Thursday Parallel 1 Live Q&A |
Thursday, 13 August 2020, 14:20 - 15:05 UTC |
Moderators: Akshay Chaudhari & Jo Schlemper |
Session Number: PP-23
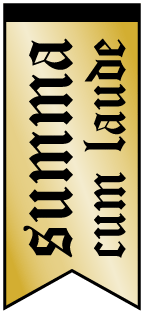 |
1022.
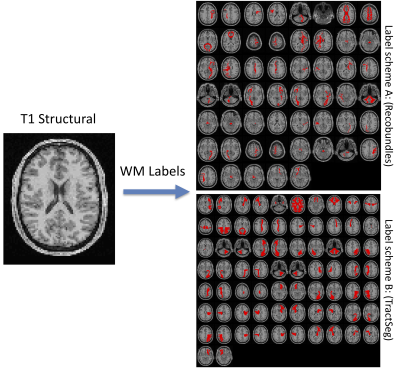 |
Learning white matter fingerprints from structural information
Colin Hansen1, Qi Yang1, Francois Rheault2, Bramsh Qamar3, Owen Williams4, Susan Resnick4, Eleftherios Garyfallidis3, Adam W Anderson5,6, Maxime Descoteaux2, Bennett A Landman5,6,7,8, and Kurt G Schilling5
1Computer Science, Vanderbilt University, Nashville, TN, United States, 2Sherbrooke Connectivity Imaging Laboratory (SCIL), Universite de Sherbrooke, Sherbrooke, QC, Canada, 3Intelligent Systems Engineering, Indiana University Bloomington, Bloomington, IN, United States, 4Laboratory of Behavioral Neuroscience, National Institute on Aging, Baltimore, MD, United States, 5Vanderbilt University Institute of Imaging Science, Nashville, TN, United States, 6Biomedical Engineering, Vanderbilt University, Nashville, TN, United States, 7Radiology and Radiological Sciences, Vanderbilt University Medical Center, Nashville, TN, United States, 8Electrical Engineering, Vanderbilt University, Nashville, TN, United States
Watch the Video
Here, we present a tool and reconstruction method to label white matter pathways directly on structural images without the need for diffusion MRI or tractography. A 3D U-net was trained utilizing 1109 scan sessions where fiber pathways were segmented using two different segmentation schemes. Results on testing datasets show anatomically viable segmentations and moderate-to-high volume overlaps with gold-standard pathways, on par with scan-rescan reproducibility of tractography on the same datasets. We envision the use of this tool for visualizing the expected course of white matter pathways when diffusion data are not available.
|
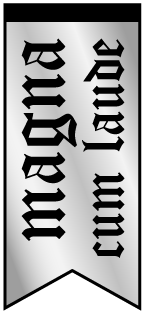 |
1023.
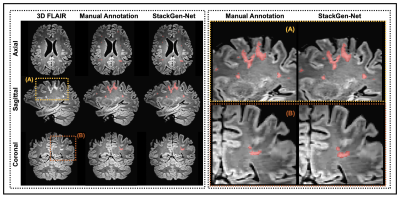 |
StackGen-Net: A Stacked Generalization of 3D Orthogonal Convolutional Neural Networks for Improved Detection of White Matter Hyperintensities
Lavanya Umapathy1, Gloria J Guzman Perez-Carrillo2, Mahesh Bharath Keerthivasan2,3, Maria I Altbach2, Blair Winegar2, Craig Weinkauf4, and Ali Bilgin1,2,5
1Department of Electrical and Computer Engineering, University of Arizona, Tucson, AZ, United States, 2Department of Medical Imaging, University of Arizona, Tucson, AZ, United States, 3Siemens Healthcare USA, Tucson, AZ, United States, 4Department of Surgery, University of Arizona, Tucson, AZ, United States, 5Department of Biomedical Engineering, University of Arizona, Tucson, AZ, United States
Watch the Video
Detection and quantification of White Matter Hyperintensities (WMH) on T2-FLAIR images can provide valuable information to assess neurological disease progression. We propose a fully automated stacked generalization ensemble of three orthogonal 3D Convolutional Neural Networks (CNNs), StackGen-Net, to detect WMH on 3D FLAIR images. Each orthogonal CNN predicts WMH on axial, sagittal, and coronal orientations. The posteriors are then combined using a Meta CNN. StackGen-Net outperforms individual CNNs in the ensemble, their ensemble combination, as well as some state-of-the-art deep learning-based models. StackGen-Net can reliably detect and quantify WMH in clinically feasible times, with performance comparable to human inter-observer variability.
|
|
1024.
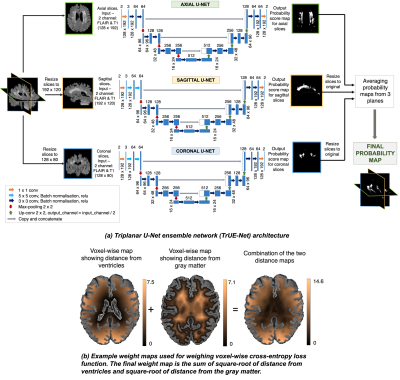 |
Detection of white matter hyperintensities using Triplanar U-Net ensemble network
Vaanathi Sundaresan1, Mark Jenkinson1, Giovanna Zamboni2, and Ludovica Griffanti1
1FMRIB, Wellcome Centre for Integrative Neuroimaging (WIN), Nuffield Department of Clinical Neurosciences, University of Oxford, Oxford, United Kingdom, 2Centre for prevention of Stroke and Dementia, Nuffield Department of Clinical Neurosciences, University of Oxford, Oxford, United Kingdom
Watch the Video
We propose a triplanar U-Net ensemble network (TrUE-Net) model for detecting white matter hyperintensities (WMHs) on structural brain MR images. The network uses a combination of loss functions based on the anatomical distribution of WMHs. The model takes T1-weighted and FLAIR images as input channels. The U-Nets in three planes (axial, coronal, sagittal) provides three 2D probability maps, which are combined by averaging across planes to obtain the final probability map. When evaluated on three different cohorts from the MICCAI WMH segmentation challenge dataset, TrUE-Net provided better average performance (Dice=0.78, voxel-wise TPR=0.75), when compared to FSL-BIANCA (Dice=0.69, voxel-wise TPR=0.74).
|
|
1025.
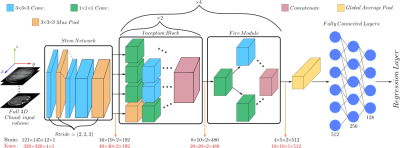 |
Organ-based estimation of the chronological age based on 3D MRI scans
Sherif Abdulatif1, Karim Armanious1,2, Anish Rao Bhaktharaguttu1, Thomas Küstner2, Bin Yang1, and Sergios Gatidis2
1University of Stuttgart, Stuttgart, Germany, 2University Hospital Tübingen, Tübingen, Germany
Watch the Video
Age is an essential clinical parameter. It is often utilized as a risk factor for various disorders with the potential of influencing therapeutic decisions. However, a discrepancy exists between the chronological age (CA) and the biological age (BA) of an individual due to many factors such as medical history, genetics and lifestyle. In this preliminary work, we propose a novel deep-learning architecture for organ-specific CA estimation from 3D MR volumes for the brain and knee. We hypothesize that the introduced organ-specific approach would enable future analysis of the BA as different organs are expected to exhibit different aging characteristics.
|
|
1026.
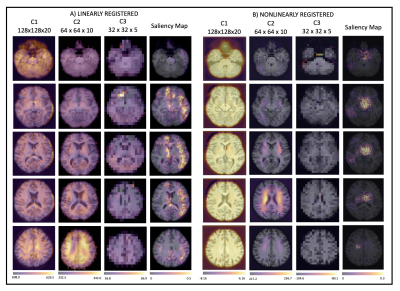 |
Assessing the effect of registration and model quality using attention gates for brain-age prediction with convolutional neural networks.
Nicola K Dinsdale1, Emma Bluemke2, Mark Jenkinson1, and Ana IL Namburete2
1WIN, University of Oxford, Oxford, United Kingdom, 2IBME, University of Oxford, Oxford, United Kingdom
Watch the Video
Nonlinear registration forms a part of standard MRI neuroimaging pipelines but leads to suppression of morphological information. Using attention gates within a convolutional neural network, we explore the effect of the nonlinear registration on age prediction, comparing to linear registration. We show that the network is driven by interpolation effects near the ventricles when trained with nonlinear data, whereas when trained with linear data it considers the whole brain volume. The network may, therefore, be missing cortical changes, limiting the utility of the networks in detecting the early stages of neurological disease.
|
|
1027.
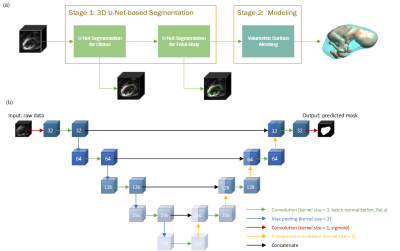 |
Deep Learning-based Fetal-Uterine Motion Modeling from Volumetric EPI Time Series
Muheng Li1, Yi Xiao1, Tingyin Liu2, Junshen Xu3, Esra Turk4, Borjan Gagoski4,5, Karen Ying1, Polina Golland2,3, P. Ellen Grant4,5, and Elfar Adalsteinsson3,6
1Department of Engineering Physics, Tsinghua University, Beijing, China, 2Computer Science and Artificial Intelligence Laboratory (CSAIL), Massachusetts Institute of Technology, Cambridge, MA, United States, 3Department of Electrical Engineering and Computer Science, Massachusetts Institute of Technology, Cambridge, MA, United States, 4Fetal-Neonatal Neuroimaging and Developmental Science Center, Boston Children’s Hospital, Boston, MA, United States, 5Harvard Medical School, Boston, MA, United States, 6Institute for Medical Engineering and Science, Massachusetts Institute of Technology, Cambridge, MA, United States
Watch the Video
We propose a three-dimensional convolutional neural network applied to echo planar EPI time series of pregnant women for the automatic segmentation of the uterus (placenta excluded) and fetal body. The segmentation results are utilized to create a dynamic model for the fetus for retrospective analyses. The 3D dynamic fetal-uterine motion model will provide quantitative information of fetal motion characteristics for diagnostic purposes and may guide future fetal imaging strategies where adaptive, online slice prescription is used to mitigate motion artifacts.
|
|
1028.
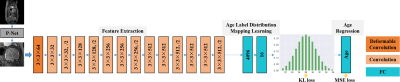 |
Multi-path Deformable Convolutional Neural Network with Label Distribution Learning for Fetal Brain Age Prediction
Lufan Liao1, Xin Zhang2, Fenqiang Zhao1, Jingjiao Lou1, Li Wang1, Xiangmin Xu2, He Zhang3, and Gang Li1
1Department of Radiology and BRIC, The University of North Carolina at Chapel Hill, Chapel Hill, CA, United States, 2School of Electronic and Information Engineering, South China University of Technology, GUANGZHOU, China, 3Department of Radiology, Obstetrics and Gynecology Hospital, Fudan University, ShangHai, China
In this study, an end-to-end framework, combining deformable convolution and label distribution learning, is developed for fetal brain age prediction based on MRI. Furthermore, a multi-path architecture is proposed to deal with multi-view MRI scenarios. Experiments on the collected dataset demonstrate that the proposed model achieves promising performance.
|
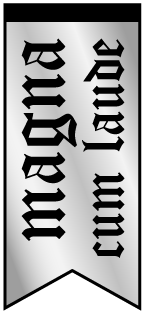 |
1029.
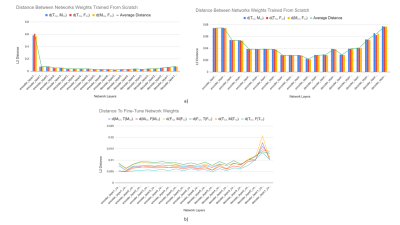 |
Exploration of Feature Space in Semantic Segmentation Convolutional Neural Networks
Logan A Thorneloe1, Arjun D Desai2,3, Garry E Gold3,4,5, Brian A Hargreaves2,3,4, Neal K Bangerter6, and Akshay S Chaudhari3
1Electrical Engineering, Brigham Young University, Provo, UT, United States, 2Electrical Engineering, Stanford University, Stanford, CA, United States, 3Radiology, Stanford University, Stanford, CA, United States, 4Bioengineering, Stanford University, Stanford, CA, United States, 5Orthopedic Surgery, Stanford University, Stanford, CA, United States, 6Bioengineering, Imperial College London, London, United Kingdom
Watch the Video
Recent advances in deep learning and convolutional neural networks (CNNs) have shown promise for automatic segmentation in MR images. However, because of the stochastic nature of the training process, it is difficult to interpret what information networks learn to represent. In this study, we explore how differences in learned weights between networks can be used to express semantic relationships between different tissues. For cartilage and meniscus segmentation in the knee, we show that network generalizability for segmenting tissues can be measured by distances between networks. We also use these findings to motivate robust training policies for fine-tuning with limited data.
|
|
1030.
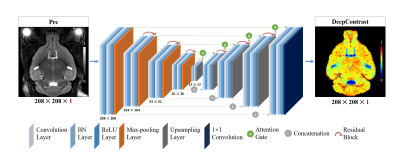 |
SUBSTITUTING GADOLINIUM IN BRAIN MRI USING DEEPCONTRAST: A PROOF-OF-CONCEPT STUDY IN MICE
Haoran Sun1, Xueqing Liu1, Xinyang Feng1, Chen Liu2, Nanyan Zhu3, Sabrina Josefina Gjerswold-Selleck1, Hong-Jian Wei4,5, Pavan Shankar Upadhyayula5,6, Angeliki Mela5,6, Cheng-Chia Wu4,5, Peter Canoll5,6, Andrew F. Laine1, John Thomas Vaughan1,
Scott A. Small7,8,9, and Jia Guo7,10
1Department of Biomedical Engineering, Columbia University, New York, NY, United States, 2Department of Electrical Engineering, Columbia University, New York, NY, United States, 3Department of Biological Science, Columbia University, New York, NY, United States, 4Department of Radiation Oncology, Columbia University, New York, NY, United States, 5Columbia University Irving Medical Center, Columbia University, New York, NY, United States, 6Department of Pathology and Cell Biology, Columbia University, New York, NY, United States, 7Department of Psychiatry, Columbia University, New York, NY, United States, 8Departments of Neurology, Columbia University, New York, NY, United States, 9Departments of Radiology, Columbia University, New York, NY, United
States, 10Mortimer B. Zickerman Mind Brain Behavior Institute, Columbia University, New York, NY, United States
Watch the Video
Cerebral blood volume (CBV) is a hemodynamic correlate of oxygen metabolism and reflects brain activity and function. High-resolution CBV maps can be generated using the steady-state gadolinium-enhanced MRI technique. Recent studies suggest that the exogenous gadolinium based contrast agent (GBCA) can accumulate in the brain after frequent use. Here, we develop and optimize a deep learning algorithm, DeepContrast, which performs equally well as exogenous GBCA in mapping CBV of the normal brain tissue and enhancing glioblastoma. Together, these studies validate our hypothesis that a deep learning approach can potentially replace the need for GBCAs in brain MRI.
|
|
1031.
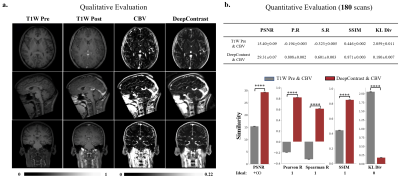 |
Substituting Gadolinium In Human Brain MRI Using DeepContrast
Chen Liu1,2, Nanyan Zhu1,3, Xinyang Feng4,5, Frank A Provenzano6, John T Vaughan4,7,8, Scott A Small6,7,9, and Jia Guo8,9
1These authors contribute equally to this work and are joint first authors, New York, NY, United States, 2Electrical Engineering, Columbia University, New York, NY, United States, 3Biological Science, Columbia University, New York, NY, United States, 4Biomedical Engineering, Columbia University, New York, NY, United States, 5Facebook, San Francisco, NY, United States, 6Neurology, Columbia University, New York, NY, United States, 7Radiology, Columbia University, New York, NY, United States, 8Mortimer B. Zuckerman Mind Brain Behavior Institute, Columbia University, New York, NY, United States, 9Psychiatry, Columbia University, New York, NY, United States
Watch the Video
MRI estimation of cerebral blood volume (CBV) is useful in mapping potential brain function. To obtain high-resolution CBV maps, it typically requires intravenous (IV) injections of Gadolinium-based contrast agents (GBCAs), the use of which has come under new scrutiny. Here, we design and implement a deep learning algorithm, DeepContrast, to estimate GBCA contrast directly from T1-weighted (T1W) structural MRI. The predicted contrast performs equally well as the GBCA-enhanced CBV map even in mapping subtle age-related functional changes in the human brain. Therefore, our study demonstrates the feasibility of substituting GBCA in human brain MRI using DeepContrast.
|
|
1032.
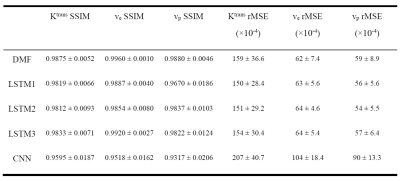 |
Estimation of Pharmacokinetic Parameters from DCE-MRI by Extracting Long and Short Time-dependent Features Using a LSTM network
Jiaren Zou1, James Balter1,2, and Yue Cao1,2,3
1Department of Radiation Oncology, University of Michigan, Ann Arbor, MI, United States, 2Department of Biomedical Engineering, University of Michigan, Ann Arbor, MI, United States, 3Department of Radiology, University of Michigan, Ann Arbor, MI, United States
Watch the Video
Conventional nonlinear least squares (LS) methods to fit DCE-MRI to a pharmacokinetic (PK) model are time-consuming. We propose a long Short-Term Memory (LSTM) network that is capable of efficiently learning temporal dependency in sequence data to map PK parameters from single-voxel DCE signals with their corresponding AIFs. The LSTM model showed 90 folds of computation time reduction with comparable performance to LS fitting, while outperforming it for temporally sparsely sampled DCE-MRI. The proposed model can potentially accelerate the data acquisition and PK parameter inference of DCE-MRI.
|
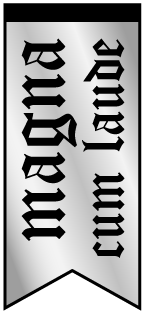 |
1033.
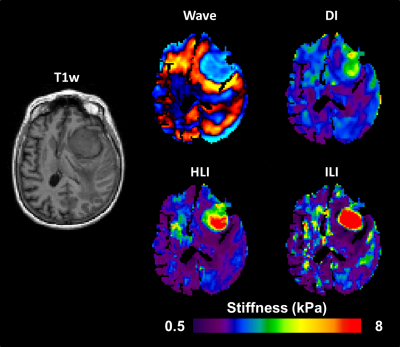 |
Improved Depiction of Meningioma Boundaries in MR Elastography Using a Novel Inhomogeneous Learned Inversion
Jonathan M Scott1, Arvin Arani2, Armando Manduca2, Joshua D Trzasko2, John Huston III2, Richard L Ehman2, and Matthew C Murphy2
1Medical Scientist Training Program, Mayo Clinic, Rochester, MN, United States, 2Radiology, Mayo Clinic, Rochester, MN, United States
Watch the Video
Magnetic Resonance Elastography stiffness estimates in small focal lesions are often inaccurate. The assumption of material homogeneity made by most inversion algorithms likely contributes to these errors. Here we describe a machine-learning based inversion algorithm trained on wave simulations of materials with piecewise smooth stiffness variations (Inhomogeneous Learned Inversion, ILI). We show that ILI offers improved delineation of tumor boundaries over two inversions assuming material homogeneity in a series of 17 patients with stiff meningiomas.
|
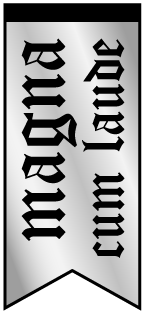 |
1034.
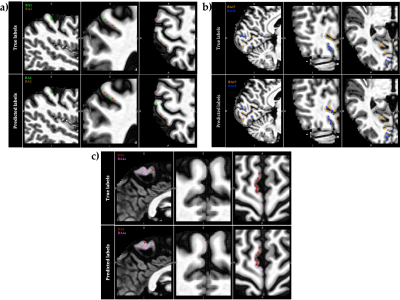 |
In vivo voxel-wise parcellation of the human cerebral cortex using 3D MR fingerprinting (MRF) and supervised machine learning classification
Shahrzad Moinian1, Viktor Vegh1,2, and David Reutens1,2
1Centre for Advanced Imaging, The University of Queensland, Brisbane, Australia, 2ARC Centre for Innovation in Biomedical Imaging Technology, The University of Queensland, Brisbane, Australia
Watch the Video
The human cerebral cortex may be divided into functionally different, microarchitectonically distinct areas. While quantitative multi-modal MRI methods can reveal microstructural characteristics of cortical tissue, accurate microarchitectural parcellation of the entire cortex is yet to be attained. Here, we examine a novel method of automated in vivo voxel-wise cortical parcellation which exploits the area-specific microstructural information present in MR fingerprinting (MRF) signals. A Radial Basis Function Support Vector Machine (RBF-SVM) classifier, trained with a volume-based feature representation, achieved a macro-average area under the Receiver Operating Characteristic curve (ROC-AUC) of 0.83.
|
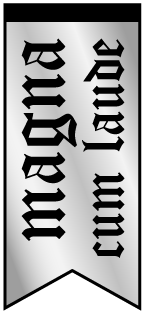 |
1035.
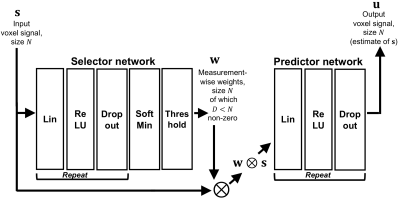 |
SARDU-Net: a new method for model-free, data-driven experiment design in quantitative MRI
Francesco Grussu1,2, Stefano B. Blumberg2, Marco Battiston1, Andrada Ianuș3, Saurabh Singh4, Fiona Gong4, Hayley Whitaker4, David Atkinson4, Claudia A. M. Gandini Wheeler-Kingshott1,5,6, Shonit Punwani4, Eleftheria Panagiotaki2, Thomy Mertzanidou2, and Daniel C. Alexander2
1Queen Square MS Centre, Queen Square Institute of Neurology, Faculty of Brain Sciences, University College London, London, United Kingdom, 2Centre for Medical Image Computing, Department of Computer Science, University College London, London, United Kingdom, 3Champalimaud Research, Champalimaud Centre for the Unknown, Lisbon, Portugal, 4Centre for Medical Imaging, University College London, London, United Kingdom, 5Department of Brain and Behavioural Sciences, University of Pavia, Pavia, Italy, 6Brain MRI 3T Center, IRCCS Mondino Foundation, Pavia, Italy
Watch the Video
This work introduces the “Select and retrieve via direct up-sampling” network (SARDU-Net), a new method for model-free, data-driven quantitative MRI (qMRI) experiment design. SARDU-Net identifies informative measurements within lengthy acquisitions and reconstructs fully-sampled signals from a sub-protocol, without prior information on the MRI contrast. It combines two deep networks: a selector, which selects a signal sub-sample, and a predictor, which retrieves input signals. SARDU-Net can be run with standard computational resources and can increase the clinical appeal of qMRI. Here we demonstrate its potential on qMRI of prostate and spinal cord, two areas where fast acquisitions are key.
|
|
1036.
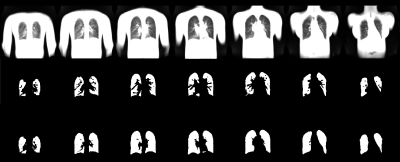 |
End-to-End Full Automated Pipeline using a Convolutional Neural Network for Lung Segmentation in Phase-Resolved Functional Lung (PREFUL) MRI
Cristian Crisosto1,2, Andreas Voskrebenzev1,2, Marcel Gutberlet1,3, Filip Klimeš1,2, Frank Wacker1,2, Till Kaireit1,2, Gesa Poeler1,2, Lea Behrendt1,2, Christopher Korz1, and Jens Vogel-Claussen1,2
1Institute of Diagnostic and Interventional Radiology, Hanover Medical School, Hannover, Germany, 2Biomedical Research in Endstage and Obstructive Lung Disease Hannover (BREATH), German Center for Lung Research (DZL), Hannover, Germany, 3Biomedical Research in Endstage and 3 Obstructive Lung Disease Hannover (BREATH), German Center for Lung Research (DZL), Hannover, Germany
Watch the Video
Translation and establishment of complex pulmonary magnetic resonance (MR) imaging techniques in the clinics requires a reliable, fully automated and fast calculation. In this work we present a semantic convolutional neural network (CNN) model for lung parenchyma and vessel segmentation in combination with parallelized computation on a high-performance computer to design an end-to-end pipeline for phase-resolved functional lung (PREFUL) MRI. The CNN was trained (n=1118) and validated (n=1064) with manually segmented images by a trained radiologist. Automatic segmentation of lung parenchyma was achieved for all tested images.
|
|