Dr. Li Feng and Dr. Ricardo Otazo are researchers at the New York University School of Medicine, whose paper on golden angle radial MRI with compressed sensing and parallel imaging is one of our Editor’s picks for the month of February. Li recently finished his doctorate at NYU, whereas Ricardo arrived in New York from the University of New Mexico, where he completed his PhD in 2007. They are both in love with what New York has to offer, academically as well as socially. We met over Skype to discuss XD-GRASP, ? free-breathing MR imaging framework that combines the acceleration capability of compressed sensing and the self-navigation properties of radial imaging to reconstruct dynamic motion-resolved multidimensional data.
MRMH: Why did you choose to work on this specific project?
Li: Since the beginning of my PhD, I have been working with Ricardo and Dr. Dan Sodickson at NYU on compressed sensing (CS) MRI. We started with Cartesian sampling, but we found it had some limitation in terms of incoherence, so we moved towards radial sampling. We found the golden angle radial sampling very interesting because it enables continuous data sampling without the need to predefine temporal frames. Later we also found that the self-navigation property of radial sampling can be further incorporated into the compressed sensing framework to reconstruct motion-resolved dynamic images.
MRMH: What is golden angle radial sampling, and why is it important?
Li: The golden angle comes from dividing 180 degrees by the golden ratio 1.618. In golden angle radial sampling, the sampled spokes carry equal amount of information and they never repeat each other. Meanwhile, they always add complementary information by filling the largest gap left by previously sampled spokes in k-space. Therefore, it is well suited for continuous k-space updates.
Ricardo: It is important because you can do continuous data acquisition, providing approximately uniform coverage of k-space. This is a perfect sampling scheme for uncorrelated samples along the temporal dimension. That is how we get temporal incoherence for compressed sensing.
MRMH: What would you say is the biggest advantage and the biggest challenge of your approach?
Li: Our free-breathing imaging framework does not require any assumptions on the motion model. This is a big advantage for moving towards our ultimate goal of rapid and continuous acquisition for easy and flexible MRI workflow.
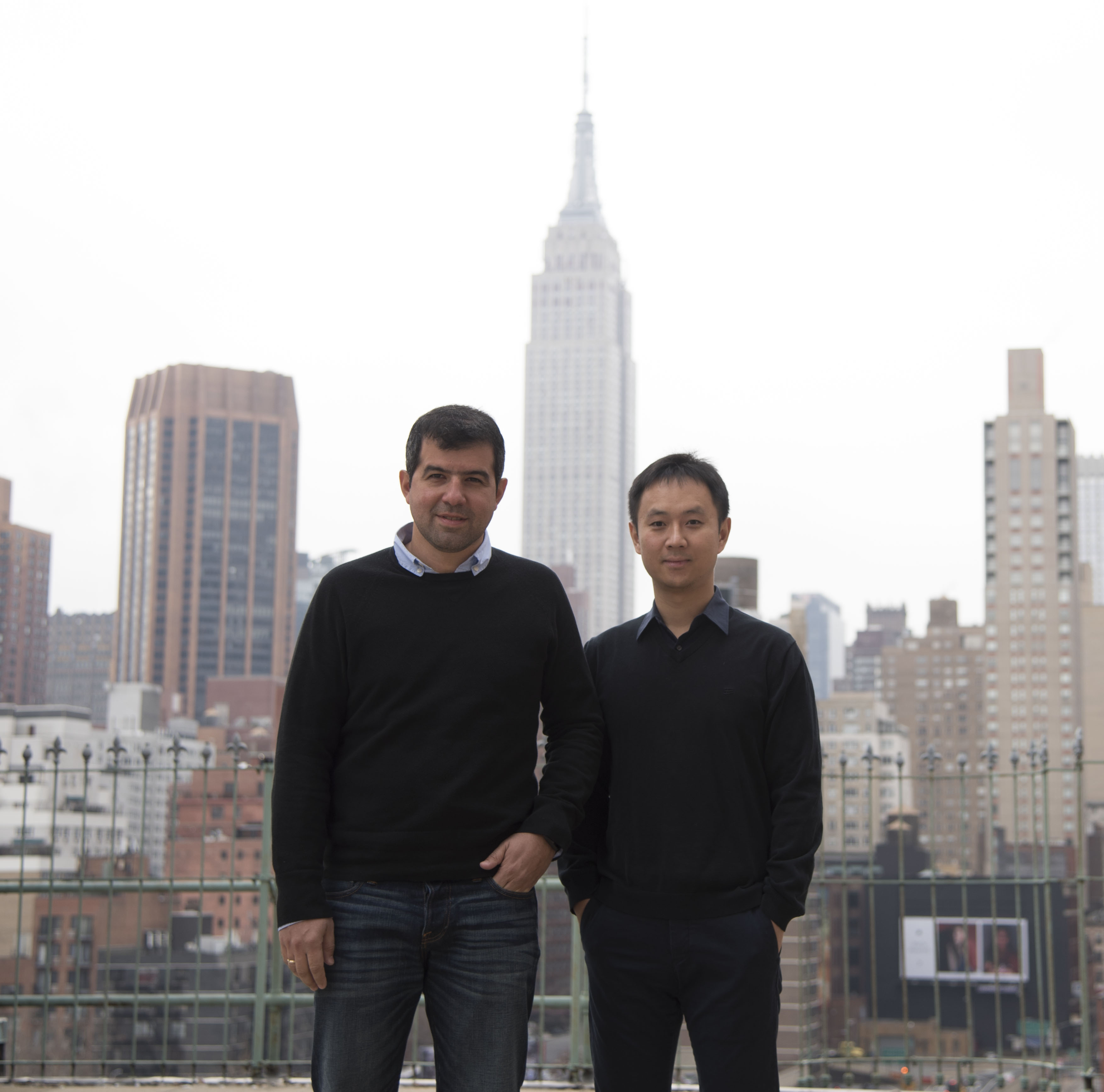
Ricardo and Li in downtown NYC
Ricardo: The biggest challenge is image quality assessment, not only for this method but for other compressed sensing reconstruction algorithms in general. Currently we show radiologists the reconstructed images, and ask them to assign a grade. It would be nice if we can have an automatic method that can tell us how well we are doing, in particular for lesion detectability.
MRMH: Is a periodic signal a requirement for compressed sensing in this framework?
Li: It is a requirement. However, respiratory and cardiac motion are major sources of artifacts in clinical imaging. As both motions are periodic, they are well suited to our framework. However, there is no requirement on the motion pattern, and the change of breathing cycle or cardiac cycle won’t matter for our method as long as there are occasional peaks and valleys.
Ricardo: If the motion is exactly periodic, that is great. In that case it is very easy to do compressed sensing, because the signal will be really sparse in the temporal Fourier transform domain. In the case of DCE imaging, it is a non-periodic process, but we can still use our approach to reconstruct an extra respiratory dimension. In the end, we are not just solving a motion correction problem. We also try to get as much information out of motion as possible, and use it to reconstruct an image.
MRMH: So how do you handle both types of motion, cardiac and respiratory?
Li: Cardiac and respiratory motion occur simultaneously at two different frequencies. After the data acquisition, we are trying to sort the data into two separate dimensions according to this frequency difference. Given they are both periodic, we can just scan for 10 to 20 seconds and then sort the acquisitions to get enough data for each dimension.
Ricardo: The sampling frequency depends on the clinical need. For dynamic contrast enhanced imaging of liver, we can just reconstruct several contrast enhancement phases that are needed for clinical diagnosis. In the case where we need finer sampling of the respiratory cycle, such as evaluation of the lung function, we can reconstruct the images with a higher temporal resolution. But that does not mean we need to image faster. We can take advantage of the fact that respiratory motion is periodic, and just image for a longer period of time and synchronize the acquired data.
MRMH: Is the computation time acceptable?
Li: That depends on the patience of the radiologists. Radiologists at NYU are fine with half an hour or even one hour long reconstruction time. The current reconstruction takes 30 to 50 minutes for the 3D DCE-liver studies.
Ricardo: We are regularly using this technique at our site for oncological imaging studies, but more powerful computers can reduce the computation time significantly. With cloud computing, like the Amazon web service, we tested our algorithm on 10,000 cores with 255 GB of memory, and got the reconstruction down to 5 seconds.
MRMH: Any parting thoughts for our readers?
Li: We would like to share our technique. That is why we put all our source code and example datasets online (http://cai2r.net/resources/software) so people can download it and try it out.
*Interview conducted by Hong Shang and Nikola Stikov.