioengineering, Imperial College London, London, United Kingdom
Watch the Video
Elliptical parametric models of the bSSFP signal equation are used to derive band-free images given multiple phase-cycled images. For large multicoil datasets, we desire coil combination before debanding to significantly reduce computational burden. Conventional coil combination methods fail as nonlinear mappings lead to distortions of the elliptical representation. We propose phase-substitutions that can be applied with any coil combination method including sum-of-squares and which allow coil combination before computationally expensive debanding operations.
|
|
0670.
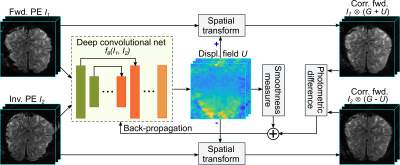 |
An Unsupervised Deep Learning Method for Correcting the Susceptibility Artifacts in Reversed Phase-encoding EPIs
Soan Thi Minh Duong1, Sui Paul Ang1, and Mark Matthias Schira2
1School of Electrical, Computer and Telecommunications Engineering, University of Wollongong, Wollongong, Australia, 2School of Psychology, University of Wollongong, Wollongong, Australia
Watch the Video
We introduce a deep learning method, named S-Net, to correct the susceptibility artifacts in a pair of reversed phase-encoding (PE) echo-planar imaging images. The S-Net is trained in an unsupervised manner using a set of reversed-PE pairs. For a new reversed-PE pair, the corrected images are computed rapidly by evaluating the learned S-Net. Evaluation of three datasets demonstrates equally good correction performance as much lower computation time (1-3s) than state-of-the-art SAC methods such as AISAC (50-60s) or TOPUP (over 1000s). This fast performance provides a dramatic speedup for medical imaging processing pipelines and makes the real-time correction for MR-scanners feasible.
|
|
0671.
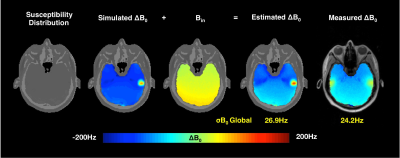 |
B0 field estimation using Ultrashort echo time/Dixon imaging with a 4-class tissue segmentation
Jiazheng Zhou1,2, Ali Aghaeifer1, Gisela Hagberg1,3, and Klaus Scheffler1,3
1High-Field Magnetic Resonance, Max Planck Institute of Biological Cybernetics, Tübingen, Germany, 2Graduate Training Centre of Neuroscience, IMPRS, University of Tübingen, Tübingen, Germany, 3Biomedical Magnetic Resonance, University Hospital Tübingen (UKT), Tübingen, Germany
Watch the Video
We use a UTE sequence combining with Dixon method to obtain the subject specific susceptibility distribution, with 4-class tissue segmentation. The susceptibility model was then used to simulate motion-induced B0 change for two head positions. A good agreement between the simulated and measured field map has been observe. A forward field map predicting strategy was explored using the susceptibility model.
|
|
0672.
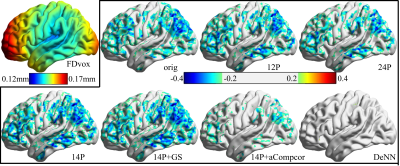 |
Disentangling time series between gray matter and non-gray matter tissue using deep neural network improves resting state fMRI data quality
Zhengshi Yang1, Xiaowei Zhuang1, Karthik Sreenivasan1, Virendra Mishra1, and Dietmar Cordes1,2
1Cleveland Clinic Lou Ruvo Center for Brain Health, Las Vegas, NV, United States, 2Department of Psychology and Neuroscience, University of Colorado, Boulder, CO, United States
Watch the Video
The fluctuation introduced by head motion, cardiac and respiratory fluctuations and other noise sources considerably confounds the interpretation of resting-state fMRI data. These noise fluctuations widely spread the whole brain regardless of the kinds of brain tissues, however, neural activity is more likely limited to gray matter tissue. Considering that the contribution of neural activity varies in different brain tissues, we hypothesized that disentangling gray matter and non-gray matter time series can clean fMRI data and improve the data quality. With such a hypothesis, we proposed a deep neural network method to denoise resting state fMRI data.
|
|