BY SAMANTHA BY
One of the editor’s picks for the month of July is a paper entitled “Gibbs Ringing in Diffusion MRI”. Recently, we talked with Drs. Jelle Veraart, Els Fieremans, and Dmitry Novikov to learn more about how they use regularization functions to mitigate artifacts induced by Gibbs ringing in diffusion MRI.
MRMH: Jelle, how did you get into MRI?
Jelle: I was working on my Master’s thesis on computer vision for industrial applications. While doing that, I met my PhD supervisor, Jan Sijbers, who convinced me to go into MRI, where I started working on noise and biases in diffusion MRI.
MRMH: And why’d you come to New York?
Jelle: Well, I was hoping to leave the noise in the background and focus on the signal instead. [laughs]
MRMH: How about you, Els and Dmitry?
Els: I was always interested in medicine and mathematics, so I studied engineering and physics, where I got familiar with medical imaging. My interest in MRI was sparked when a sick family member required MR imaging. I got to visit an MRI facility and reading room, and was really impressed by the images of the inside of the brain. It was then that I became fascinated with MRI, so I decided to do a PhD in diffusion MRI.
Dmitry: I was always fascinated by physics and did my PhD in theoretical condensed matter physics at MIT, followed by postdocs at Princeton and Yale. It was through understanding the physics of transport of disordered systems that I got to realize that the problems in MRI are not that different from this wealth of understanding that has been accumulated in theoretical physics for over half a century, but in a different context. So I eventually switched fields, and am now a faculty member at NYU focusing on using diffusion to understand brain microstructure.
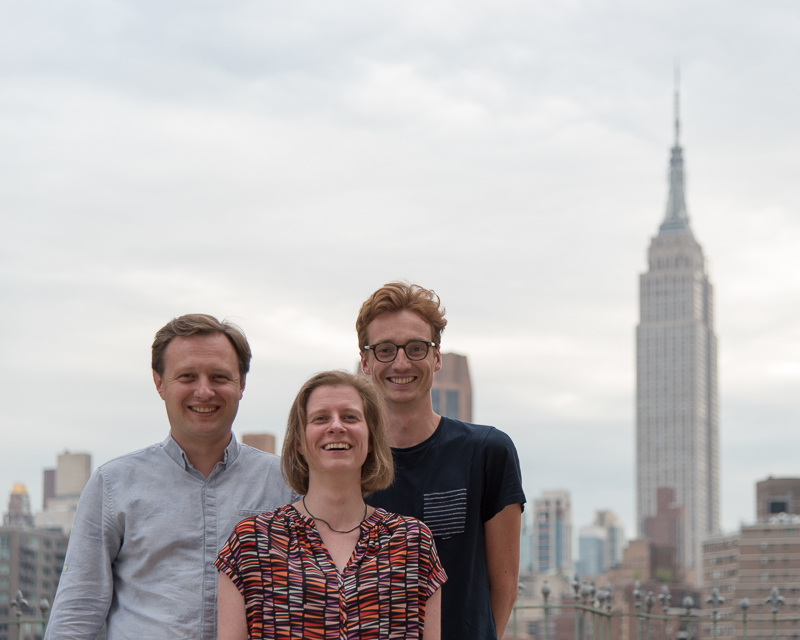
Dmitry Novikov, Els Fieremans, and Jelle Veraart in NYC.
MRMH: Let’s jump into some of the technical details of the paper. How does Gibbs ringing occur?
Jelle: Basically, a function can be written as a sum of waves with different frequencies. If you have sharp edges, like a sudden step from high to low intensities such as from CSF to the corpus callosum, you need high frequencies to describe this step. In MRI, however, because of scan time and resolution limits, we have to limit ourselves to low frequencies. Therefore, those steps cannot be well approximated, and the ringing occurs from those low frequency waves. That ringing creates over and undershoots in your signal. So, the problem is that pattern depends on the underlying signal, and in diffusion MRI, that signal depends on your b-value.
Dmitry: And the direction.
MRMH: How does this bias your diffusion MRI?
Jelle: When your non diffusion-weighted signal is being underestimated and, at the same time, the associated diffusion weighted signal is being overestimated, such as in the corpus callosum, rather than a decay in signal, you see an uptake. This can lead to negative apparent diffusivities, negative apparent kurtosis values, and basically all of the parameters that you estimate from this signal can be biased or just plain unphysical.
Dmitry: More generally, now that the field is working towards quantifying microstructure at a sub-voxel level, a lot of biophysical modeling is involved and any artifacts like Gibbs ringing, can bias any of the microstructural metrics. That was one of the main messages of our paper.
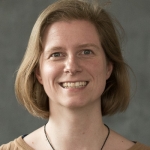
Co-author, Els Fieremans.
Els: To that end, we got really interested in getting rid of specific artifacts, because usually, the community uses smoothing to get rid of Gibbs ringing. However, we wanted to step away from that and have a more specific, targeted way of removing each specific artifact, including ringing, individually without losing anatomical detail.
MRMH: When did you decide smoothing just wasn’t going to cut it and started investigating better ways to tackle this problem?
Jelle: The timeline of Gibbs ringing in diffusion MRI is actually very interesting. In 2002, there was one ISMRM abstract by Gareth Barker and coauthors, but there was no follow-up on that problem. In 2006, Cheng Guan Koay and coauthors started doing constrained fitting, realizing it was still a problem. In 2008, Tobias Block, who is also now at NYU, came up with regularization to solve Gibbs ringing in MRI, so since we were so close to each other things started coming together with us. In the meantime, other groups have been working on this problem too. In the last year, there were three papers on Gibbs ringing in diffusion MRI – ours, Daniele Perrone and coauthors using TV regularization, and Elias Kellner and coauthors using an elegant idea of sub-voxel shifts. Three different approaches, but now we can start thinking of the best way to solve this problem.
Dmitry: Maybe the best solution isn’t even out there yet, but I think it’s really great that people are starting to pay attention to artifacts like this one. This attention to processing details is not accidental, but is rather commensurate with the exponential explosion of interest in diffusion microstructure – the number of papers in microstructure has doubled every 2.7 years for the past 15 years. Truly exponential!
MRMH: So in this paper, you tested a lot of regularization terms. What is the difference between total variation (TV) and total generalized variation (TGV)?
Jelle: In L1 regularization, you need some underlying model that sparsifies your image. With TV you assume your image can be modeled by a piecewise constant function. With TGV, it is a generalization of that, so you basically allow gradients in your image. It’s a less patchy, more natural representation of the image.
MRMH: How did these regularization functions reduce Gibbs ringing artifacts in your images?
Jelle: What we see in Gibbs ringing are oscillations, so by forcing it to a piecewise linear function, we can suppress Gibbs. We also suppress other fluctuations, such as noise, but the downside is that we might remove anatomical detail if the wrong regularization parameter is chosen. A fine balance between what’s noise, what’s Gibbs and what’s anatomy, has to be met. Compressed sensing has been using L1 regularization for a while now, but has never really answered the question of how we optimize the regularization parameter. We found that we have to tune the regularization parameter as a function of the noise level – which we recently also learned how to estimate based on random matrix theory methods.
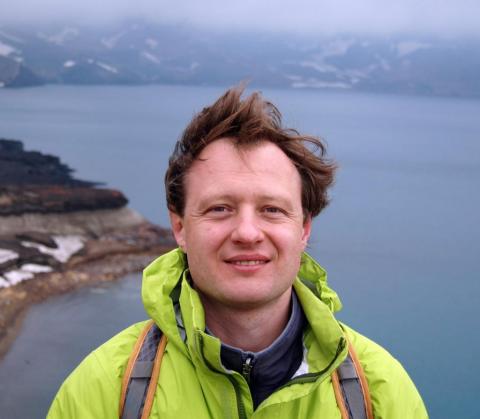
Dmitry Novikov on travels in Iceland.
Dmitry: That’s also how we avoid over-regularization.
Jelle: It’s funny…I was trying to step away from the noise, but when I started looking at the signal, I was automatically pushed to the noise again! Understanding noise seems to be essential to understand signal.
MRMH: How long does the processing take for full brain coverage? Is this something you think could be implemented on the scanner for on the fly reconstruction?
Jelle: In the current implementation, it’s CPU driven, so it would take about 45 minutes. With that said, I don’t think this technique is necessarily the endpoint. Rather than taking it to the clinic now, I think we should step back, compare all of the methods, and decide which one needs to be optimized in terms of computational time.
Dmitry: Or come up with something even better! We need to first educate ourselves, then the research community, and then the clinical community.
Els: We are collaborating with the neuroradiologists to evaluate the effect of reducing artifacts and noise. Here at NYU we have a really good connection with clinicians, so we are trying to see how our work is relevant.