Tuesday Parallel 3 Live Q&A |
Tuesday, 11 August 2020, 15:15 - 16:00 UTC |
Moderators: Teresa Correia & Joshua Trzasko |
Session Number: PP-24
|
0673.
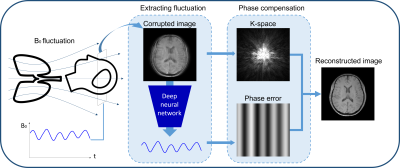 |
DeepRespi: Retrospective correction for respiration-induced B0 fluctuation artifacts using deep learning
Hongjun An1, Hyeong-Geol Shin1, Woojin Jung1, and Jongho Lee1
1Department of Electrical and computer Engineering, Seoul National University, Seoul, Korea, Republic of
Watch the Video
B0 fluctuation from respiration can induce significant artifacts in MRI images. In this study, a new retrospective correction method that requires no modification in sequences (e.g. no navigator) is proposed. This method utilizes a convolution neural network (CNN), DeepRespi, to extract a respiration pattern from a corrupted image. The respiration pattern is applied back to the corrupted image for phase compensation. When tested, the CNN successfully extracted the respiration pattern (correlation coefficient = 0.94 ± 0.04) and the corrected images showed on average 68.9 ± 13.2% reduction in NRMSE when comparing the corrupted vs. corrected images.
|
|
0674.
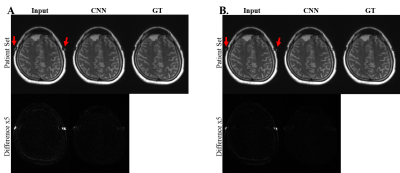 |
Convolutional Neural Network for Slice Encoding for Metal Artifact Correction (SEMAC) MRI
Sunghun Seo1, Won-Joon Do1, Huan Minh Luu1, Ki Hwan Kim1, Seung Hong Choi2, and Sung-Hong Park1
1Department of Bio and Brain Engineering, Korea Advanced Institute of Science and Technology, Daejeon, Korea, Republic of, 2Department of Radiology, Seoul National University College of Medicine, Seoul, Korea, Republic of
Watch the Video
We propose convolutional neural network (CNN) to accelerate Slice Encoding for Metal Artifact Correction (SEMAC). The concept was tested on metal‑embedded agarose phantoms and patients with metallic neuro plates in the cerebral region. CNN was trained to output images with high SEMAC factor from input images with low SEMAC factor, achieving acceleration factors of 2 or 3. The metal artifacts in low SEMAC factor data were visually and quantitatively suppressed well in the output of CNN (p<0.01), which was comparable to that of the high SEMAC factor. The study shows the feasibility of reducing scan time of SEMAC through CNN.
|
|
0675.
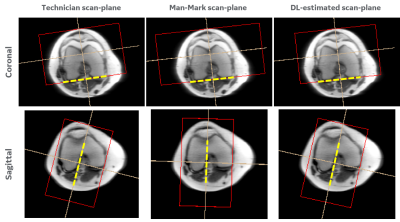 |
Consistency in human and machine-learning based scan-planes for clinical knee MRI planning
Chitresh Bhushan1, Dattesh D. Shanbhag2, Andre Maximo3, Uday Patil2, Radhika Madhavan1, Matthew Frick4, Kimberly K. Amrami4, Desmond Teck Beng yeo1, and Thomas Foo1
1GE Research, Niskayuna, NY, United States, 2GE Healthcare, Bengaluru, India, 3GE Healthcare, Rio de Janeiro, Brazil, 4Mayo Clinic, Rochester, MN, United States
Video Permission Withheld
We evaluate the consistency and clinical applicability of our automated deep-learning based intelligent slice placement (ISP) approach for knee scan planning. We use 146 clinical knee exams that were retrospectively selected to have anatomically consistent scan planning along with manual-marking from in-house radiologist to access the variability across MR technicians. The results indicate that our automated ISP approach has better consistency than the variability seen across MR technicians for coronal and sagittal knee scan planning, indicating promising clinical applicability of our automated ISP approach.
|
|
0676.
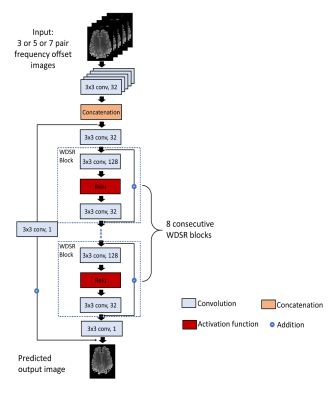 |
Accelerating the B0 Inhomogeneity Correction for GluCEST Imaging Using Deep Learning
Yiran Li1, Danfeng Xie1, Abigail Cember2, Ravi Prakash Reddy Nanga2, Hanlu Yang1, Dushyant Kumar2, Hari Hariharan2, Li Bai1, John A. Detre3, Ravinder Reddy2, and Ze Wang4
1Department of Electrical and Computer Engineering, Temple University, Philadelphia, PA, United States, 2Department of Radiology, University of Pennsylvania Perelman School of Medicine, Philadelphia, PA, United States, 3Department of Neurology, University of Pennsylvania Perelman School of Medicine, Philadelphia, PA, United States, 4Department of Diagnostic Radiology and Nuclear Medicine, University of Maryland School of Medicine, Baltimore, MD, United States
Glutamate Chemical Exchange Saturation Transfer (GluCEST) MRI is a noninvasive technique for mapping parenchymal glutamate in the brain. GluCEST signal is sensitive to magnetic field (B0) inhomogeneity. Corrections for B0 inhomogeneity often require repeated data acquisitions at several saturation offset frequencies, which however dramatically prolongs the total acquisition time and can cause practical issues such as increased sensitive to patient motions. Another technique challenge in GluCEST MRI is the low signal-to-noise-ratio (SNR) as the signal is derived from the small z-spectrum difference. Both issues were addressed in this study with a novel deep learning-based algorithm armed with wide activation neurons.
|
|
0677.
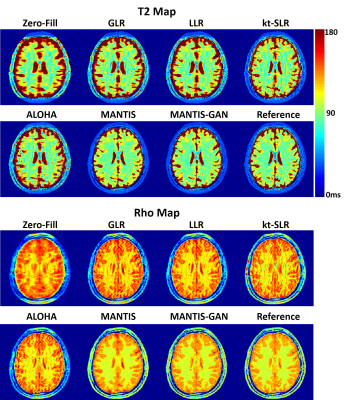 |
High-Performance Rapid Quantitative Imaging with Model-Based Deep Adversarial Learning
Fang Liu1,2 and Li Feng3
1Gordon Center for Medical Imaging, Massachusetts General Hospital, Harvard Medical School, Boston, MA, United States, 2Radiology, University of Wisconsin-Madison, Madison, WI, United States, 3Biomedical Engineering and Imaging Institute and Radiology, Icahn School of Medicine at Mount Sinai, New York, NY, United States
Watch the Video
The purpose of this work was to develop a novel deep learning-based reconstruction framework for rapid MR parameter mapping. Building upon our previously proposed Model-Augmented Neural neTwork with Incoherent k-space Sampling (MANTIS) technique combining efficient end-to-end CNN mapping and k-space consistency to enforce joint data and model fidelity, this new method further extends to incorporate the latest adversarial training (MANTIS-GAN), so that more realistic parameter maps can be directly estimated from highly-accelerated k-space data. The performance of MANTIS-GAN was demonstrated for fast T2 mapping. Our study showed that MANTIS-GAN represents a promising approach for efficient and accurate MR parameter mapping.
|
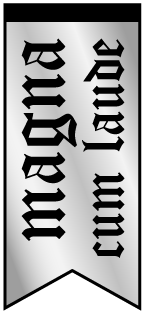 |
0678.
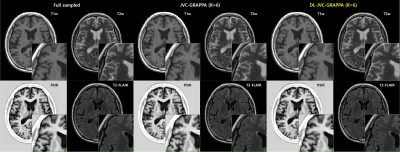 |
Joint Parallel Imaging reconstruction with Deep Learning for Multi-Contrast Synthetic MRI
Jae-Hun Lee1, Kanghyun Ryu1, Sung-Min Gho2, Ho-Sung Kim3, Mohammed A. Al-masni1, and Dong-Hyun Kim1
1Deparment of Electrical & Electronic Engineering, Yonsei Univ., Seoul, Korea, Republic of, 2MR Collaboration and Development, GE Healthcare, Seoul, Korea, Republic of, 3Department of Radiology, Asan medical center, Seoul, Korea, Republic of
Watch the Video
Synthetic MRI or magnetic resonance imaging compilation (MAGiC) uses multiple-dynamic multiple-echo acquisition(MDME) and acquires 8 contrast images in a single scan. SENSE or GRAPPA method is conventionally used to reconstruct undersampled acquisition for respective contrast images. However, the method enables limited acceleration up to 2~3. In this study, combined reconstruction method (Joint Parallel Imaging with Deep Learning) is explored. The proposed method shows acceptable image quality with RMSE (4.6%) at the higher acceleration factor (up to 8) comparable to conventional GRAPPA with acceleration rate of 2~3.
|
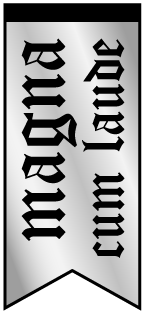 |
0679.
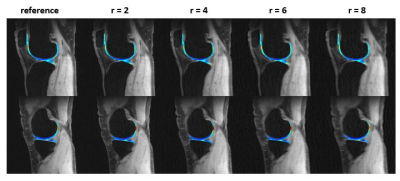 |
Joint 3D parameter mapping and motion correction using a kernel low rank method with offline training
Chaoyi Zhang1, JeeHun Kim2, Hongyu Li1, Peizhou Huang3, Ruiying Liu1, Dong Liang4, Xiaoliang Zhang3, Xiaojuan Li2, and Leslie Ying1,3
1Electrical Engineering, University at Buffalo, SUNY, Buffalo, NY, United States, 2Biomedical Engineering, Program of Advanced Musculoskeletal Imaging (PAMI), Cleveland Clinic, OH, United States, 3Biomedical Engineering, University at Buffalo, SUNY, Buffalo, NY, United States, 4Paul C Lauterbur Research Center for Biomedical Imaging, Shenzhen institutes of Advanced Technology, Shenzhen, China
Watch the Video
Magnetic resonance parameter mapping (e.g. T1, T2, T2* and T1ρ) has shown potential in quantitative assessment while the clinical applications are limited by long acquisition time especially in 3D acquisition. In our previous work, we use single-exponential model to generate off-line single-exponential training data instead of low resolution training data, which reduced the reconstruction time. In clinical use, when motion is introduced in acquisition, single-exponential model is not satisfied and the reconstruction may fail. With this motivation, this abstract alternatively reconstruct the images and correct motion in 3D parameter mapping.
|
|
0680.
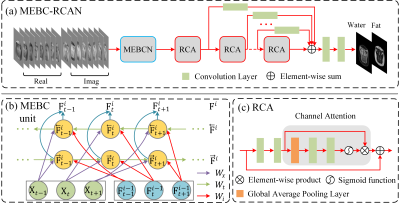 |
Construction of water-fat separation deep learning model combined with multi-echo nature of gradient-recalled echo sequence
Kewen Liu1, Xiaojun Li1, Qinjia Bao2, Chaoyang Liu3, Hongxia Xiong4, Zhao Li3, Yuan Ma1, Panpan Fang1, and Yalei Chen1
1School of Information Engineering, Wuhan University of Technology, Wuhan, China, 2United Imaging of Scientific Instruments, Shanghai, China, 3State Key Laboratory of Magnetic Resonance and Atomic and Molecular Physics, Wuhan Institute of Physics and Mathmatics, Innovation Academy for Precision Measurement Science and Technology, Wuhan, China, 4School of Civil Engineering & Architecture, Wuhan University of Technology, Wuhan, China
Watch the Video
We proposed a novel deep learning network architecture (MEBC-RCAN) for water-fat separation based on multi-echo GRE sequence. The network architecture contains three main components: the first part is Multi-Echo Bidirectional Convolutional (MEBC) to explore the correlations of successive images in multi-echo GRE; the second part is Residual Channel Attention (RCA) network to mimic the iterative optimization in traditional water-fat separation method; and the third part is Multi-Layer Feature Fusion (MLFF) to combine separation information learned from every RCA network. The results show that the proposed network could effectively obtain the high-quality water and fat images from clinical multi-echo GRE data.
|
|
0681.
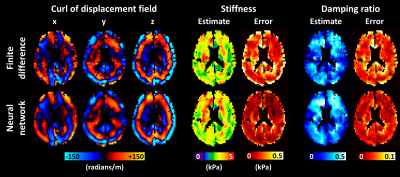 |
Artificial neural networks for numerical differentiation with application to magnetic resonance elastography
Matthew C Murphy1, Joshua D Trzasko1, Jonathan M Scott1, Armando Manduca1, John Huston, III1, and Richard L Ehman1
1Mayo Clinic, Rochester, MN, United States
Watch the Video
An artificial neural network (ANN) was trained to estimate the partial derivatives of a spatially varying field, and compared against a finite difference approach. For the application of elastography, training data were generated using a wave equation. After the training examples were corrupted by noise and missing data, the network was trained to estimate the analytical solution to the partial derivatives. In simulation, the ANN improved accuracy in noisy data but blurred sharp boundaries relative to a finite difference method. In vivo, using the ANN to compute the curl of the displacement field improved confidence in subsequent property estimates.
|
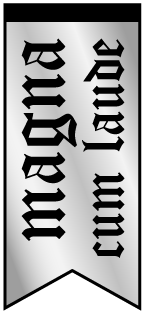 |
0682.
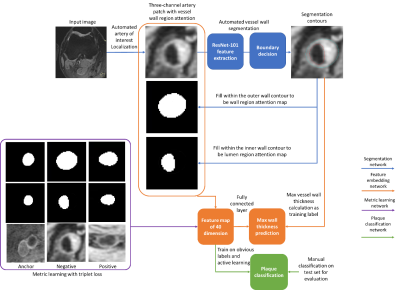 |
Visualizing and utilizing latent features of MR vessel wall images using weakly supervised deep learning analysis workflow
Li Chen1, Wenjin Liu 1, Gador Canton 1, Niranjan Balu 1, Thomas Hatsukami 1, John C. Waterton 2, Jenq-Neng Hwang 1, and Chun Yuan 1
1University of Washington, Seattle, WA, United States, 2Centre for Imaging Sciences, Manchester Academic Health Science Centre, The University of Manchester, Manchester, United Kingdom
Watch the Video
Atherosclerotic plaque information can be extracted from MR vessel wall images through transforming the images into a high dimensional feature space. However, a huge amount of human supervision has traditionally been required to achieve a meaningful feature space representation. We demonstrated that by using a weakly supervised deep learning workflow including transfer learning, active learning, and metric learning, a meaningful feature space for vessel wall analysis can be generated, which can help us to visualize the high dimensional representations of normal and diseased vessel walls images, and lead to a plaque classification area under the curve of 0.93.
|
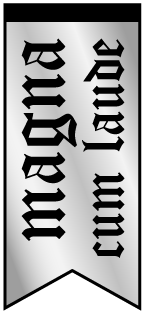 |
0683.
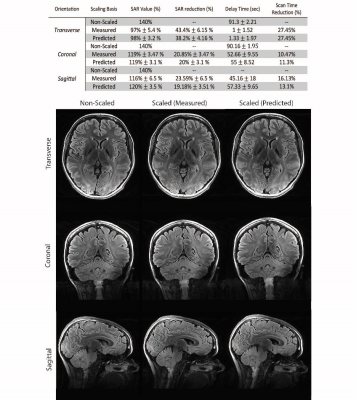 |
Investigating the robustness of convolutional neural network based B1+ prediction from localizer scans for SAR efficient 7T FLAIR imaging
Shahrokh Abbasi-Rad1, Kieran O'Brien1,2,3, Samuel Kelly1, Viktor Vegh1,3, Anders Rodell2, Yasvir Tesiram1, Jin Jin2,3,4,5, Markus Barth1,3,4, and Steffen Bollmann1,3
1Centre for Advanced Imaging, University of Queensland, Brisbane, Australia, 2Siemens Healthcare Pty Ltd, Brisbane, Australia, 3ARC Centre for Innovation in Biomedical Imaging Technology, University of Queensland, Brisbane, Australia, 4School of Information Technology and Electrical Engineering, University of Queensland, Brisbane, Australia, 5Mark and Mary Stevens Neuroimaging and Informatics Institute, University of Southern California, Los Angeles, CA, United States
Watch the Video
In 7T MRI adiabatic pulses enable robust inversion of spins at the cost of increased SAR and longer scan times. A convolutional neural network was used to estimate the B1+ profile from a localizer scan, Bloch equation simulations were used to calculate the required B1+ for adiabaticity, and adiabatic pulse power was scaled accordingly reducing SAR by up to 38%. We investigated the robustness and efficiency of this approach and showed a substantial SAR reduction is possible without an additional B1 map acquisition. This resulted in an up to 27% faster T2-FLAIR acquisition with full brain coverage.
|
|
0684.
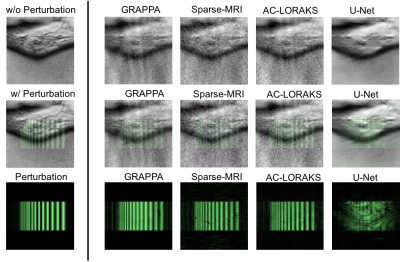 |
Local Perturbation Responses: A tool for understanding the characteristics of advanced nonlinear MR reconstruction algorithms
Chin-Cheng Chan1 and Justin P. Haldar1
1Electrical and Computer Engineering, University of Southern California, Los Angeles, CA, United States
Watch the Video
As MR image reconstruction algorithms become increasingly nonlinear, data-driven, and difficult to understand intuitively, it becomes more important that tools are available to assess the confidence that users should have about image reconstruction results. In this work, we suggest that a quantity known as the “local perturbation response” (LPR) provides useful information that can be used for this purpose. The LPR is analogous to a conventional point-spread function, but is well-suited to general image reconstruction methods that may have nonlinear and/or shift-varying characteristics. We illustrate the LPR in the context of several common image reconstruction techniques.
|
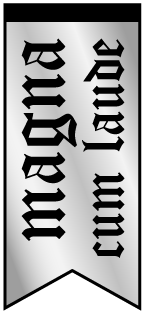 |
0685.
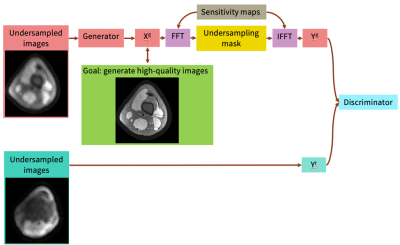 |
Unsupervised Image Reconstruction using Deep Generative Adversarial Networks
Elizabeth Cole1, Frank Ong1, John Pauly1, and Shreyas Vasanawala2
1Electrical Engineering, Stanford University, Stanford, CA, United States, 2Radiology, Stanford University, Stanford, CA, United States
Watch the Video
Many deep learning-based reconstruction methods require fully-sampled ground truth data for supervised training. However, instances exist where acquiring fully sampled data is either difficult or impossible, such as in dynamic contrast enhancement (DCE), 3D cardiac cine, 4D flow, etc. for training a reconstruction network. We present a deep learning framework for reconstructing MRI without using any fully sampled data. We test the method in two scenarios, and find the method produces higher quality images which reveal vessels and recover more anatomical structure. This method has potential in applications, such as DCE, cardiac cine, low contrast agent imaging, and real-time imaging.
|
|
0686.
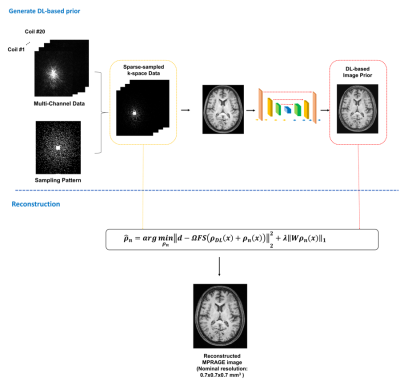 |
Highly Accelerated MPRAGE Imaging of the Brain Incorporating Deep Learning Priors with Subject-Specific Novel Features
Yue Guan1, Yudu Li2,3, Ziyu Meng3,4, Tianyao Wang5, Rong Guo2,3, Ruihao Liu4, Yao Li4, Yiping Du4, and Zhi-Pei Liang2,3
1Institute for Medical Imaging Technology, School of Biomedical Engineering, Shanghai Jiao Tong University, Shanghai, China, 2Department of Electrical and Computer Engineering, University of Illinois at Urbana-Champaign, Urbana, IL, United States, 3Beckman Institute for Advanced Science and Technology, University of Illinois at Urbana-Champaign, Urbana, IL, United States, 4Shanghai Jiao Tong University, Shanghai, China, 5Department of Radiology, The Fifth People's Hospital of Shanghai, Shanghai, China
Watch the Video
MPRAGE imaging has been widely used in clinical applications and various attempts have been made for its acceleration. This paper presents a new method to accelerate MPRAGE imaging using sparse and random sampling of k-space and constrained reconstruction incorporating image priors and subject-specific novel features. In our current implementation, the MPRAGE image priors were obtained using deep learning on data from the Human Connectome Project, and novel localized features were recovered by solving a sparsity-constrained reconstruction. In vivo experimental results demonstrated that the proposed method can produce high-quality whole-brain MPRAGE images in 0.7x0.7x0.7 mm3 nominal resolution from a 1.5-min scan.
|
|