One of the editor’s picks for the month of February is a paper entitled “Reducing Sensitivity Losses Due to Respiration and Motion in Accelerated Echo Planar Imaging by Reordering the Autocalibration Data Acquisition” by Drs. Jonathan R. Polimeni and Lawrence L. Wald. Currently, both are faculty members at the Athinoula A. Martinos Center for Biomedical Imaging at Harvard Medical School and Massachusetts General Hospital. We recently contacted them to talk about their method, which uses fast low- angle excitation echo-planar technique (FLEET) to reorder the auto-calibration signal (ACS) acquisition to compensate for motion and respiratory effects.
MRMH: Jon, how did you get interested in MRI?
Jon: My background is actually in neuroscience, but I got involved with MRI because I thought we were limited in our measurements that we had at the time to really answer the questions that we wanted to ask. So I wanted to learn more about MRI, and I joined Larry’s group.
Larry: Jon, when did you join us?
Jon: 2007.
Larry: Really?
MRMH: Time flies…[laughs]. How about you, Larry?
Larry: I studied condensed matter physics and was fortunate enough to be in the lab of Erwin Hahn at Berkeley doing NMR. When I graduated and got my PhD, I thought medical imaging was more interesting, and with this knowledge in MR, it was kind of a logical transition to MRI.
MRMH: So, how did your current project get started?
Jon: Once parallel imaging started kicking off, a lot of users at the Martinos Center would notice “eyeball artifacts” in their EPI data. Once we implemented a fixation task during the training data, these were removed. At 7T, we would sort of give the volunteers a pep talk and tell them not to move during the beginning of each run.
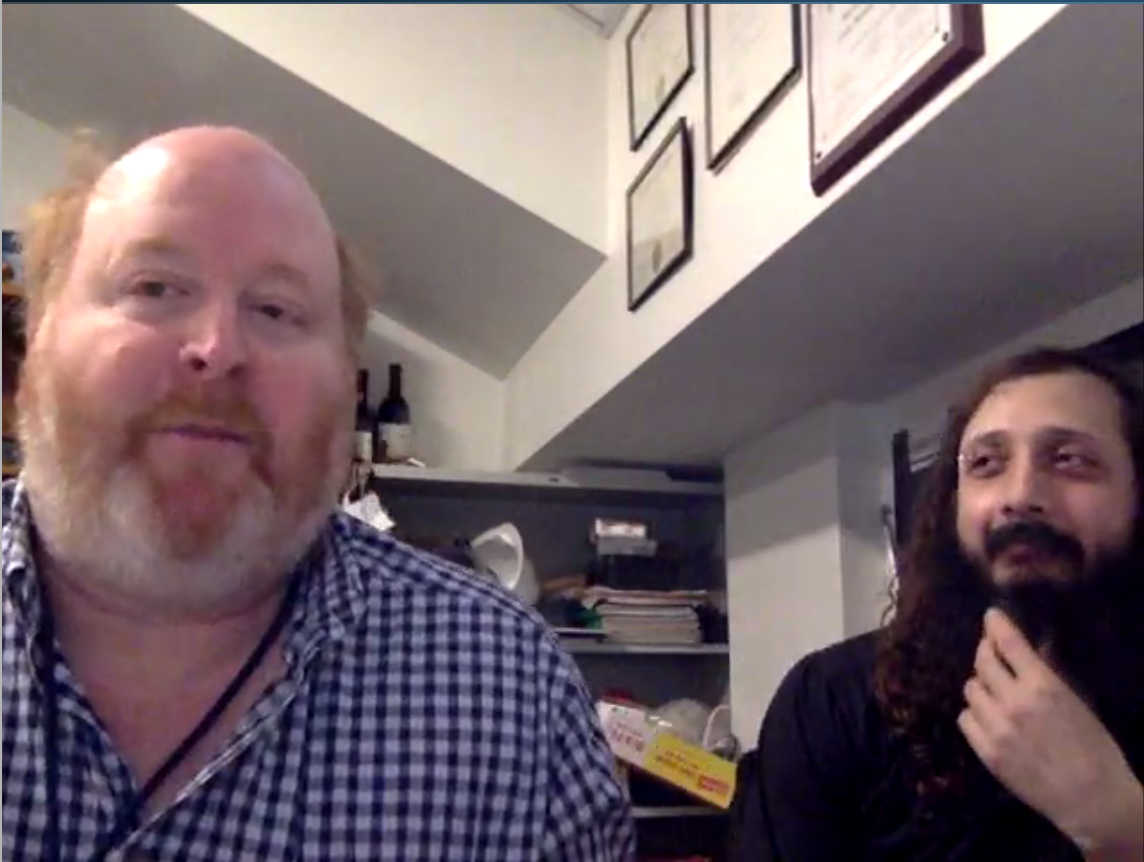
Larry and Jon captured mid-thought during the Q&A
Larry: We started noticing all of these problems that we couldn’t explain – discontinuous SNR between slices, the temporal SNR (tSNR) of GRAPPA wasn’t as good as expected. But then early on we noticed it would be a big mistake to let the subject scratch their nose during the training data. So we forced everyone to switch off the stimuli during the ACS, so that the subject was resting. Just common sense, but then all of these problems started solving themselves. When we had a subject hold his breath, all the problems vanished and we could point to respiration.
MRMH: Eureka! So, that’s how you really pinpointed that motion and respiration were an issue. What made you think about reordering the slices?
Jon: Ironically, though parallel imaging techniques were being pushed to enable single-shot EPI, on the Siemens platform, when an acceleration factor above 2 is applied, the calibration data is segmented multi-shot EPI. So every run was still subject to motion —but only at the very beginning when the ACS data were acquired. We saw a striking discontinuous tSNR across slices for GRAPPA-accelerated acquisitions with R=3 or higher. At R=2, however, the calibration data was still acquired with single-shot EPI and the discontinuous tSNR was no longer present. We had to minimize the time interval between segments.
Larry: With the conventional method, the multi-shot EPI acquisition corrupts your data. If you have a 4 second TR, you have 4 seconds in between your shots, which is plenty of time for you to be in a totally different respiratory state. Our solution was to cut that time down and reorder the slices one after the other for each segment.
MRMH: You present a lot of data in the paper – could you break it down for us in terms of what you saw in the phantom versus in vivo data?
Jon: With phantoms, image SNR and tSNR should be the same measure. As we reduced the flip angle
to acquire FLEET data, we saw that reduced SNR in the ACS data had a regularization effect: it could improve the SNR in the reconstructed images. Even in the phantom, which hopefully isn’t doing much breathing, we could improve the image SNR by virtue of the fact that the reduced flip angle in the FLEET acquisition was adding a little bit of noise, which gives the kernel a few more degrees of freedom to estimate an accurate fit.
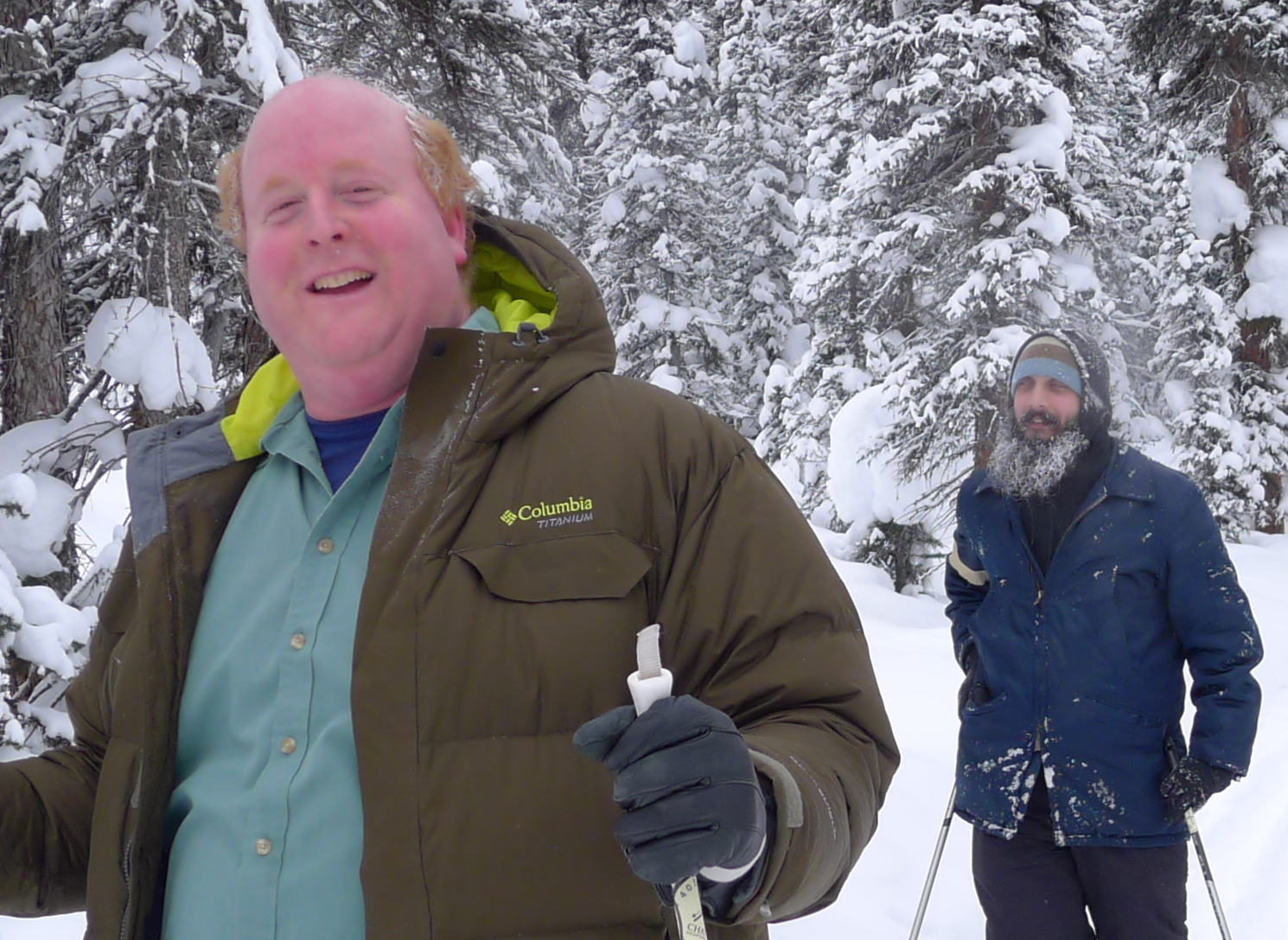
Larry and Jon traversing snowy climes
Larry: Then we added motion to the phantoms and noticed that with conventional ACS data, there were a lot of errors, but FLEET effectively froze motion by acquiring the data for one slice more quickly. With the conventional method, the first shot of slice 1 is acquired, you go through all the slices, and then take the second shot of slice 1 – now that’s a TR apart. To me that’s the beauty of the whole method. We didn’t really change much of the acquisition. We simply reordered the loop structure of the ACS between the shots and the slices. The user never notices, but suddenly you’re more robust to motion and respiration, with an average 25% increase in tSNR. There’s no downside that we can see. That’s a rare thing in MRI!
MRMH: What about the in vivo data?
Larry: This really let us show the impact of respiration on the ACS data. Our FLEET method had the same effect as a breath hold, showing that the method was effective in reducing the noise caused by respiration.
MRMH: Are there any artifacts due to cardiac cycle?
Jon: That’s a good question…but more difficult to prove! The breath hold test, however, was able to resolve most of the artifacts we were seeing in terms of SNR, so this showed respiration was the dominant factor.
Larry: Yeah, we can’t really have a volunteer turn on and off their cardiac cycle [laughs].
MRMH: What are some applications you have in mind?
Jon: The Maastricht group is actually using it on their ASL data for perfusion. Diffusion and fMRI are also good applications. Some of our colleagues have been asking about body imaging, which you can imagine is going to be more problematic than the brain.
Larry: Certainly any EPI application should automatically have it – we don’t really see any negative, so why not give it a try?
*Interview conducted by Samantha By and Nikola Stikov.